Zeroth-Order primal-dual Alternating Projection Gradient Algorithms for Nonconvex Minimax Problems with Coupled linear Constraints
CoRR(2024)
摘要
In this paper, we study zeroth-order algorithms for nonconvex minimax
problems with coupled linear constraints under the deterministic and stochastic
settings, which have attracted wide attention in machine learning, signal
processing and many other fields in recent years, e.g., adversarial attacks in
resource allocation problems and network flow problems etc. We propose two
single-loop algorithms, namely the zero-order primal-dual alternating projected
gradient (ZO-PDAPG) algorithm and the zero-order regularized momentum
primal-dual projected gradient algorithm (ZO-RMPDPG), for solving deterministic
and stochastic nonconvex-(strongly) concave minimax problems with coupled
linear constraints. The iteration complexity of the two proposed algorithms to
obtain an ε-stationary point are proved to be
𝒪(ε ^-2) (resp. 𝒪(ε ^-4)) for
solving nonconvex-strongly concave (resp. nonconvex-concave) minimax problems
with coupled linear constraints under deterministic settings and
𝒪̃(ε ^-3) (resp.
𝒪̃(ε ^-6.5)) under stochastic settings
respectively. To the best of our knowledge, they are the first two zeroth-order
algorithms with iterative complexity guarantees for solving
nonconvex-(strongly) concave minimax problems with coupled linear constraints
under the deterministic and stochastic settings.
更多查看译文
AI 理解论文
溯源树
样例
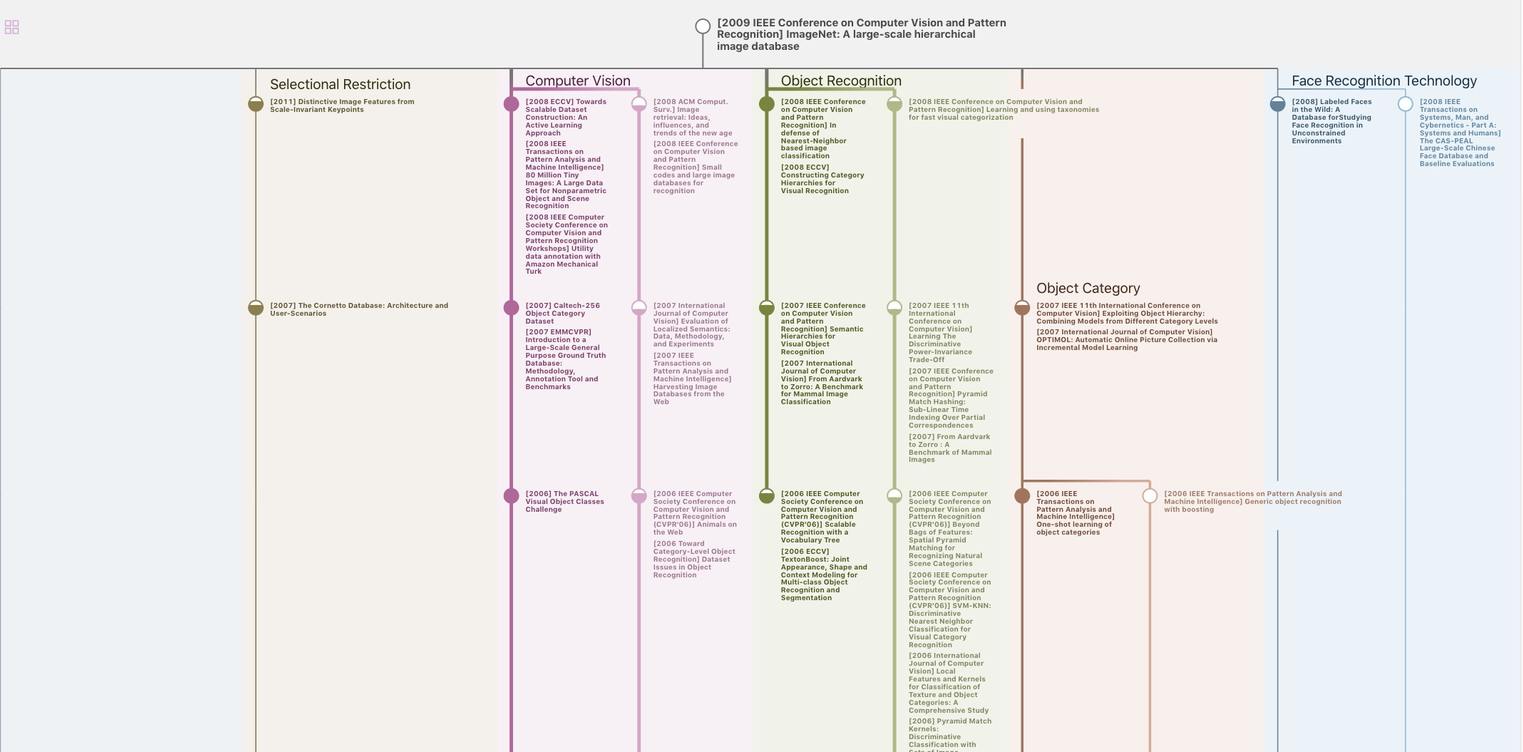
生成溯源树,研究论文发展脉络
Chat Paper
正在生成论文摘要