Technical note: Generalizable and promptable artificial intelligence model to augment clinical delineation in radiation oncology
MEDICAL PHYSICS(2024)
摘要
Background: Efficient and accurate delineation of organs at risk (OARs) is a critical procedure for treatment planning and dose evaluation. Deep learning-based auto-segmentation of OARs has shown promising results and is increasingly being used in radiation therapy. However, existing deep learning-based auto-segmentation approaches face two challenges in clinical practice: generalizability and human-AI interaction. A generalizable and promptable auto-segmentation model, which segments OARs of multiple disease sites simultaneously and supports on-the-fly human-AI interaction, can significantly enhance the efficiency of radiation therapy treatment planning. Purpose: Meta's segment anything model (SAM) was proposed as a generalizable and promptable model for next-generation natural image segmentation. We further evaluated the performance of SAM in radiotherapy segmentation. Methods: Computed tomography (CT) images of clinical cases from four disease sites at our institute were collected: prostate, lung, gastrointestinal, and head & neck. For each case, we selected the OARs important in radiotherapy treatment planning. We then compared both the Dice coefficients and Jaccard indices derived from three distinct methods: manual delineation (ground truth), automatic segmentation using SAM's 'segment anything' mode, and automatic segmentation using SAM's 'box prompt' mode that implements manual interaction via live prompts during segmentation. Results: Our results indicate that SAM's segment anything mode can achieve clinically acceptable segmentation results in most OARs with Dice scores higher than 0.7. SAM's box prompt mode further improves Dice scores by 0.1 similar to 0.5. Similar results were observed for Jaccard indices. The results show that SAM performs better for prostate and lung, but worse for gastrointestinal and head & neck. When considering the size of organs and the distinctiveness of their boundaries, SAM shows better performance for large organs with distinct boundaries, such as lung and liver, and worse for smaller organs with less distinct boundaries, like parotid and cochlea. Conclusions: Our results demonstrate SAM's robust generalizability with consistent accuracy in automatic segmentation for radiotherapy. Furthermore, the advanced box-prompt method enables the users to augment auto-segmentation interactively and dynamically, leading to patient-specific auto-segmentation in radiation therapy. SAM's generalizability across different disease sites and different modalities makes it feasible to develop a generic auto-segmentation model in radiotherapy.
更多查看译文
关键词
artificial intelligence,clinical delineation,generalizable,promptable,radiation oncology,segment anything model
AI 理解论文
溯源树
样例
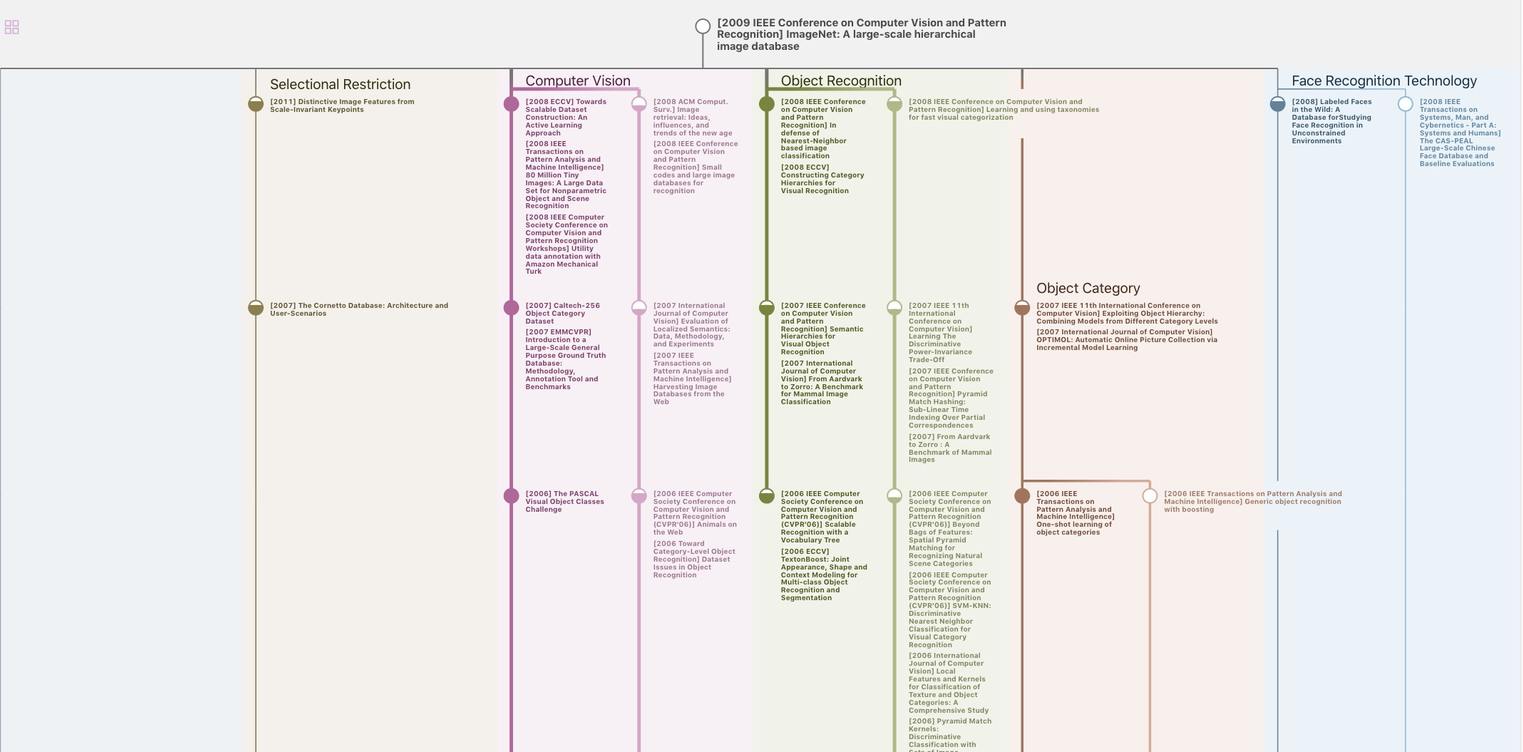
生成溯源树,研究论文发展脉络
Chat Paper
正在生成论文摘要