A Deep Patient-Similarity Learning Framework for the Assessment of Diastolic Dysfunction in Elderly Patients.
European heart journal. Cardiovascular Imaging(2024)
摘要
BACKGROUND AND AIMS:Age-related changes in cardiac structure and function are well recognized and make the clinical determination of abnormal LV diastolic function (LVDD) particularly challenging in the elderly. We investigated whether a deep neural network (DeepNN) model[70] of LVDD, previously validated in a younger cohort, can be implemented in an older population to predict incident heart failure (HF).
METHODS:A previously developed DeepNN was tested on 5,596 older participants (66-90 years; 57% female; 20% black) from the Atherosclerosis Risk in Communities study. The association of DeepNN predictions with HF or all-cause death for the American College of Cardiology Foundation/American Heart Association Stage A/B (n = 4,054) and Stage C/D (n = 1,542) subgroups was assessed.
RESULTS:The DeepNN-predicted High-Risk compared to the Low-Risk phenogroup demonstrated an increased incidence of HF and death for both Stage A/B and Stage C/D (log-rank p < 0.0001 for all). In multivariable analyses, the High-Risk phenogroup remained an independent predictor of HF and death in both Stages A/B (adjusted hazard ratio (HR) [95% confidence interval], 6.52[4.20-10.13] and 2.21(1.68-2.91), both p < 0.0001) and Stage C/D (6.51[4.06-10.44] and 1.03(1.00-1.06), both p < 0.0001) respectively. In addition, DeepNN showed incremental value over the 2016 ASE/EACVI guidelines (Net reclassification index, 0.5[CI:0.4-0.6], p < 0.001; C-statistic improvement, DeepNN [0.76] vs. ASE/EACVI [0.70], p < 0.001) overall and maintained across stage-groups.
CONCLUSIONS:Despite training with a younger cohort, a deep patient-similarity-based learning framework for assessing LVDD provides a robust prediction of all-cause death and incident HF for older patients.
更多查看译文
AI 理解论文
溯源树
样例
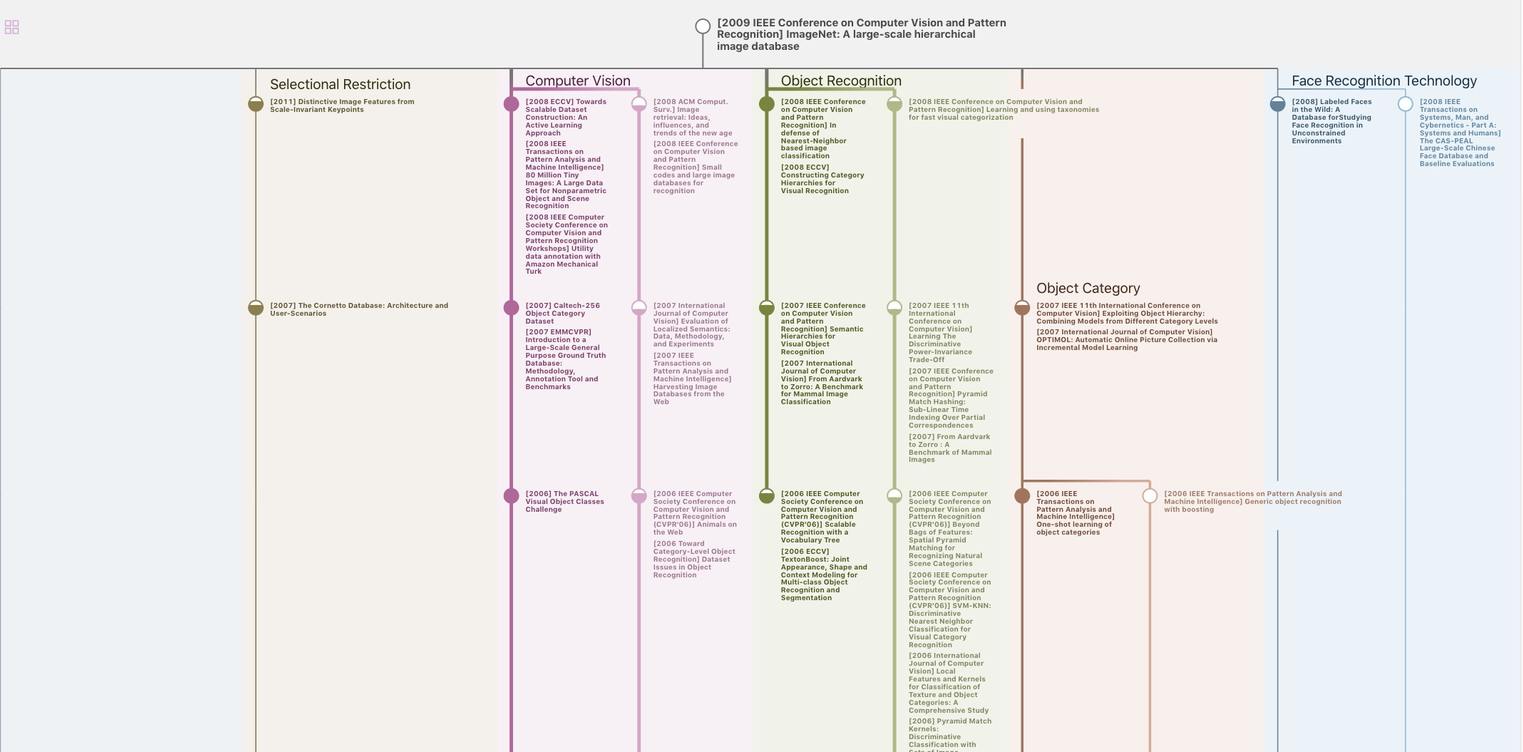
生成溯源树,研究论文发展脉络
Chat Paper
正在生成论文摘要