Multi-Task Learning of Object States and State-Modifying Actions from Web Videos.
IEEE transactions on pattern analysis and machine intelligence(2024)
摘要
We aim to learn to temporally localize object state changes and the corresponding state-modifying actions by observing people interacting with objects in long uncurated web videos. We introduce three principal contributions. First, we develop a self-supervised model for jointly learning state-modifying actions together with the corresponding object states from an uncurated set of videos from the Internet. The model is self-supervised by the causal ordering signal, i.e., initial object state manipulating action end state. Second, we explore alternative multi-task network architectures and identify a model that enables efficient joint learning of multiple object states and actions, such as pouring water and pouring coffee, together. Third, we collect a new dataset, named ChangeIt, with more than 2600 hours of video and 34 thousand changes of object states. We report results on an existing instructional video dataset COIN as well as our new large-scale ChangeIt dataset containing tens of thousands of long uncurated web videos depicting various interactions such as hole drilling, cream whisking, or paper plane folding. We show that our multi-task model achieves a relative improvement of 40% over the prior methods and significantly outperforms both image-based and video-based zero-shot models for this problem.
更多查看译文
关键词
Weakly-supervised learning,temporal action localization,object states
AI 理解论文
溯源树
样例
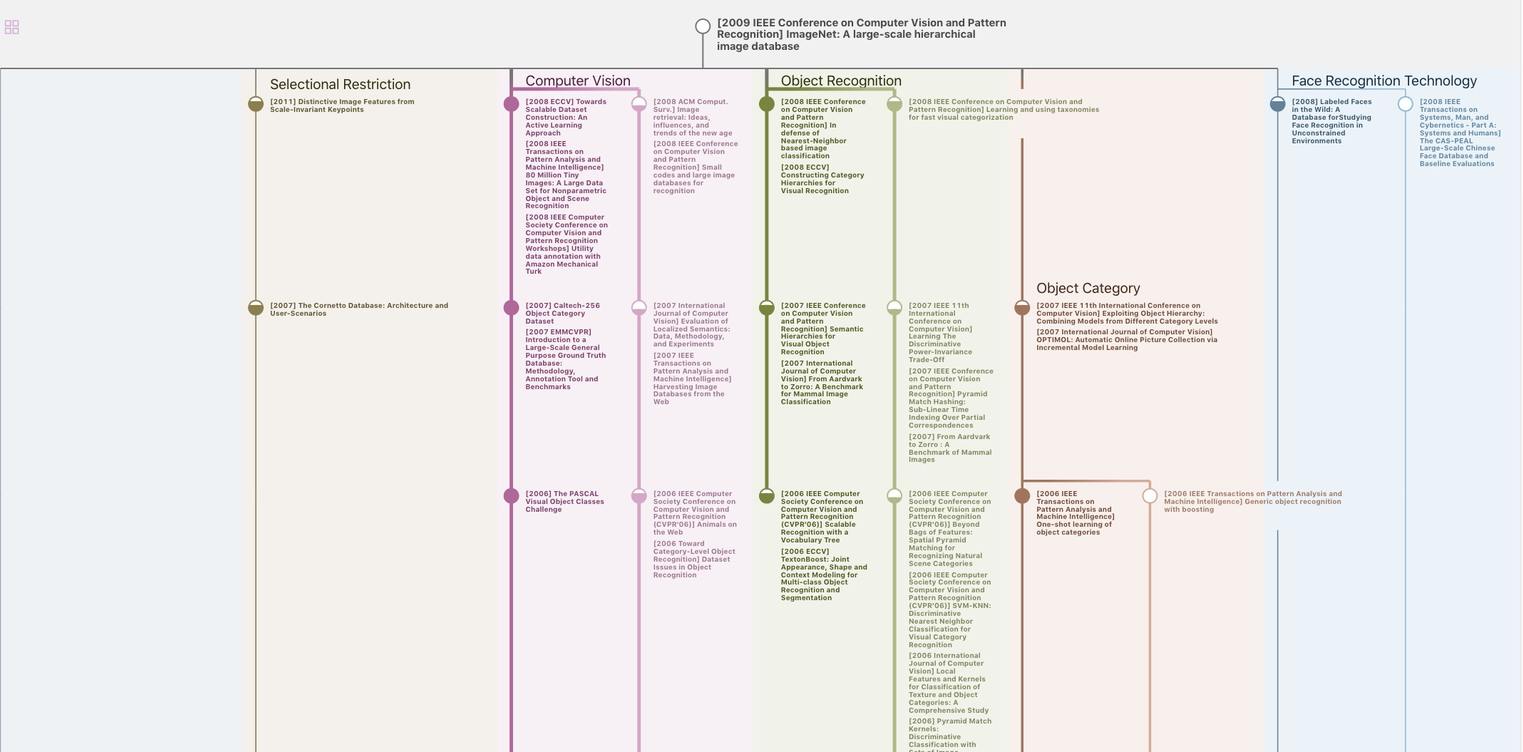
生成溯源树,研究论文发展脉络
Chat Paper
正在生成论文摘要