Feedback Decision Transformer: Offline Reinforcement Learning With Feedback
23RD IEEE INTERNATIONAL CONFERENCE ON DATA MINING, ICDM 2023(2023)
摘要
Recent trajectory optimization methods for dline reinforcement learning (RL) define the problem as one of conditional-sequence policy modeling. One of these methods is Decision Transformer (DT), a Transformer-based trajectory optimization approach that achieved competitive results with the current state-of-the-art. Despite its high capabilities, DT underperforms when the training data does not contain full trajectories, or when the recorded behavior does not offer sufficient coverage of the states-actions space. We propose Feedback Decision Transformer (FDT), a data -driven approach that uses limited amounts of high-quality feedback at critical states to significantly improve DT's performance. Our approach analyzes and estimates the Q-function across the states -actions space, and identifies areas where feedback is likely to be most impactful. Next, we integrate this feedback into our model, and use it to improve our model's performance. Extensive evaluation and analysis on four Atari games show that FDT significantly outperforms DT in multiple setups and configurations.
更多查看译文
关键词
Deep Reinforcement Learning,Offline Reinforcement Learning,RL with lltiman Feedback
AI 理解论文
溯源树
样例
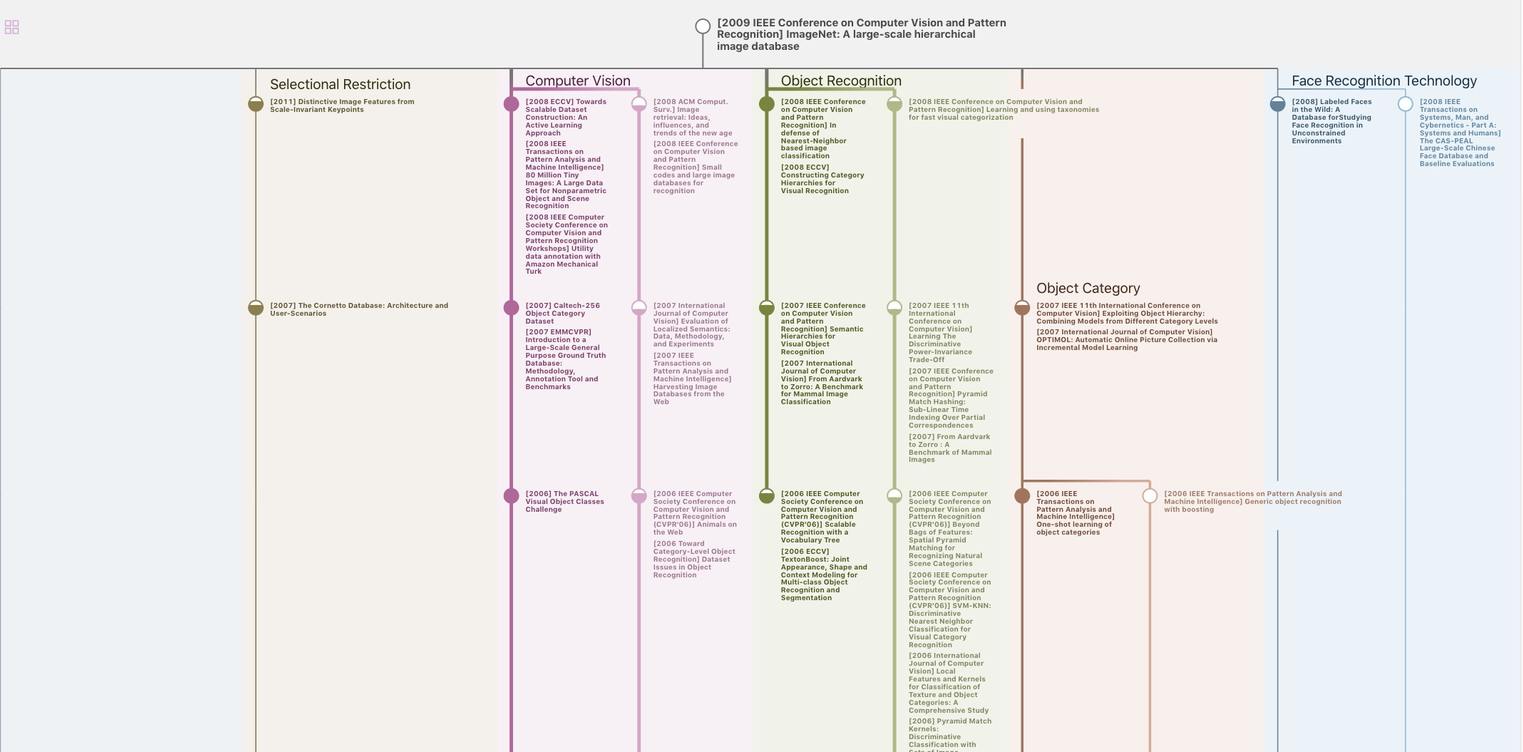
生成溯源树,研究论文发展脉络
Chat Paper
正在生成论文摘要