Machine learning-derived model for predicting poor post-treatment quality of life in Korean cancer survivors
Supportive Care in Cancer(2024)
摘要
Purpose A substantial number of cancer survivors have poor quality of life (QOL) even after completing cancer treatment. Thus, in this study, we used machine learning (ML) to develop predictive models for poor QOL in post-treatment cancer survivors in South Korea. Methods This cross-sectional study used online survey data from 1,005 post-treatment cancer survivors in South Korea. The outcome variable was QOL, which was measured using the global QOL subscale of the European Organization of Cancer and Treatment for Cancer Quality of Life Questionnaire, where a global QOL score < 60.4 was defined as poor QOL. Three ML models (random forest (RF), support vector machine, and extreme gradient boosting) and three deep learning models were used to develop predictive models for poor QOL. Model performance regarding accuracy, area under the receiver operating characteristic curve, F1 score, precision, and recall was evaluated. The SHapely Additive exPlanation (SHAP) method was used to identify important features. Results Of the 1,005 participants, 65.1% had poor QOL. Among the six models, the RF model had the best performance (accuracy = 0.85, F1 = 0.90). The SHAP method revealed that survivorship concerns (e.g., distress, pain, and fatigue) were the most important factors that affected poor QOL. Conclusions The ML-based prediction model developed to predict poor QOL in Korean post-treatment cancer survivors showed good accuracy. The ML model proposed in this study can be used to support clinical decision-making in identifying survivors at risk of poor QOL.
更多查看译文
关键词
Cancer survivors,Machine learning,Prediction model,Quality of life
AI 理解论文
溯源树
样例
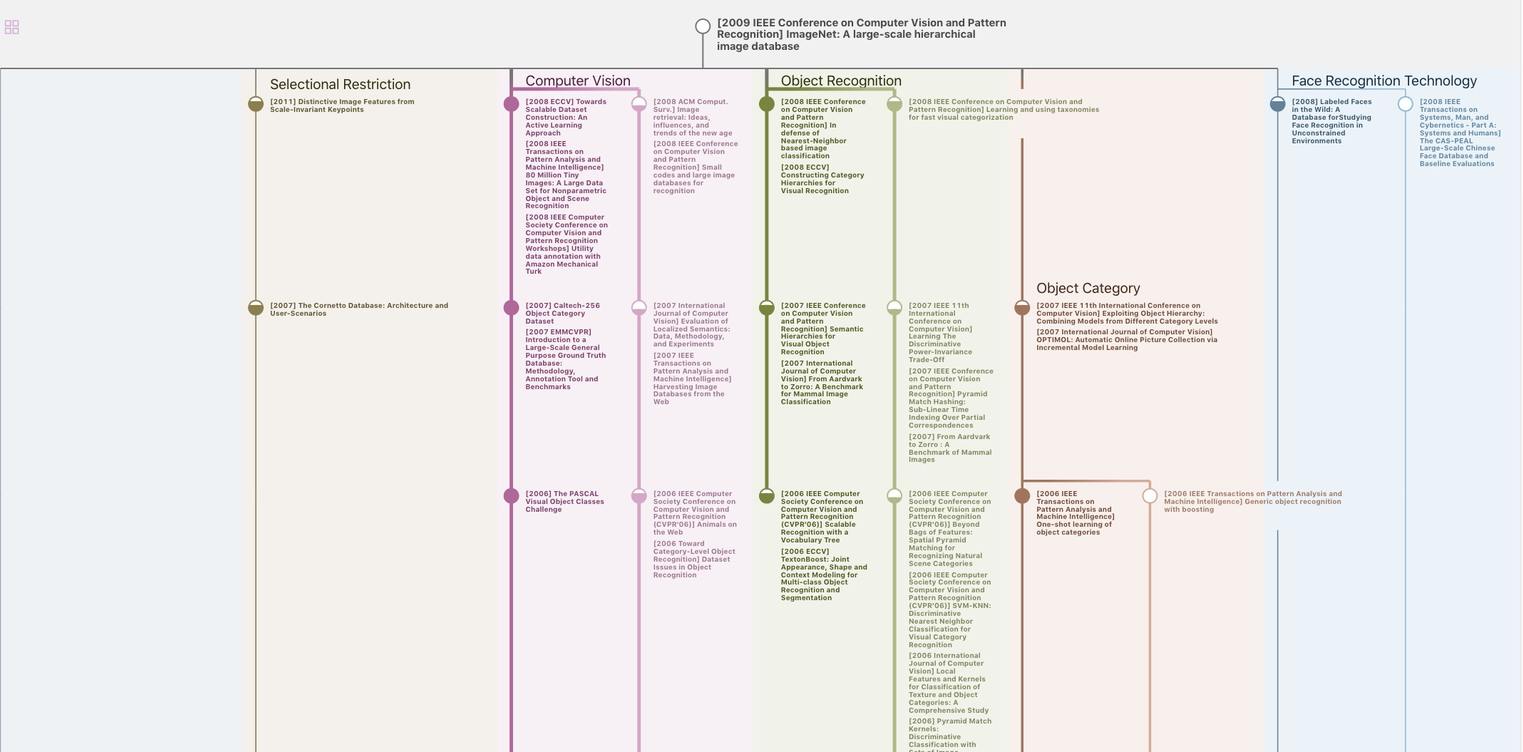
生成溯源树,研究论文发展脉络
Chat Paper
正在生成论文摘要