Distributional Cloning for Stabilized Imitation Learning via ADMM
23RD IEEE INTERNATIONAL CONFERENCE ON DATA MINING, ICDM 2023(2023)
摘要
The two leading solution paradigms for imitation learning (IL), BC and GAIL, each suffers from notable drawbacks. BC, a supervised learning approach to mimic expert actions, is vulnerable to covariate shift. GAIL applies adversarial training to minimize the discrepancy between expert and learner behaviors, which is prone to unstable training and mode collapse. In this work, we propose DC Distributional Cloning a novel IL approach for addressing the covariate shift and mode collapse problems simultaneously. DC directly maximizes the likelihood of observed expert and learner demonstrations, and gradually encourages the learner to evolve towards expert behaviors based on an averaging effect. The DC solution framework contains two stages in each training loop, where in stage one the mixed expert and learner state distribution is estimated via SoftFlow, and in stage two the learner policy is trained to match both the expert's policy and state distribution via ADMM. Experimental evaluation of DC compared with several baselines in 10 different physics-based control tasks reveal superior results in learner policy performance, training stability, and mode distribution preservation.
更多查看译文
关键词
Terms imitation learning,neural ordinary differential equations
AI 理解论文
溯源树
样例
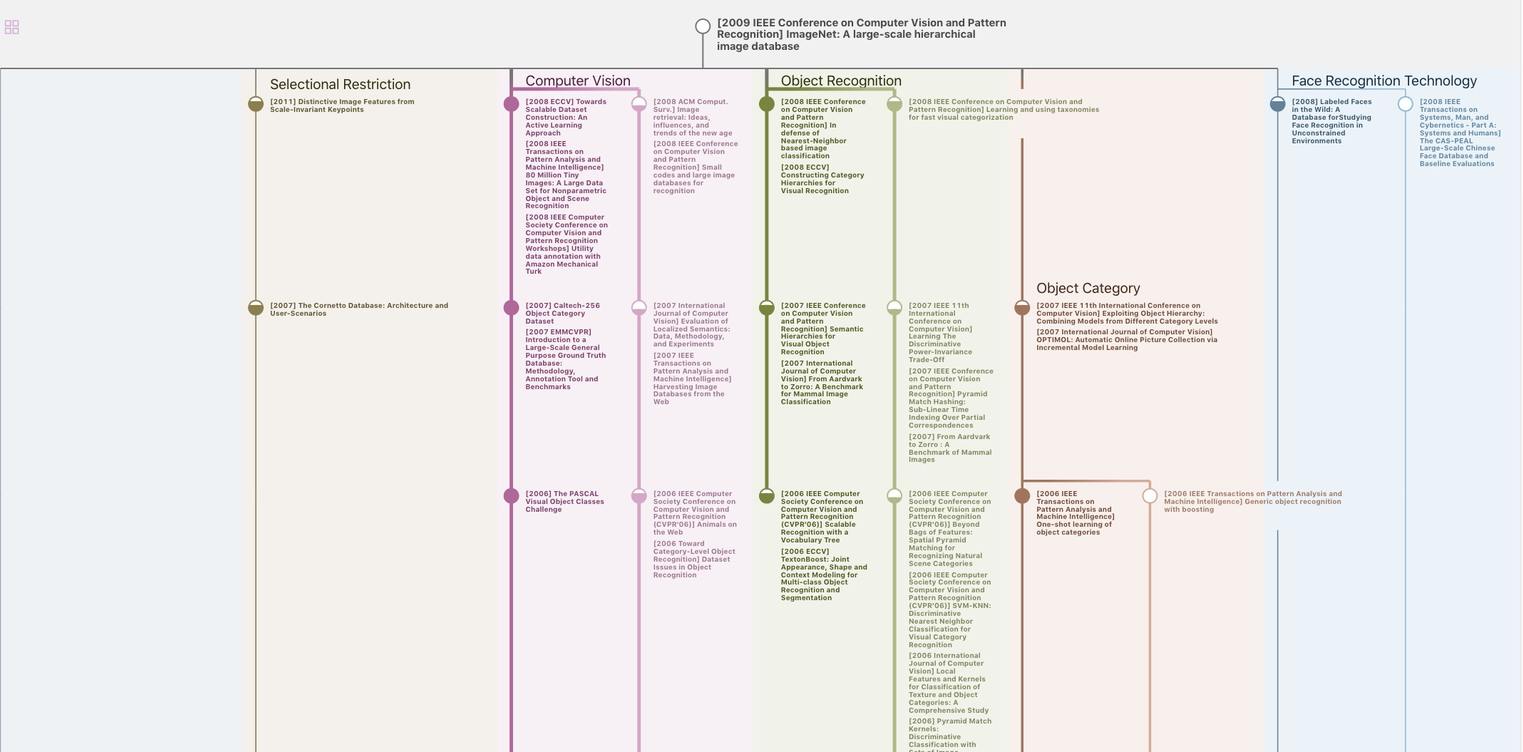
生成溯源树,研究论文发展脉络
Chat Paper
正在生成论文摘要