Infinitely Deep Graph Transformation Networks
23RD IEEE INTERNATIONAL CONFERENCE ON DATA MINING, ICDM 2023(2023)
摘要
This work develops a node-edge co-evolution model for attributed graph transformation, where both the node and edge attributes undergo changes due to complex interactions. Due to two fundamental obstacles, learning and approximating attributed graph transformation have not been thoroughly explored: 1) the difficulty of jointly considering four types of atomic interactions including nodes -to-edges, nodes-to-nodes, edges -to-nodes, and edges-to-edges interactions. 2) the difficidly of capturing iterative long-range interactions between nodes and edges. To solve these issues, we offer a novel and scalable equilibrium model, NEC infinity, with node-edge message passing and edge-node message passing. Additionally, we propose an efficient optimization algorithm that is based on implicit gradient theorem and includes a theoretical analysis of NEC infinity. The effectiveness and efficiency of the proposed model have been demonstrated through extensive experiments on synthetic and real -world data sets.
更多查看译文
关键词
Terms GNN,IGNN,Implicit model,Graph translation,Graph transformation,Attributed graph
AI 理解论文
溯源树
样例
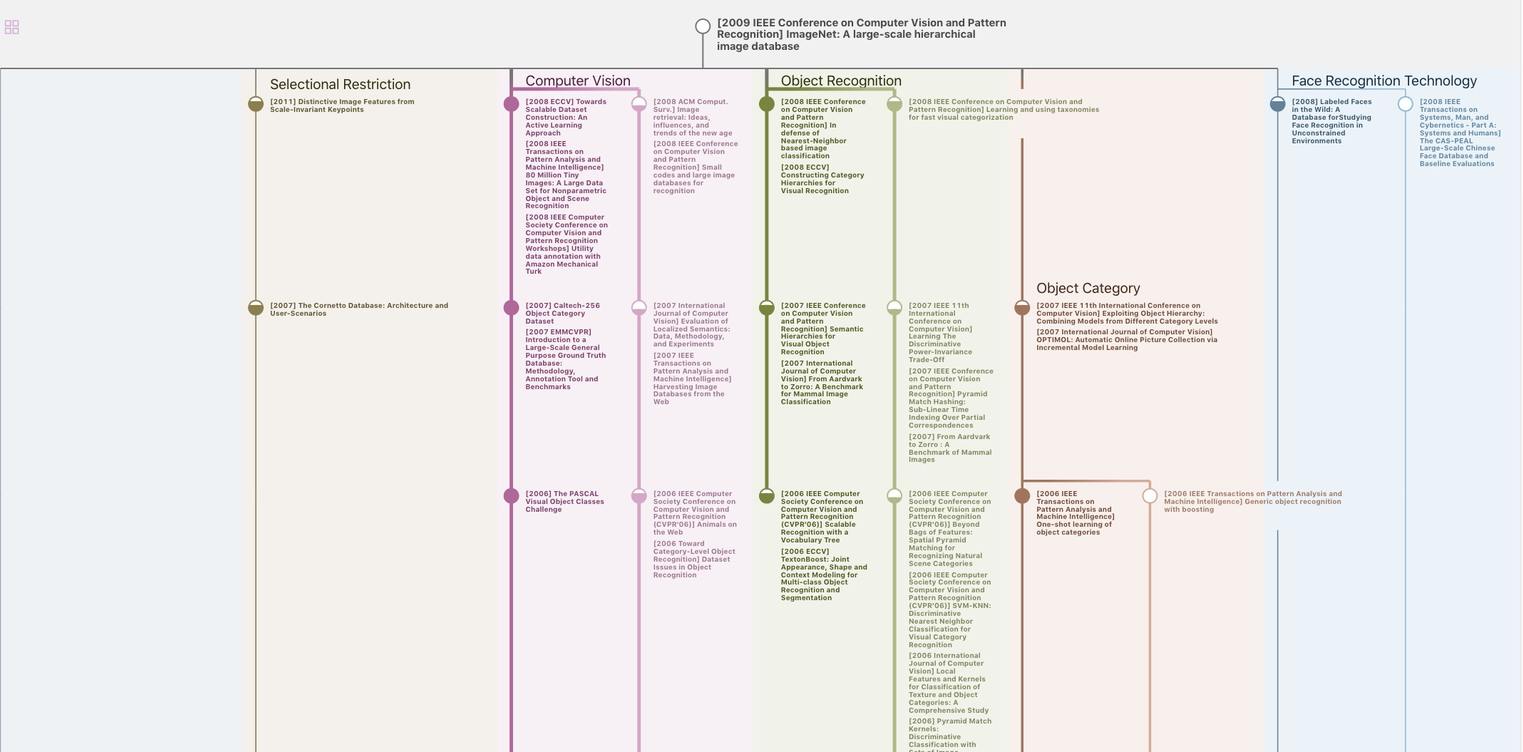
生成溯源树,研究论文发展脉络
Chat Paper
正在生成论文摘要