Adaptive Student Inference Network for Efficient Single Image Super-Resolution
23RD IEEE INTERNATIONAL CONFERENCE ON DATA MINING, ICDM 2023(2023)
摘要
Recent advances in single image super -resolution (SISR) have achieved remarkable performance through deep learning. However, the high computational cost hinders the deployment of SISR models on edge devices. Instead of proposing new SISR models, a new trend is emerging to improve network efficiency by reducing parameters, FLOPs, and inference time through slight modifications to the original models. However, recent methods usually focus on reducing only one of three metrics, i.e., FLOPs, parameters and inference time, which inevitably increases the other two metrics. In this paper, we propose a novel Adaptive Student Inference Network (ASIN) on popular SISK models, which aims at reducing FLOPS and inference time while maintaining the number of parameters and restoring clearer high-resolution images. Specifically, our ASIN divides a SISR model into three components (head, body and tail) and adopts various strategies for each part. For head and tail parts, to ensure the restored images contain more detailed information, a novel auxiliary Enhanced Teacher Network (ET-Net) is designed, which is trained with the ground -truth images to obtain more prior knowledge to guide student network to extract more accurate textures using a new knowledge distillation method. For the body part, owing to the varying difficulties of the reconstructions in different regions, we propose an Adaptive Depth Predicted Module (ADPM) to dynamically shorten average depth of network to reduce the computational cost of overall network. Extensive experiments on two datasets demonstrate the effectiveness and state-of-the-art performance of our ASIN compared to its counterparts.
更多查看译文
关键词
Efficient single image super-resolution,knowledge distillation,region-aware
AI 理解论文
溯源树
样例
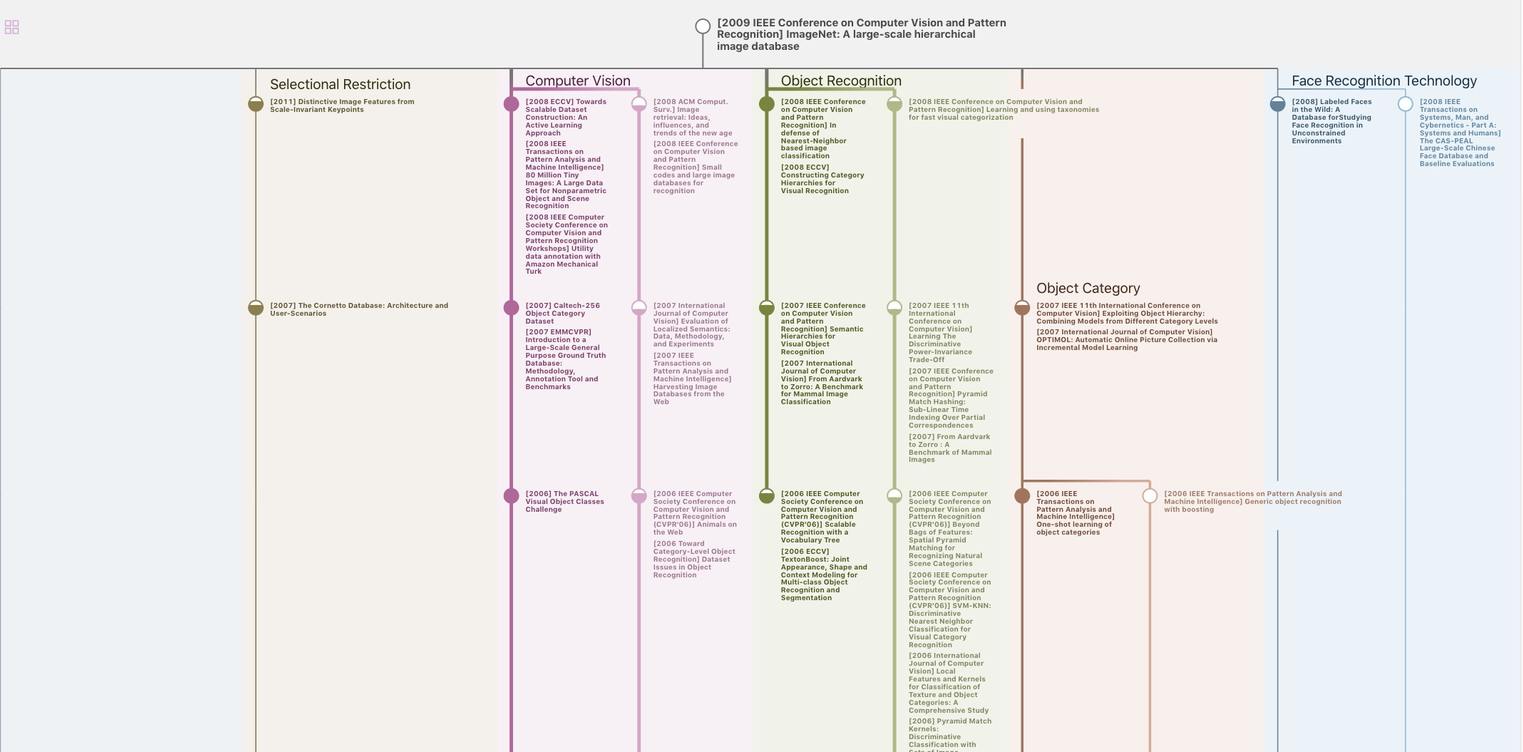
生成溯源树,研究论文发展脉络
Chat Paper
正在生成论文摘要