Using UAV-Based Multispectral Imagery, Data -Driven Models, and Spatial Cross -Validation for Corn Grain Yield Prediction
23RD IEEE INTERNATIONAL CONFERENCE ON DATA MINING, ICDM 2023(2023)
摘要
Input cost reductions and yield optimization can be done using yield precision maps created by machine learning models to address the increase in food demand predicted by 2050. However, without taking into account the spatial structure of the data, the precision map's accuracy evaluation assessment runs the risk of being overly optimistic. In the current work, a corn yield prediction study was conducted, and the predictive abilities of two vegetation indices (VIs) and five spectral bands for a single image acquisition date were evaluated. We also examined the impacts of image spatial and spectral resolution on model performance. We used a Canadian smart farm's yield data, mullispectral (MS) and red-green-blue (RGB) imagery captured by unmanned aerial vehicles (LAN's), and we trained deep neural networks (DNN), random forest (RF), and linear regression (LR) models using standard cross-validation and spatial cross-validation approaches. We found that multi-band datasets led to better performance than single-VI datasets. MS imagery led to generally better performance than RGB imagery High spatial resolution imagery led to better performance than lower spatial resolution imagery. RF was the best performing model while LR was the worst. The choice of RF's hyperparameters had more of an impact on performance when the number of features was small and less of an impact when the number of features was large or when the input dataset had a lot of spatial structure.
更多查看译文
关键词
precision agriculture,remote sensing,unmanned aerial vehicle,hyperparameter tuning,multispectral imagery,machine learning,deep learning,yield prediction,spatial data,spatial cross -validation
AI 理解论文
溯源树
样例
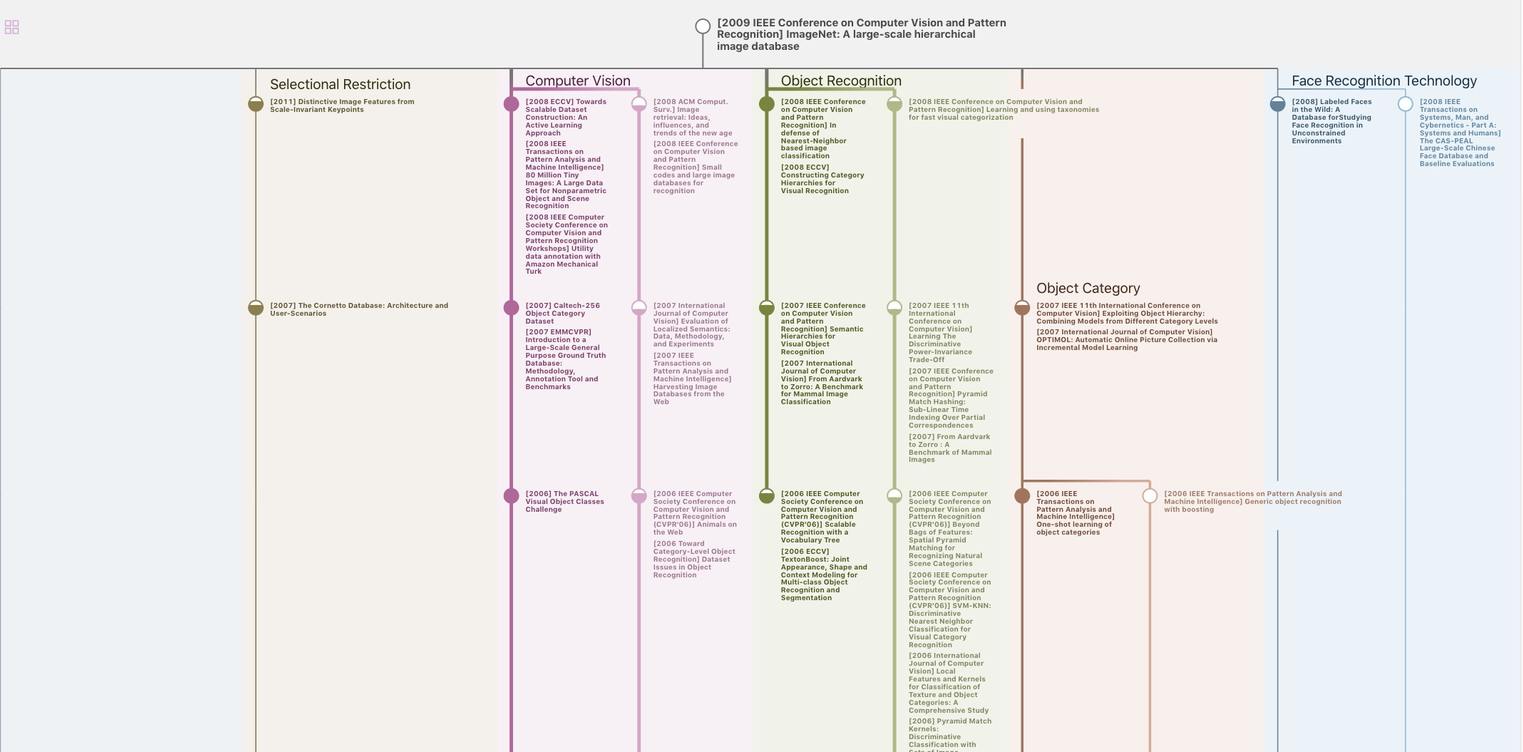
生成溯源树,研究论文发展脉络
Chat Paper
正在生成论文摘要