CVaDeS: A Conditional Variational Deep Survival Model for Survival Analysis
23RD IEEE INTERNATIONAL CONFERENCE ON DATA MINING, ICDM 2023(2023)
摘要
Transeriptome-hased survival modeling is a critical yet complicated task in cancer treatment due to the strong association between the prognosis of a patient and the progression of tumors based on heterogeneous molecular mechanisms. An effective prognosis model needs to consider the uncertainty that naturally stems from the high molecular heterogeneity together with numerous unforeseeable factors related to prognosis, and requires a perfect match between the selected covariates and the modeling approach of the joint distribution between covariates and right-censored survival time. Unfortunately, these necessary factors have not been fully considered in existing survival models. In this paper, we propose a novel conditional variational deep survival model (CVaDeS for short) for survival analysis. CVaDeS accurately predicts the risk values of patients and effectively distinguishes high -risk and low-risk individuals. Also, the proposed model CVaDeS can be effectively combined with Cox feature selection for survival analysis. Extensive experimental results demonstrate that the combination of Cox feature selection with CVaDeS significantly improves the predictive performance of the model, surpassing baseline models.
更多查看译文
关键词
Survival Analysis,Gene Screening,Conditional Variational Autoencoder
AI 理解论文
溯源树
样例
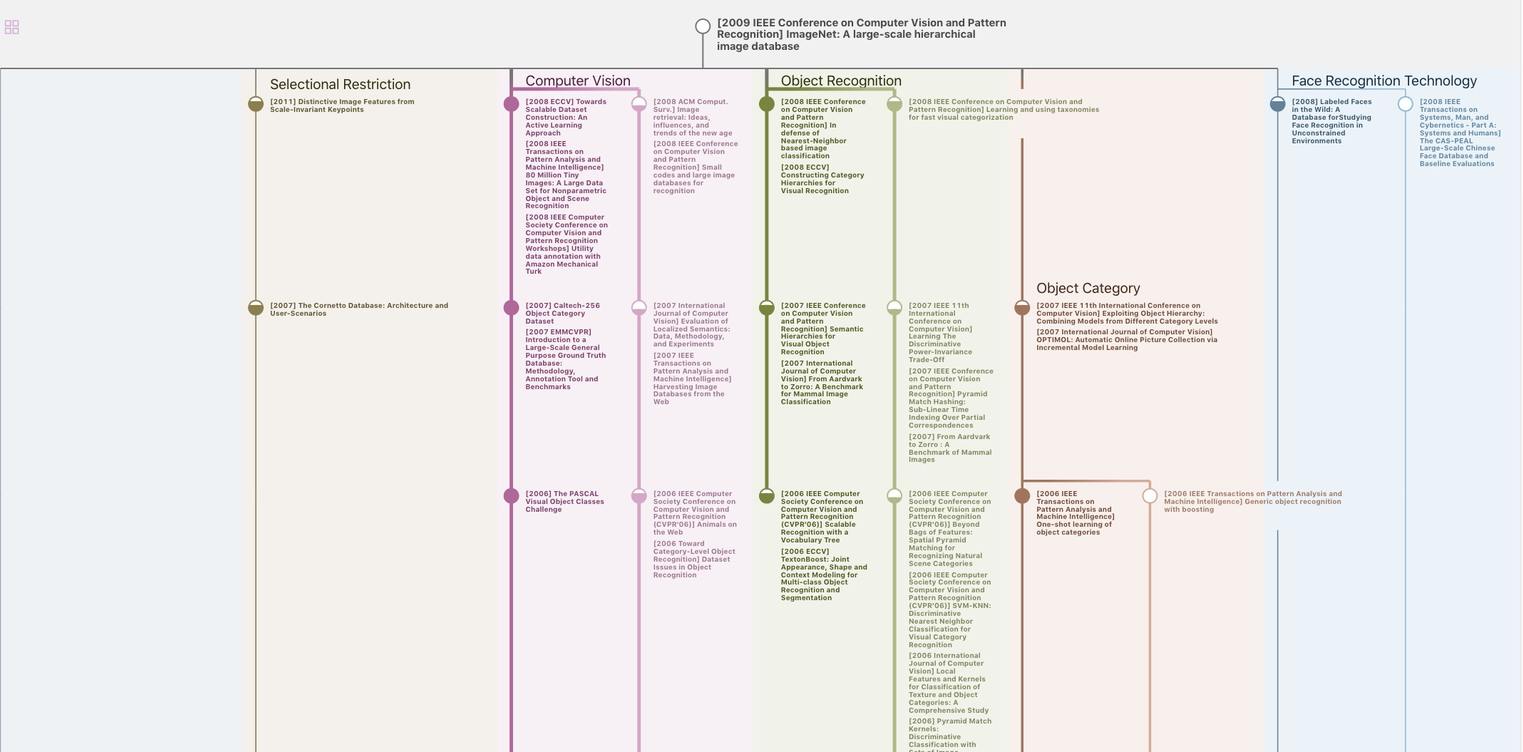
生成溯源树,研究论文发展脉络
Chat Paper
正在生成论文摘要