Efficient Cardinality and Cost Estimation with Bidirectional Compressor -based Ensemble Learning
23RD IEEE INTERNATIONAL CONFERENCE ON DATA MINING, ICDM 2023(2023)
摘要
Query optimization is of great importance for the performance of a database, in which cardinality and cost estimation have a pivotal role. To enable accurate cardinality and cost estimation, we propose a novel framework based on bidirectional compressor and ensemble networks called BICE. In particular, we design a feature extractor composed of four sub-encoders, which can extract various types of information in a query plan tree and hybrid learning strategies for encoding. We encode joins based on a graph embedding method and design parallel networks for filters to improve the encoding efficiency. Then we propose a bidirectional LSTM-based compressor to learn the encoding and obtain fixed-length vectors, reducing the learning difficulty of the estimation model. Finally, we propose different data sampling strategies based on Bayesian neural networks and active learning, and an ensemble model is established based on transfer learning, which enables accurate estimation and adaptation to large-scale data queries. Extensive experiments offer insight into the effectiveness and efficiency of the proposed framework.
更多查看译文
关键词
Terms Cardinality estimation,Cost estimation,Query optimization
AI 理解论文
溯源树
样例
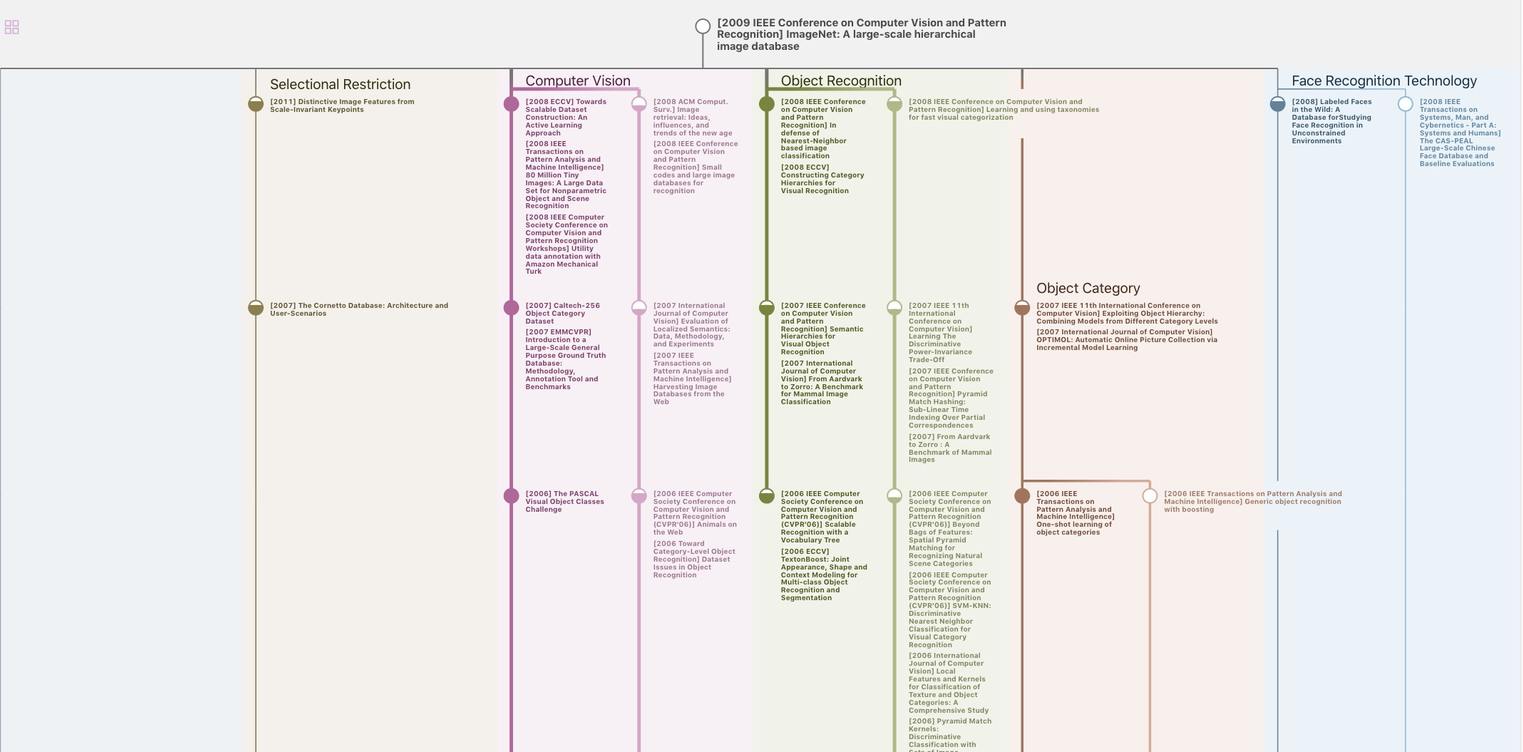
生成溯源树,研究论文发展脉络
Chat Paper
正在生成论文摘要