Variational mode decomposition-based EEG analysis for the classification of disorders of consciousness
FRONTIERS IN NEUROSCIENCE(2024)
摘要
Aberrant alterations in any of the two dimensions of consciousness, namely awareness and arousal, can lead to the emergence of disorders of consciousness (DOC). The development of DOC may arise from more severe or targeted lesions in the brain, resulting in widespread functional abnormalities. However, when it comes to classifying patients with disorders of consciousness, particularly utilizing resting-state electroencephalogram (EEG) signals through machine learning methods, several challenges surface. The non-stationarity and intricacy of EEG data present obstacles in understanding neuronal activities and achieving precise classification. To address these challenges, this study proposes variational mode decomposition (VMD) of EEG before feature extraction along with machine learning models. By decomposing preprocessed EEG signals into specified modes using VMD, features such as sample entropy, spectral entropy, kurtosis, and skewness are extracted across these modes. The study compares the performance of the features extracted from VMD-based approach with the frequency band-based approach and also the approach with features extracted from raw-EEG. The classification process involves binary classification between unresponsive wakefulness syndrome (UWS) and the minimally conscious state (MCS), as well as multi-class classification (coma vs. UWS vs. MCS). Kruskal-Wallis test was applied to determine the statistical significance of the features and features with a significance of p < 0.05 were chosen for a second round of classification experiments. Results indicate that the VMD-based features outperform the features of other two approaches, with the ensemble bagged tree (EBT) achieving the highest accuracy of 80.5% for multi-class classification (the best in the literature) and 86.7% for binary classification. This approach underscores the potential of integrating advanced signal processing techniques and machine learning in improving the classification of patients with disorders of consciousness, thereby enhancing patient care and facilitating informed treatment decision-making.
更多查看译文
关键词
variational mode decomposition,disorders of consciousness,resting-state EEG,ensemble bagged tree,patient care,resource management,treatment options and rehabilitation strategies
AI 理解论文
溯源树
样例
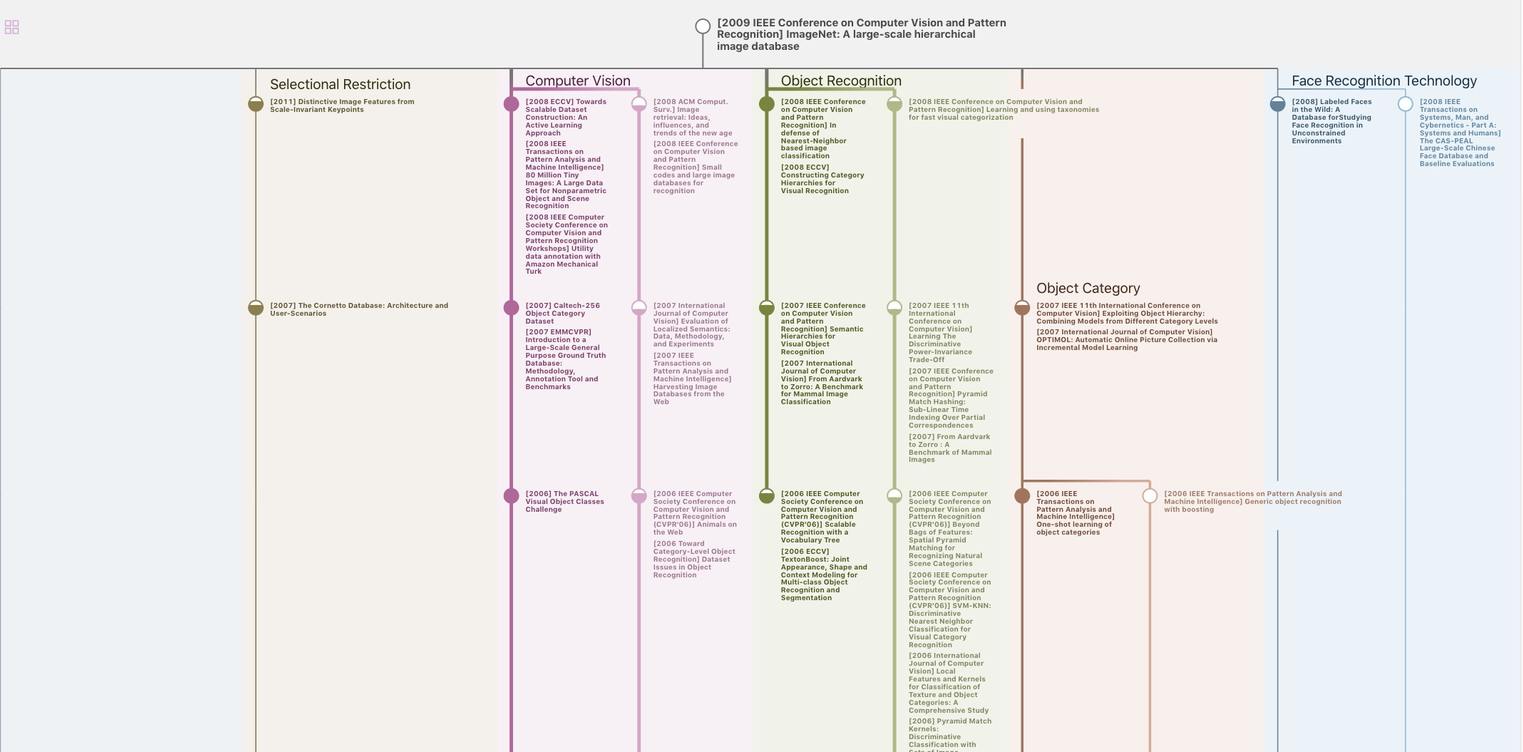
生成溯源树,研究论文发展脉络
Chat Paper
正在生成论文摘要