Global Information Embedding Network for Few-Shot Learning.
IEEE Signal Processing Letters(2024)
摘要
Few-shot learning aims to recognize new objects using a few labeled samples. Metric-based methods can effectively achieve this goal by building an appropriate feature space, for which a convolutional neural network (CNN) often acts as the backbone network. Unfortunately, CNN is adept at processing local spatial or channel neighborhood information, resulting in a lack of images’ global representation. In this letter, a global information embedding network (GIEN) is built for few-shot learning tasks. We have made two improvements in GIEN: one is the global information embedding (GIE) block, which captures the long-range dependencies of the images by a learnable frequency filter; the other is the two-branch training strategy, which combines cross-entropy loss and supervised contrastive loss to further promote discriminative features. Equipped with GIE, the proposed two-branch framework can obtain a stronger feature representation and achieve competitive performance on few-shot classification benchmark datasets such as
mini
ImageNet and
tiered
ImageNet.
更多查看译文
关键词
Few-shot learning,meta-learning,global informa- tion,attention mechanism,contrastive learning
AI 理解论文
溯源树
样例
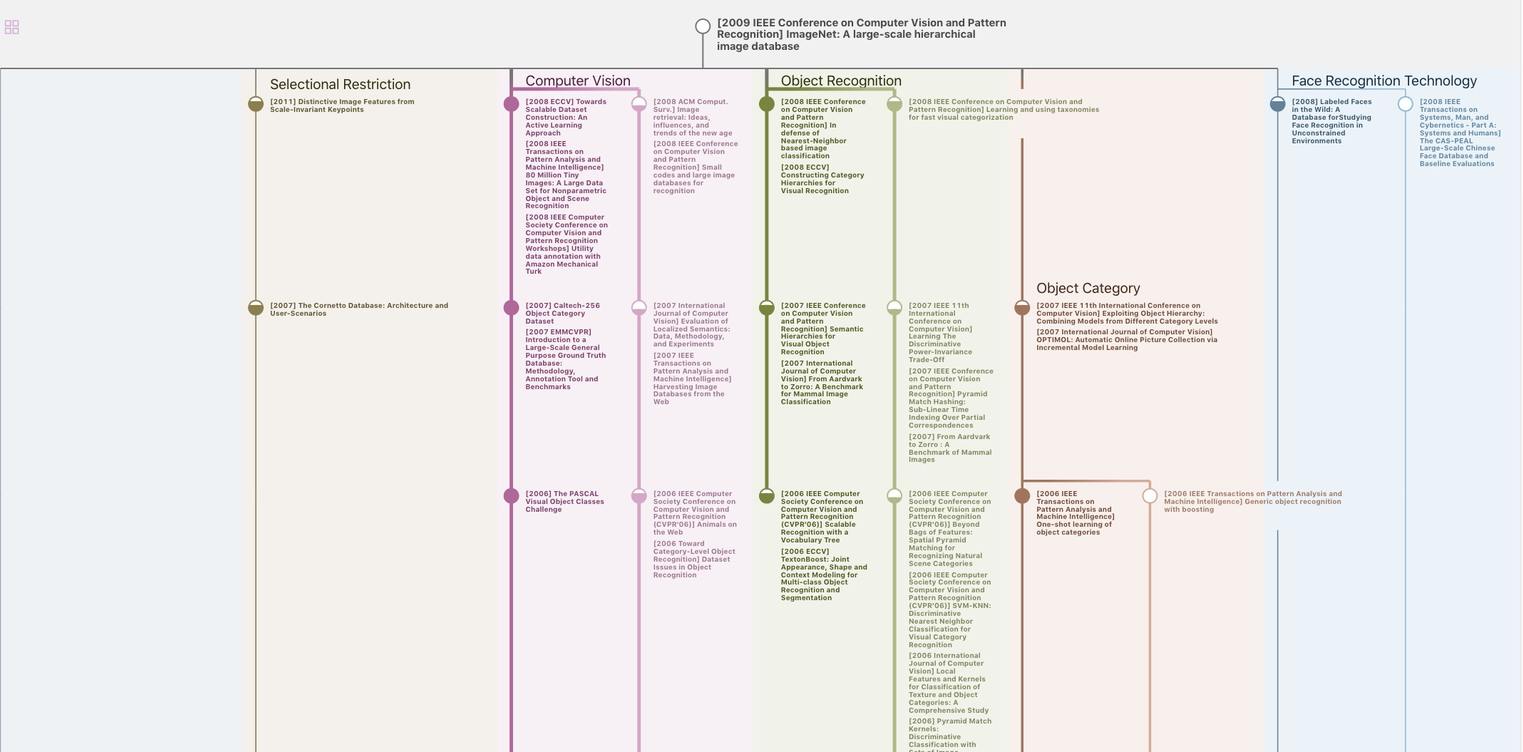
生成溯源树,研究论文发展脉络
Chat Paper
正在生成论文摘要