Iterative Self-Supervised Learning for Legal Similar Case Retrieval.
IEEE Access(2024)
摘要
In the realm of legal artificial intelligence (AI), the spotlight has been cast on its remarkable precision and efficiency, especially in tasks such as similar case retrieval where the identification of pertinent cases in response to a given query is of paramount importance. This task, distinct from traditional text retrieval, presents a set of unique challenges that necessitate the availability of high-quality, annotated datasets to facilitate efficient model training. The intricacies of handling extended queries and candidate documents, coupled with the varied interpretations of similarity, further compound the complexity of this endeavor. This study introduces an innovative training approach, combining dense and sparse retrieval methods. Utilizing a sparse retrieval model, we extract unlabeled data from extensive legal cases. Subsequently, a dense retrieval model screens this data, merging it with labeled data to create pseudo-labeled data, iteratively training until convergence. The results demonstrate exceptional performance in the Chinese law retrieval task dataset, showcasing a notable 3.66% precision enhancement and a substantial 3.62% improvement in mean average precision (MAP). However, the dataset’s imbalance across different charges of cases poses a challenge, potentially affecting retrieval performance for long-tailed legal cases. Nonetheless, these outcomes signify accelerated and more efficient retrieval of similar cases for legal professionals. Additionally, they provide high-quality references for non-legal individuals lacking expertise in the field.
更多查看译文
关键词
Legal information retrieval,similar case retrieval,iterative training,self-supervised learning
AI 理解论文
溯源树
样例
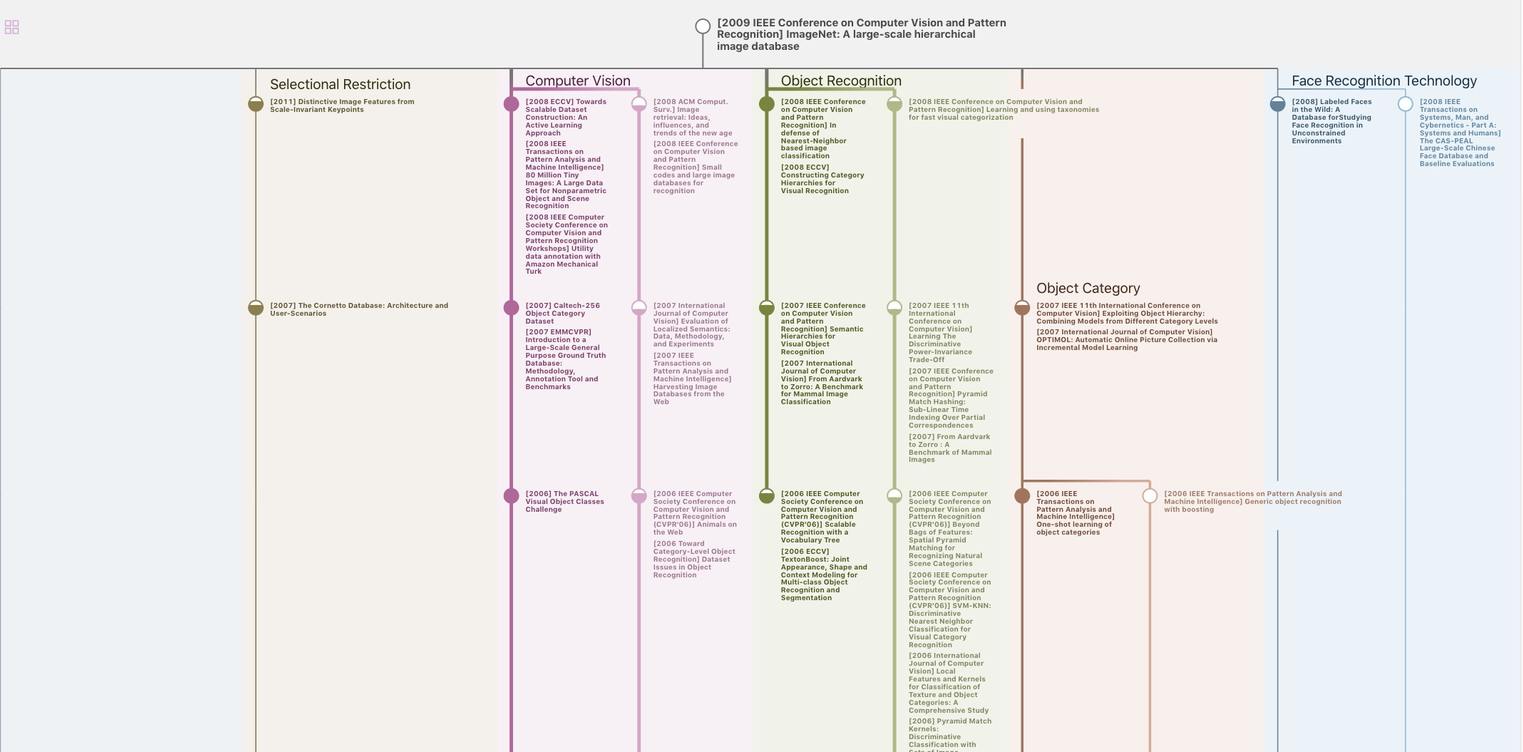
生成溯源树,研究论文发展脉络
Chat Paper
正在生成论文摘要