DMPNet: Distributed Multi-Scale Pyramid Network for Real-Time Semantic Segmentation.
IEEE Access(2024)
摘要
In semantic segmentation, an input image is partitioned into multiple meaningful segments each corresponding to a specific object or region. Multi-scale context plays a vital role in the accurate recognition of objects of different sizes and hence is key to overall accuracy enhancement. To achieve this goal, we introduce a novel strategy called Distributed Multi-scale Pyramid Pooling (DMPP) to extract multi-scale context at multiple levels of feature hierarchy. More specifically, we employ Pyramid Pooling Modules (PPM) in a distributed fashion after all three stages during the encoding phase. This enhances the feature representation capability of the network and leads to better performance. To extract context at a more granular level, we propose an Efficient Multi-scale Context Aggregation (EMCA) module which uses a combination of small and large kernels with large and small dilation rates, respectively. This alleviates the problem of sparse sampling and leads to consistent recognition of different regions. Apart from model accuracy, small model size and efficient execution are critically important for real-time mobile applications. To achieve it, we employ a resource-friendly combination of depthwise and factorized convolutions in the EMCA module to drastically reduce the number of parameters without significantly compromising the accuracy. Based on the EMCA module and DMPP, we propose a lightweight and real-time Distributed Multi-scale Pyramid Network (DMPNet) that achieves an excellent accuracy-efficiency trade-off. We also conducted extensive experiments on both driving datasets (i.e., Cityscapes and CamVid) and a general-purpose dataset (i.e., ADE20K) to show the effectiveness of the proposed method.
更多查看译文
关键词
Semantic segmentation,deep learning,real-time processing,autonomous driving,resource-constrained
AI 理解论文
溯源树
样例
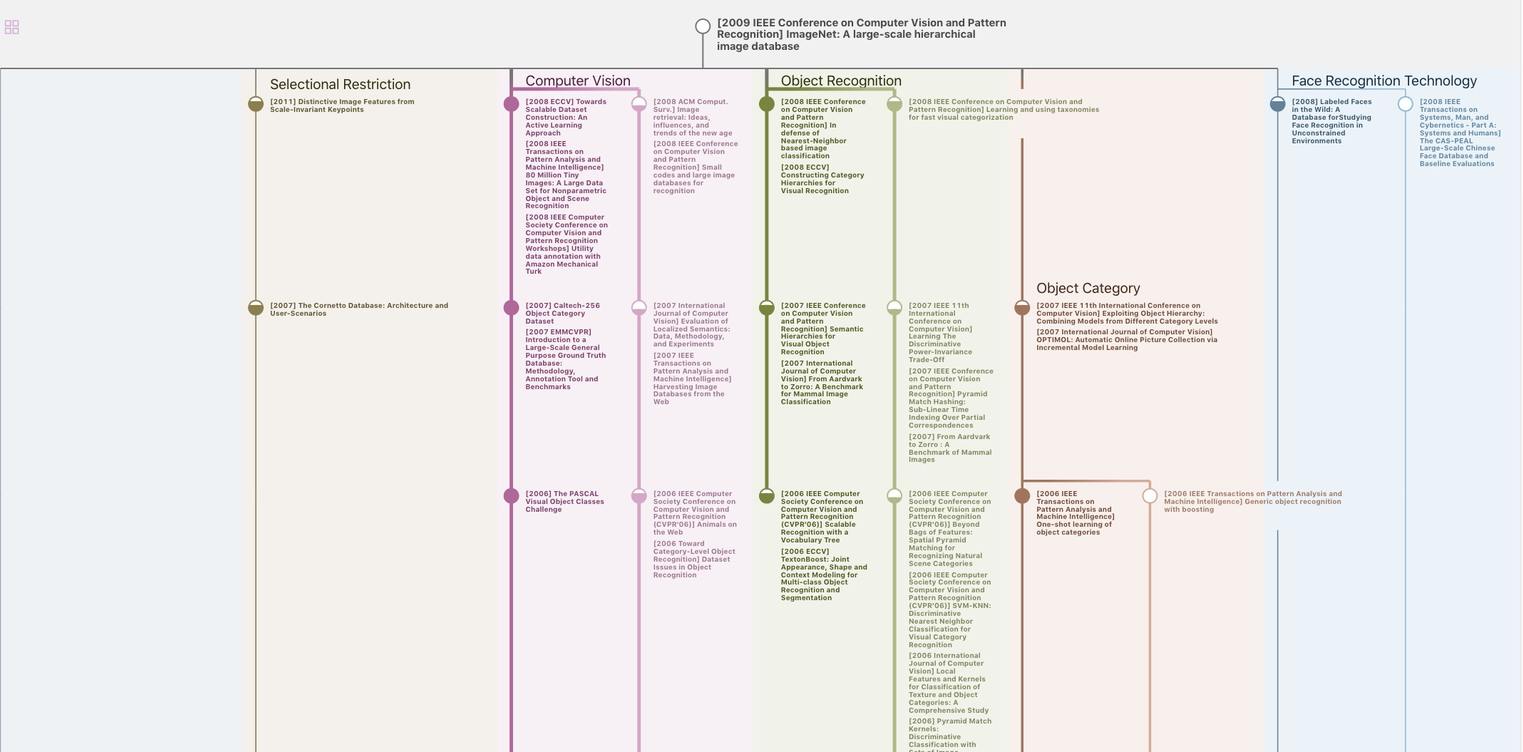
生成溯源树,研究论文发展脉络
Chat Paper
正在生成论文摘要