Towards mitigating uncann(eye)ness in face swaps via gaze-centric loss terms
Computers & Graphics(2024)
摘要
Advances in face swapping have enabled the automatic generation of highly realistic faces. Yet face swaps are perceived differently than when looking at real faces, with key differences in viewer behavior surrounding the eyes. Face swapping algorithms generally place no emphasis on the eyes, relying on pixel or feature matching losses that consider the entire face to guide the training process. We further investigate viewer perception of face swaps, focusing our analysis on the presence of an uncanny valley effect. We additionally propose a novel loss equation for the training of face swapping models, leveraging a pretrained gaze estimation network to directly improve representation of the eyes. We confirm that viewed face swaps do elicit uncanny responses from viewers. Our proposed improvements significant reduce viewing angle errors between face swaps and their source material. Our method additionally reduces the prevalence of the eyes as a deciding factor when viewers perform deepfake detection tasks. Our findings have implications on face swapping for special effects, as digital avatars, as privacy mechanisms, and more; negative responses from users could limit effectiveness in said applications. Our gaze improvements are a first step towards alleviating negative viewer perceptions via a targeted approach.
更多查看译文
关键词
Face swapping,Gaze estimation,Perception
AI 理解论文
溯源树
样例
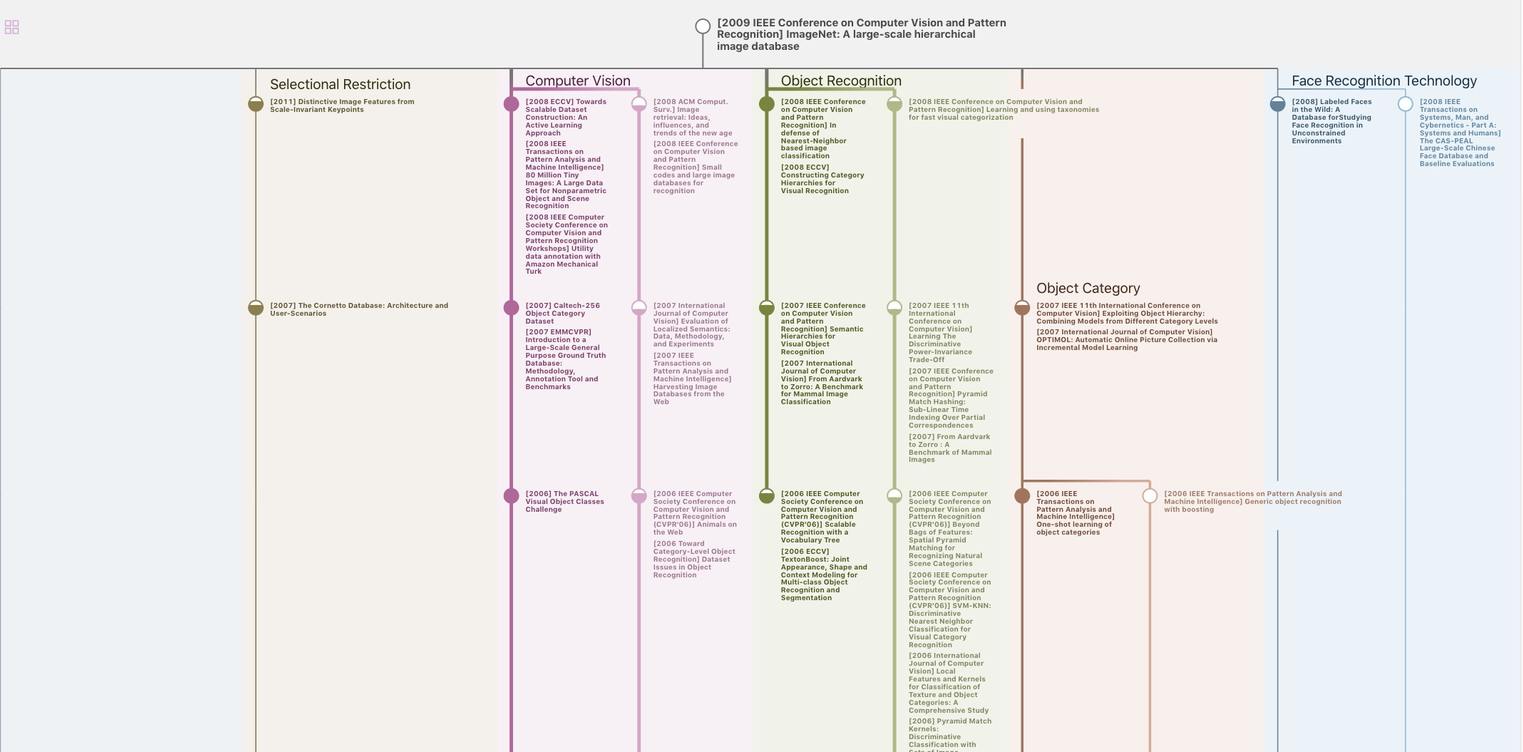
生成溯源树,研究论文发展脉络
Chat Paper
正在生成论文摘要