Enhancing Neural Subset Selection: Integrating Background Information into Set Representations
CoRR(2024)
摘要
Learning neural subset selection tasks, such as compound selection in
AI-aided drug discovery, have become increasingly pivotal across diverse
applications. The existing methodologies in the field primarily concentrate on
constructing models that capture the relationship between utility function
values and subsets within their respective supersets. However, these approaches
tend to overlook the valuable information contained within the superset when
utilizing neural networks to model set functions. In this work, we address this
oversight by adopting a probabilistic perspective. Our theoretical findings
demonstrate that when the target value is conditioned on both the input set and
subset, it is essential to incorporate an invariant sufficient
statistic of the superset into the subset of interest for effective learning.
This ensures that the output value remains invariant to permutations of the
subset and its corresponding superset, enabling identification of the specific
superset from which the subset originated. Motivated by these insights, we
propose a simple yet effective information aggregation module designed to merge
the representations of subsets and supersets from a permutation invariance
perspective. Comprehensive empirical evaluations across diverse tasks and
datasets validate the enhanced efficacy of our approach over conventional
methods, underscoring the practicality and potency of our proposed strategies
in real-world contexts.
更多查看译文
关键词
Neural Set Function,Hierarchical Structure,Invariance,Subset Selection
AI 理解论文
溯源树
样例
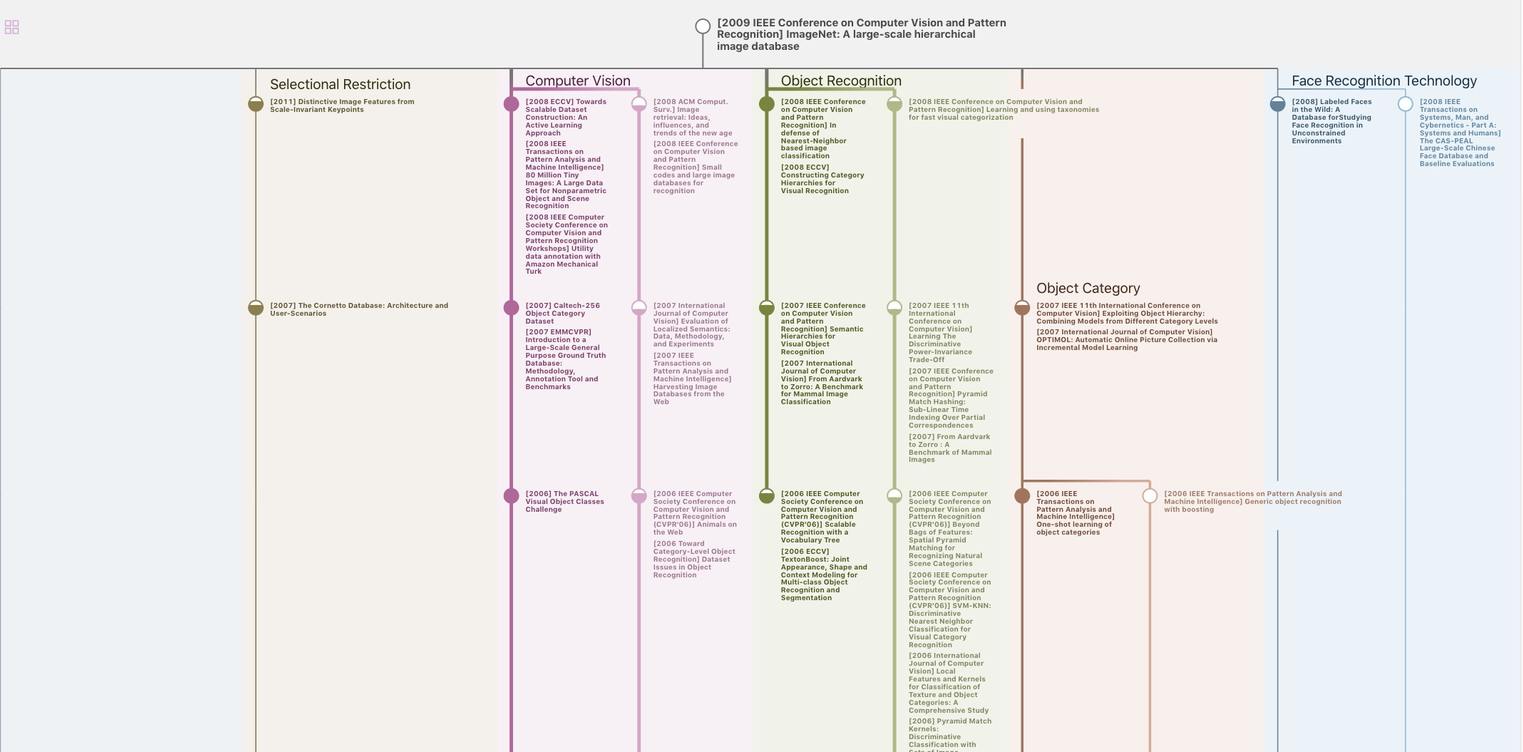
生成溯源树,研究论文发展脉络
Chat Paper
正在生成论文摘要