Non-Stationary Latent Auto-Regressive Bandits
CoRR(2024)
摘要
We consider the stochastic multi-armed bandit problem with non-stationary
rewards. We present a novel formulation of non-stationarity in the environment
where changes in the mean reward of the arms over time are due to some unknown,
latent, auto-regressive (AR) state of order k. We call this new environment
the latent AR bandit. Different forms of the latent AR bandit appear in many
real-world settings, especially in emerging scientific fields such as
behavioral health or education where there are few mechanistic models of the
environment. If the AR order k is known, we propose an algorithm that
achieves Õ(k√(T)) regret in this setting. Empirically, our
algorithm outperforms standard UCB across multiple non-stationary environments,
even if k is mis-specified.
更多查看译文
AI 理解论文
溯源树
样例
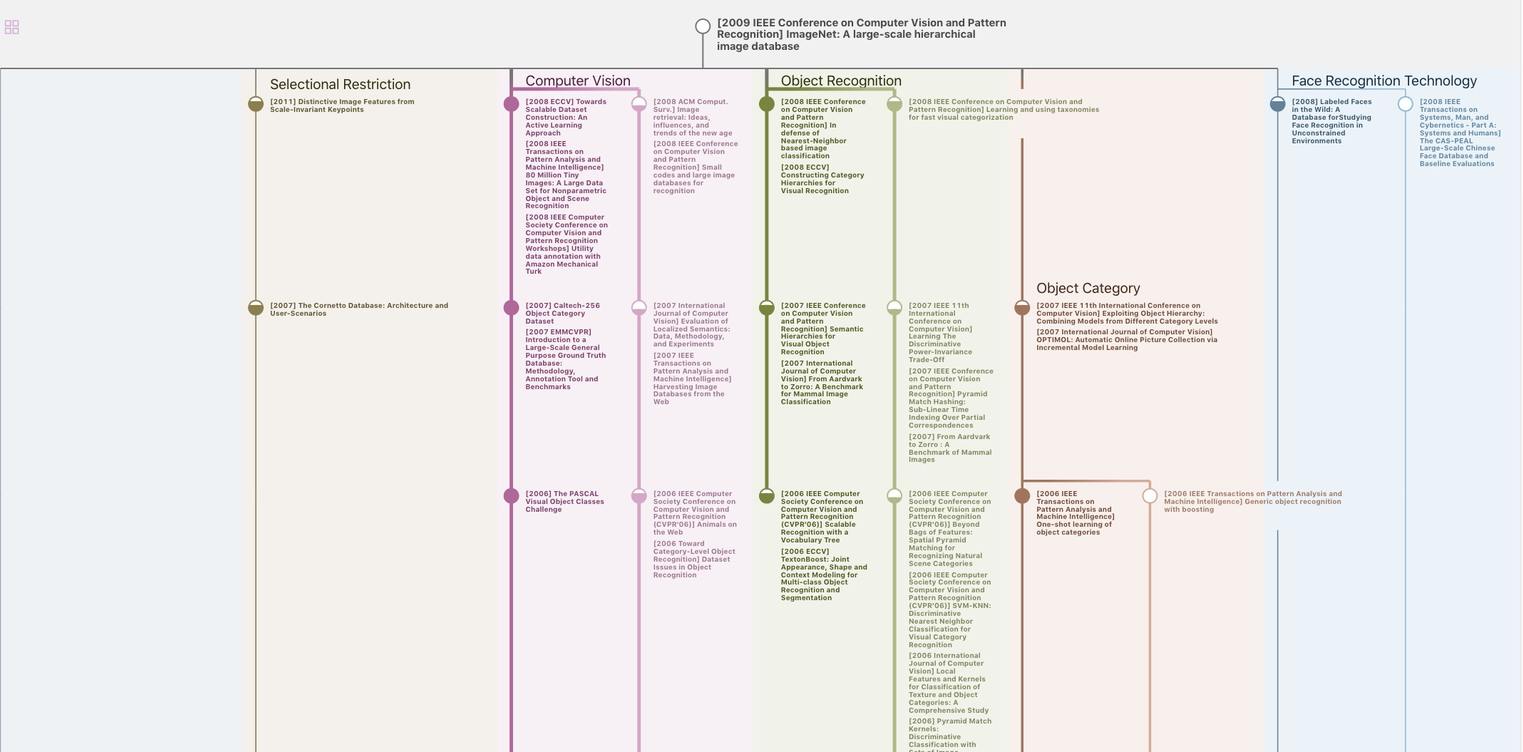
生成溯源树,研究论文发展脉络
Chat Paper
正在生成论文摘要