PROSAC: Provably Safe Certification for Machine Learning Models under Adversarial Attacks
CoRR(2024)
摘要
It is widely known that state-of-the-art machine learning models, including
vision and language models, can be seriously compromised by adversarial
perturbations. It is therefore increasingly relevant to develop capabilities to
certify their performance in the presence of the most effective adversarial
attacks. Our paper offers a new approach to certify the performance of machine
learning models in the presence of adversarial attacks with population level
risk guarantees. In particular, we introduce the notion of (α,ζ)
machine learning model safety. We propose a hypothesis testing procedure, based
on the availability of a calibration set, to derive statistical guarantees
providing that the probability of declaring that the adversarial (population)
risk of a machine learning model is less than α (i.e. the model is
safe), while the model is in fact unsafe (i.e. the model adversarial population
risk is higher than α), is less than ζ. We also propose Bayesian
optimization algorithms to determine efficiently whether a machine learning
model is (α,ζ)-safe in the presence of an adversarial attack, along
with statistical guarantees. We apply our framework to a range of machine
learning models including various sizes of vision Transformer (ViT) and ResNet
models impaired by a variety of adversarial attacks, such as AutoAttack,
SquareAttack and natural evolution strategy attack, to illustrate the operation
of our approach. Importantly, we show that ViT's are generally more robust to
adversarial attacks than ResNets, and ViT-large is more robust than smaller
models. Our approach goes beyond existing empirical adversarial risk-based
certification guarantees. It formulates rigorous (and provable) performance
guarantees that can be used to satisfy regulatory requirements mandating the
use of state-of-the-art technical tools.
更多查看译文
AI 理解论文
溯源树
样例
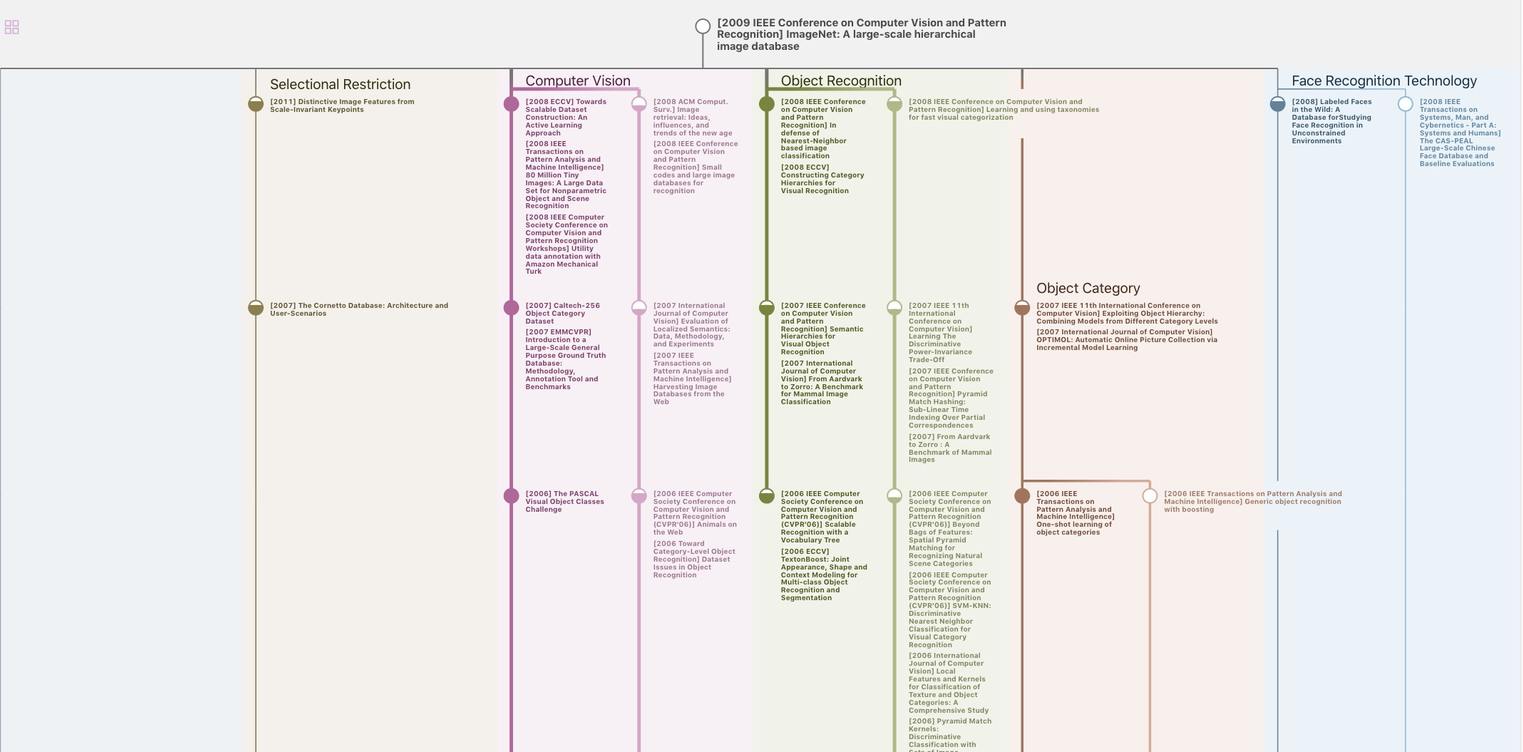
生成溯源树,研究论文发展脉络
Chat Paper
正在生成论文摘要