Preference Poisoning Attacks on Reward Model Learning
CoRR(2024)
摘要
Learning utility, or reward, models from pairwise comparisons is a
fundamental component in a number of application domains. These approaches
inherently entail collecting preference information from people, with feedback
often provided anonymously. Since preferences are subjective, there is no gold
standard to compare against; yet, reliance of high-impact systems on preference
learning creates a strong motivation for malicious actors to skew data
collected in this fashion to their ends. We investigate the nature and extent
of this vulnerability systematically by considering a threat model in which an
attacker can flip a small subset of preference comparisons with the goal of
either promoting or demoting a target outcome. First, we propose two classes of
algorithmic approaches for these attacks: a principled gradient-based
framework, and several variants of rank-by-distance methods. Next, we
demonstrate the efficacy of best attacks in both these classes in successfully
achieving malicious goals on datasets from three diverse domains: autonomous
control, recommendation system, and textual prompt-response preference
learning. We find that the best attacks are often highly successful, achieving
in the most extreme case 100
However, which attack is best can vary significantly across domains,
demonstrating the value of our comprehensive vulnerability analysis that
involves several classes of attack algorithms. In addition, we observe that the
simpler and more scalable rank-by-distance approaches are often competitive
with the best, and on occasion significantly outperform gradient-based methods.
Finally, we show that several state-of-the-art defenses against other classes
of poisoning attacks exhibit, at best, limited efficacy in our setting.
更多查看译文
AI 理解论文
溯源树
样例
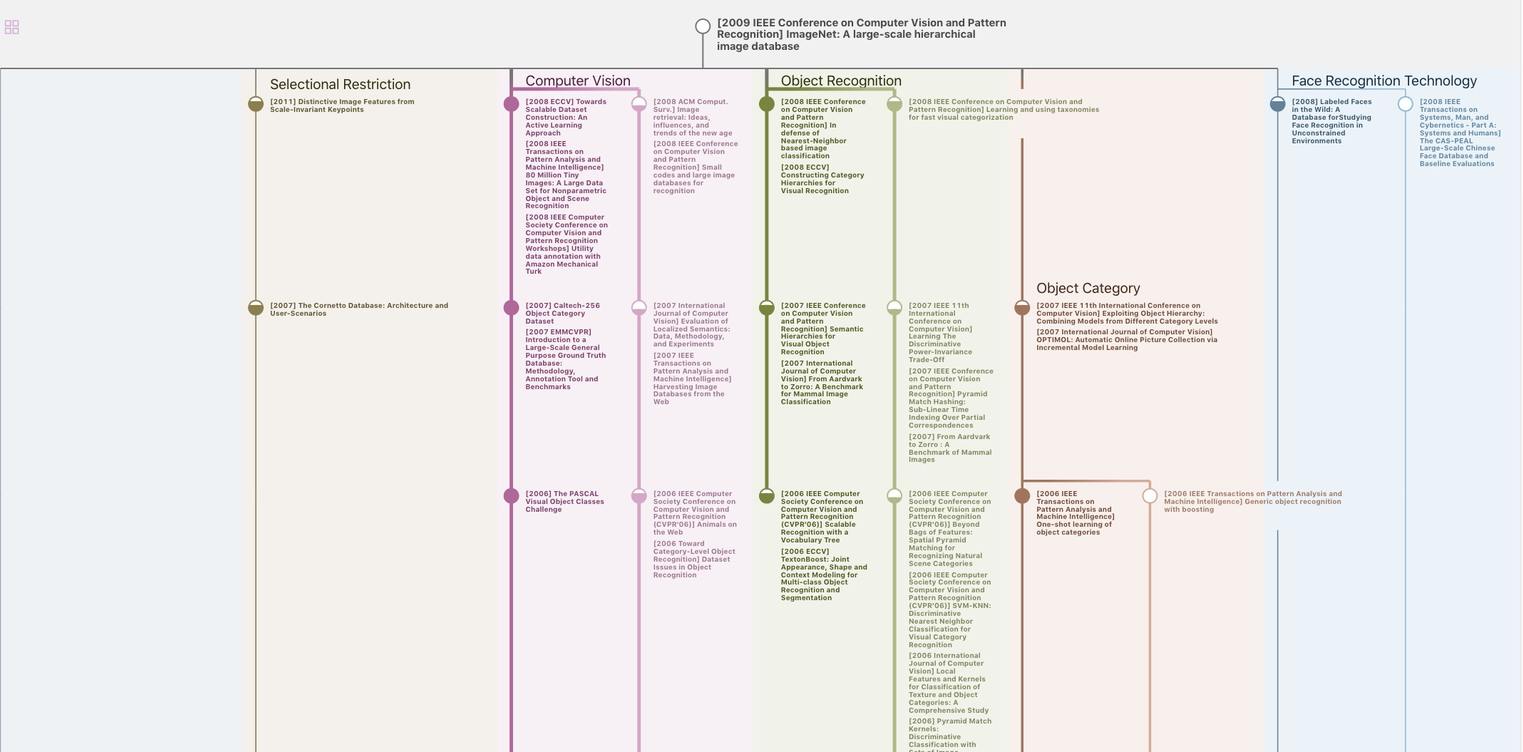
生成溯源树,研究论文发展脉络
Chat Paper
正在生成论文摘要