σ-zero: Gradient-based Optimization of ℓ_0-norm Adversarial Examples
arxiv(2024)
摘要
Evaluating the adversarial robustness of deep networks to gradient-based
attacks is challenging. While most attacks consider ℓ_2- and
ℓ_∞-norm constraints to craft input perturbations, only a few
investigate sparse ℓ_1- and ℓ_0-norm attacks. In particular,
ℓ_0-norm attacks remain the least studied due to the inherent complexity
of optimizing over a non-convex and non-differentiable constraint. However,
evaluating adversarial robustness under these attacks could reveal weaknesses
otherwise left untested with more conventional ℓ_2- and ℓ_∞-norm
attacks. In this work, we propose a novel ℓ_0-norm attack, called
σ-zero, which leverages an ad hoc differentiable approximation of the
ℓ_0 norm to facilitate gradient-based optimization, and an adaptive
projection operator to dynamically adjust the trade-off between loss
minimization and perturbation sparsity. Extensive evaluations using MNIST,
CIFAR10, and ImageNet datasets, involving robust and non-robust models, show
that σ-zero finds minimum ℓ_0-norm adversarial examples without
requiring any time-consuming hyperparameter tuning, and that it outperforms all
competing sparse attacks in terms of success rate, perturbation size, and
scalability.
更多查看译文
AI 理解论文
溯源树
样例
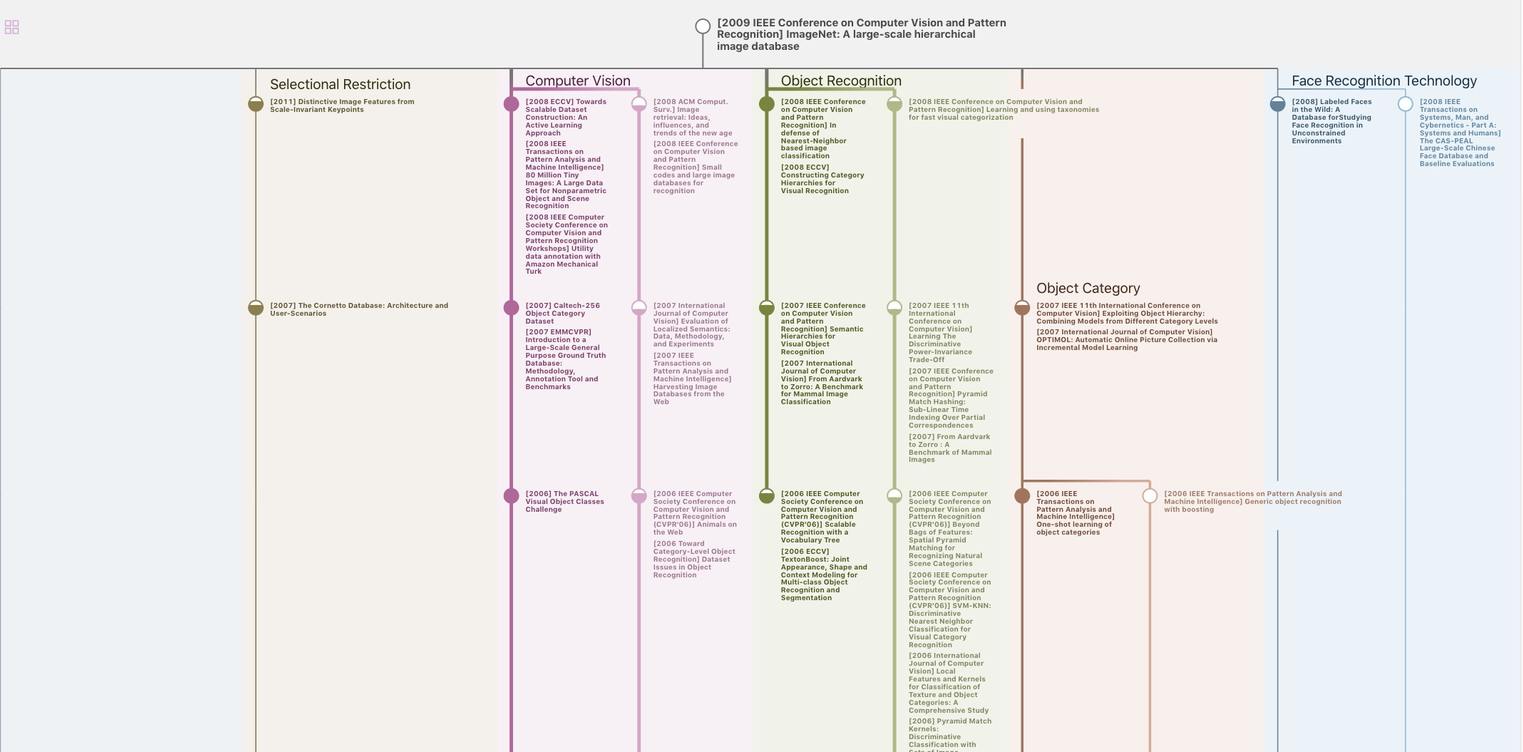
生成溯源树,研究论文发展脉络
Chat Paper
正在生成论文摘要