Question answering systems for health professionals at the point of care-a systematic review
JOURNAL OF THE AMERICAN MEDICAL INFORMATICS ASSOCIATION(2024)
摘要
Objectives Question answering (QA) systems have the potential to improve the quality of clinical care by providing health professionals with the latest and most relevant evidence. However, QA systems have not been widely adopted. This systematic review aims to characterize current medical QA systems, assess their suitability for healthcare, and identify areas of improvement.Materials and methods We searched PubMed, IEEE Xplore, ACM Digital Library, ACL Anthology, and forward and backward citations on February 7, 2023. We included peer-reviewed journal and conference papers describing the design and evaluation of biomedical QA systems. Two reviewers screened titles, abstracts, and full-text articles. We conducted a narrative synthesis and risk of bias assessment for each study. We assessed the utility of biomedical QA systems.Results We included 79 studies and identified themes, including question realism, answer reliability, answer utility, clinical specialism, systems, usability, and evaluation methods. Clinicians' questions used to train and evaluate QA systems were restricted to certain sources, types and complexity levels. No system communicated confidence levels in the answers or sources. Many studies suffered from high risks of bias and applicability concerns. Only 8 studies completely satisfied any criterion for clinical utility, and only 7 reported user evaluations. Most systems were built with limited input from clinicians.Discussion While machine learning methods have led to increased accuracy, most studies imperfectly reflected real-world healthcare information needs. Key research priorities include developing more realistic healthcare QA datasets and considering the reliability of answer sources, rather than merely focusing on accuracy.
更多查看译文
关键词
clinical decision support,question answering,evidence-based medicine,natural language processing,artificial intelligence
AI 理解论文
溯源树
样例
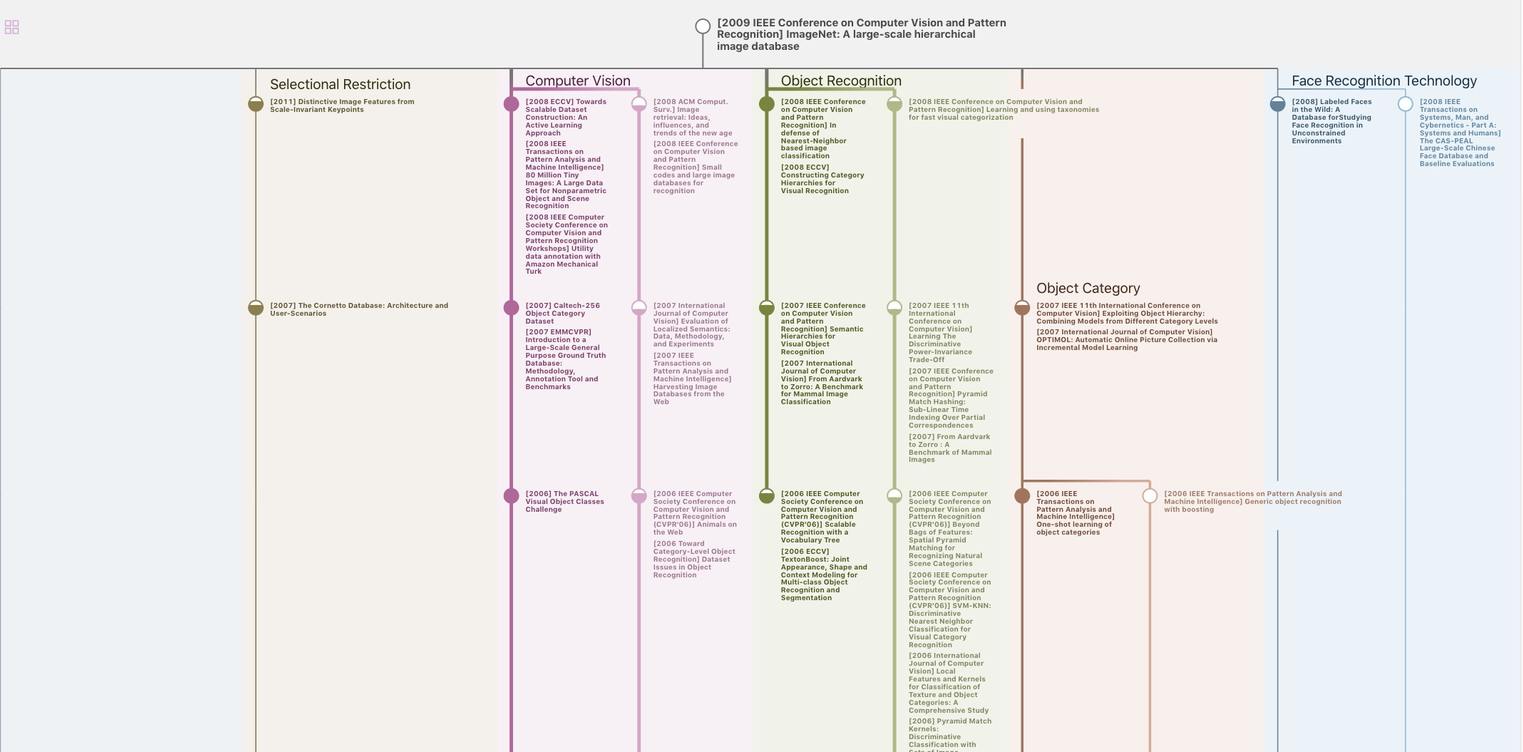
生成溯源树,研究论文发展脉络
Chat Paper
正在生成论文摘要