A Holistic Approach for Equity-aware Carbon Reduction of Ridesharing Platforms
ACM International Conference on Future Energy Systems(2024)
摘要
Ridesharing services have revolutionized personal mobility, offering
convenient on-demand transportation anytime. While early proponents of
ridesharing suggested that these services would reduce the overall carbon
emissions of the transportation sector, recent studies reported a type of
rebound effect showing substantial carbon emissions of ridesharing platforms,
mainly due to their deadhead miles traveled between two consecutive rides.
However, reducing deadhead miles' emissions can incur longer waiting times for
riders and starvation of ride assignments for some drivers. Therefore, any
efforts towards reducing the carbon emissions from ridesharing platforms must
consider the impact on the quality of service, e.g., waiting time, and on the
equitable distribution of rides across drivers. This paper proposes a holistic
approach to reduce the carbon emissions of ridesharing platforms while
minimizing the degradation in user waiting times and equitable ride assignments
across drivers. Towards this end, we decompose the global carbon reduction
problem into two sub-problems: carbon- and equity-aware ride assignment and
fuel-efficient routing. For the ride assignment problem, we consider the
trade-off between the amount of carbon reduction and the rider's waiting time
and propose simple yet efficient algorithms to handle the conflicting
trade-offs. For the routing problem, we analyze the impact of fuel-efficient
routing in reducing the carbon footprint, trip duration, and driver efficiency
of ridesharing platforms using route data from Google Maps. Our comprehensive
trace-driven experimental results show significant emissions reduction with a
minor increase in riders' waiting times. Finally, we release E^2-RideKit, a
toolkit that enables researchers to augment ridesharing datasets with emissions
and equity information for further research on emission analysis and platform
improvement.
更多查看译文
AI 理解论文
溯源树
样例
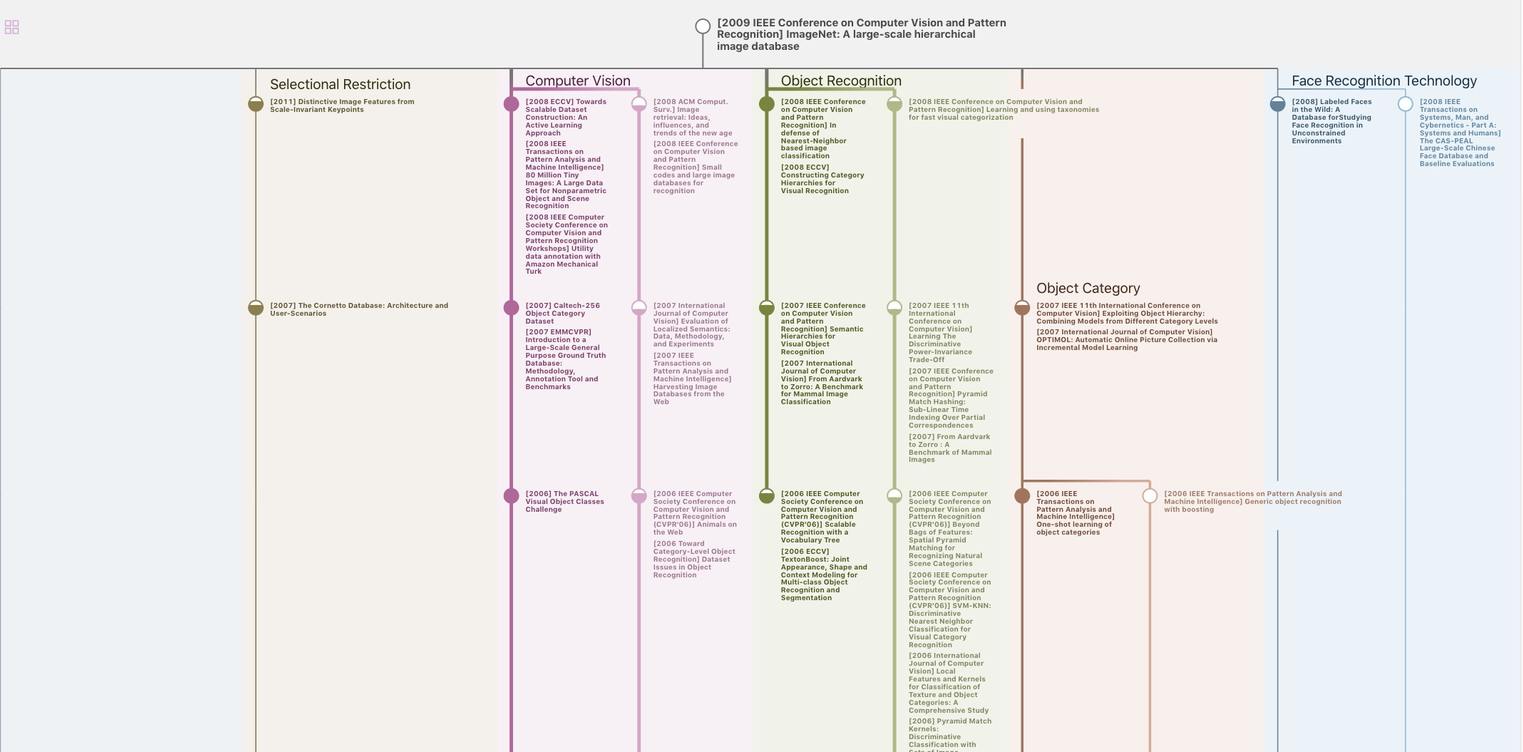
生成溯源树,研究论文发展脉络
Chat Paper
正在生成论文摘要