Online Uniform Risk Times Sampling: First Approximation Algorithms, Learning Augmentation with Full Confidence Interval Integration
CoRR(2024)
摘要
In digital health, the strategy of allocating a limited treatment budget
across available risk times is crucial to reduce user fatigue. This strategy,
however, encounters a significant obstacle due to the unknown actual number of
risk times, a factor not adequately addressed by existing methods lacking
theoretical guarantees. This paper introduces, for the first time, the online
uniform risk times sampling problem within the approximation algorithm
framework. We propose two online approximation algorithms for this problem, one
with and one without learning augmentation, and provide rigorous theoretical
performance guarantees for them using competitive ratio analysis. We assess the
performance of our algorithms using both synthetic experiments and a real-world
case study on HeartSteps mobile applications.
更多查看译文
AI 理解论文
溯源树
样例
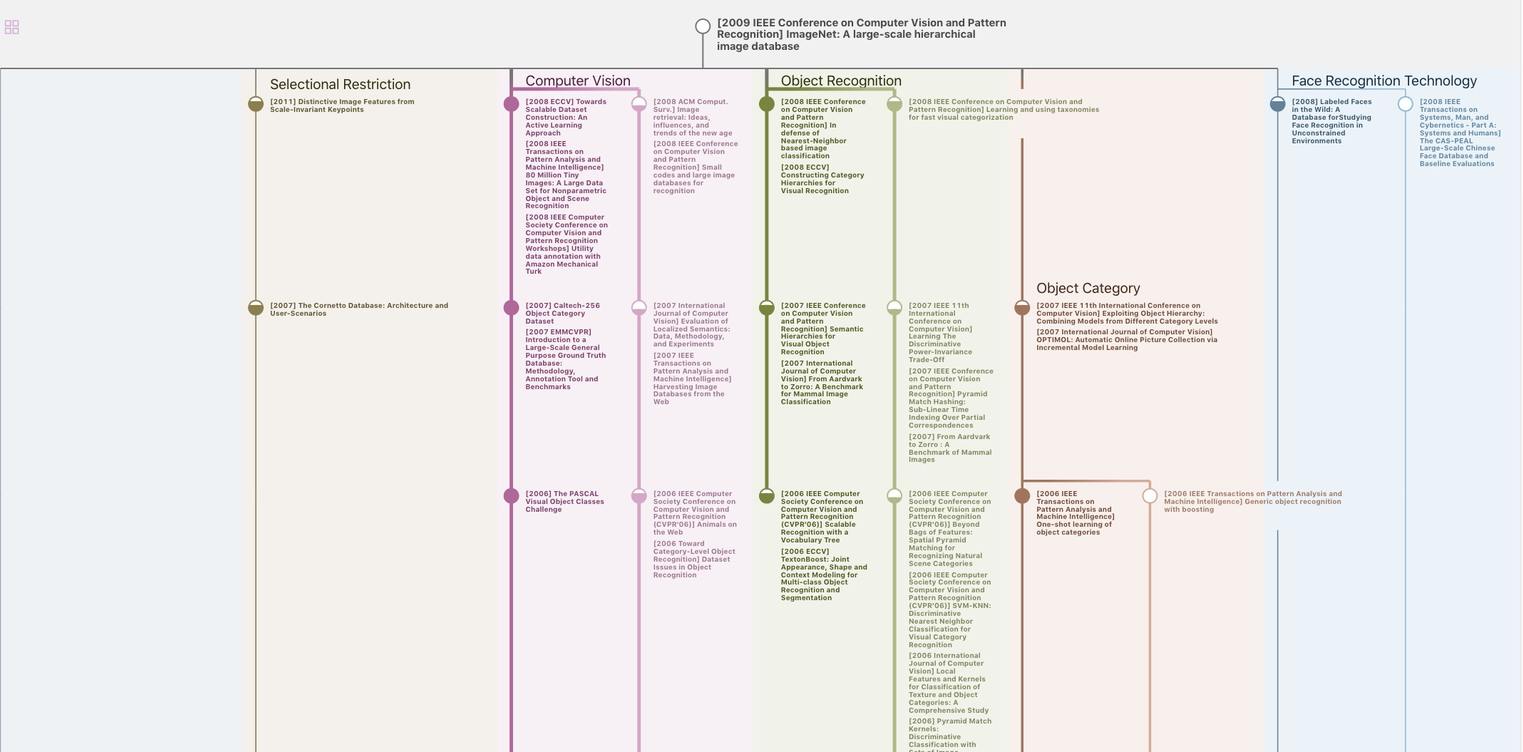
生成溯源树,研究论文发展脉络
Chat Paper
正在生成论文摘要