Experiment-driven atomistic materials modeling: A case study combining XPS and ML potentials to infer the structure of oxygen-rich amorphous carbon
arxiv(2024)
摘要
An important yet challenging aspect of atomistic materials modeling is
reconciling experimental and computational results. Conventional approaches
involve generating numerous configurations through molecular dynamics or Monte
Carlo structure optimization and selecting the one with the closest match to
experiment. However, this inefficient process is not guaranteed to succeed. We
introduce a general method to combine atomistic machine learning (ML) with
experimental observables that produces atomistic structures compatible with
experiment by design. We use this approach in combination with grand-canonical
Monte Carlo within a modified Hamiltonian formalism, to generate configurations
that agree with experimental data and are chemically sound (low in energy). We
apply our approach to understand the atomistic structure of oxygenated
amorphous carbon (a-CO_x), an intriguing carbon-based material, to answer
the question of how much oxygen can be added to carbon before it fully
decomposes into CO and CO_2. Utilizing an ML-based X-ray photoelectron
spectroscopy (XPS) model trained from GW and density functional theory (DFT)
data, in conjunction with an ML interatomic potential, we identify a-CO_x
structures compliant with experimental XPS predictions that are also
energetically favorable with respect to DFT. Employing a network analysis, we
accurately deconvolve the XPS spectrum into motif contributions, both revealing
the inaccuracies inherent to experimental XPS interpretation and granting us
atomistic insight into the structure of a-CO_x. This method generalizes to
multiple experimental observables and allows for the elucidation of the
atomistic structure of materials directly from experimental data, thereby
enabling experiment-driven materials modeling with a degree of realism
previously out of reach.
更多查看译文
AI 理解论文
溯源树
样例
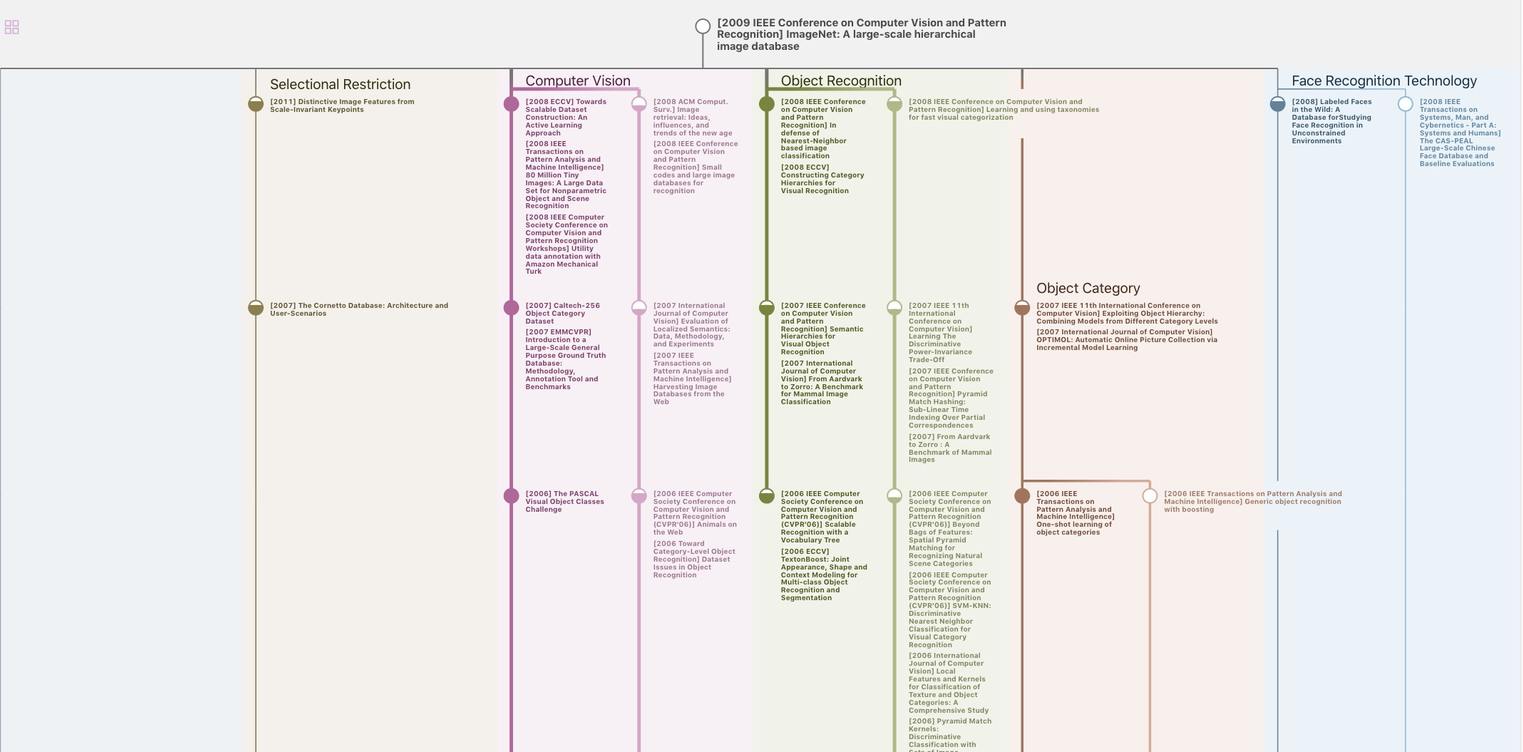
生成溯源树,研究论文发展脉络
Chat Paper
正在生成论文摘要