Diagnosing and tracking depression based on eye movement in response to virtual reality
FRONTIERS IN PSYCHIATRY(2024)
摘要
Introduction Depression is a prevalent mental illness that is primarily diagnosed using psychological and behavioral assessments. However, these assessments lack objective and quantitative indices, making rapid and objective detection challenging. In this study, we propose a novel method for depression detection based on eye movement data captured in response to virtual reality (VR).Methods Eye movement data was collected and used to establish high-performance classification and prediction models. Four machine learning algorithms, namely eXtreme Gradient Boosting (XGBoost), multilayer perceptron (MLP), Support Vector Machine (SVM), and Random Forest, were employed. The models were evaluated using five-fold cross-validation, and performance metrics including accuracy, precision, recall, area under the curve (AUC), and F1-score were assessed. The predicted error for the Patient Health Questionnaire-9 (PHQ-9) score was also determined.Results The XGBoost model achieved a mean accuracy of 76%, precision of 94%, recall of 73%, and AUC of 82%, with an F1-score of 78%. The MLP model achieved a classification accuracy of 86%, precision of 96%, recall of 91%, and AUC of 86%, with an F1-score of 92%. The predicted error for the PHQ-9 score ranged from -0.6 to 0.6.To investigate the role of computerized cognitive behavioral therapy (CCBT) in treating depression, participants were divided into intervention and control groups. The intervention group received CCBT, while the control group received no treatment. After five CCBT sessions, significant changes were observed in the eye movement indices of fixation and saccade, as well as in the PHQ-9 scores. These two indices played significant roles in the predictive model, indicating their potential as biomarkers for detecting depression symptoms.Discussion The results suggest that eye movement indices obtained using a VR eye tracker can serve as useful biomarkers for detecting depression symptoms. Specifically, the fixation and saccade indices showed promise in predicting depression. Furthermore, CCBT demonstrated effectiveness in treating depression, as evidenced by the observed changes in eye movement indices and PHQ-9 scores. In conclusion, this study presents a novel approach for depression detection using eye movement data captured in VR. The findings highlight the potential of eye movement indices as biomarkers and underscore the effectiveness of CCBT in treating depression.
更多查看译文
关键词
depression,diagnose,XGBoost,MLP,CCBT
AI 理解论文
溯源树
样例
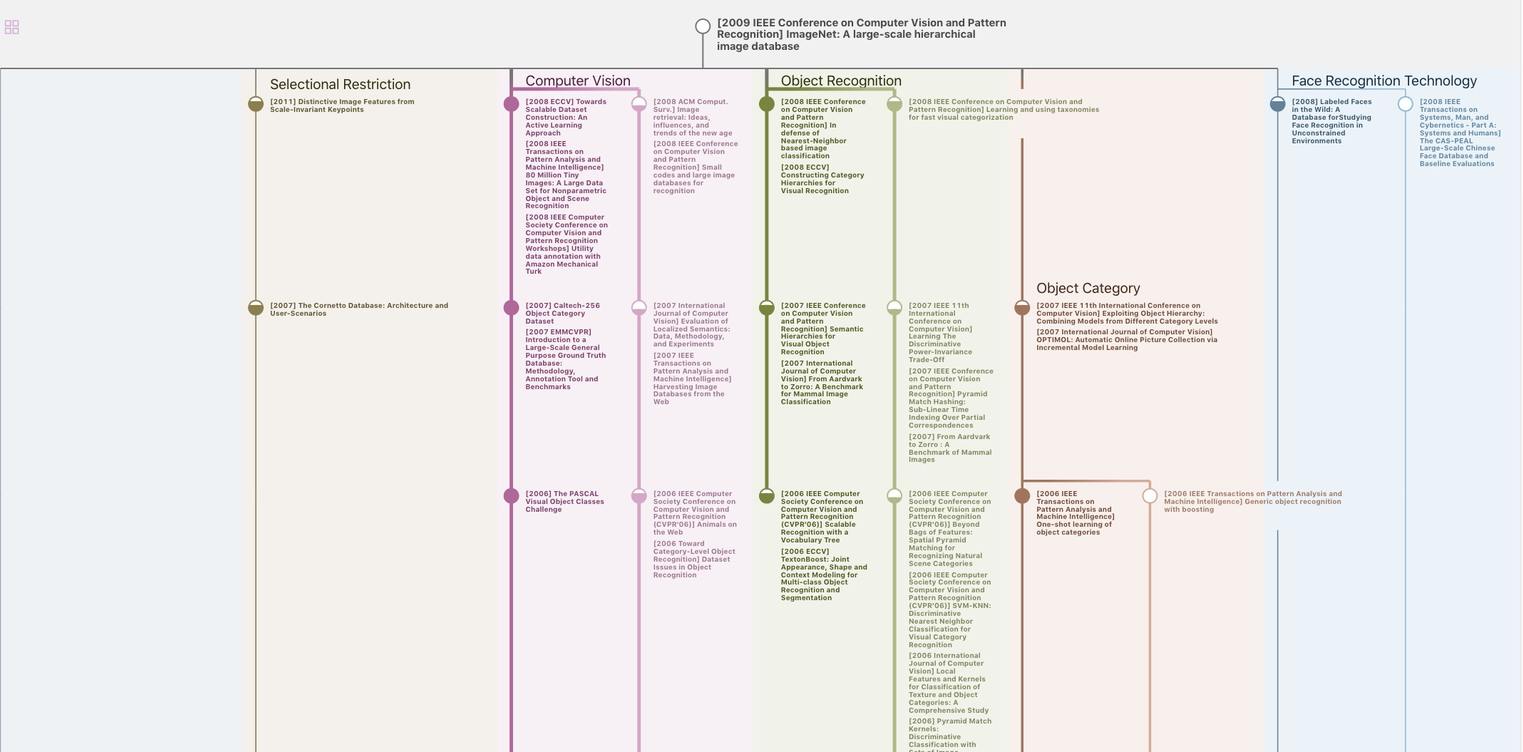
生成溯源树,研究论文发展脉络
Chat Paper
正在生成论文摘要