3D convolutional neural networks uncover modality-specific brain-imaging predictors for Alzheimer’s disease sub-scores
Brain Informatics(2024)
摘要
Different aspects of cognitive functions are affected in patients with Alzheimer’s disease. To date, little is known about the associations between features from brain-imaging and individual Alzheimer’s disease (AD)-related cognitive functional changes. In addition, how these associations differ among different imaging modalities is unclear. Here, we trained and investigated 3D convolutional neural network (CNN) models that predicted sub-scores of the 13-item Alzheimer’s Disease Assessment Scale–Cognitive Subscale (ADAS–Cog13) based on MRI and FDG–PET brain-imaging data. Analysis of the trained network showed that each key ADAS–Cog13 sub-score was associated with a specific set of brain features within an imaging modality. Furthermore, different association patterns were observed in MRI and FDG–PET modalities. According to MRI, cognitive sub-scores were typically associated with structural changes of subcortical regions, including amygdala, hippocampus, and putamen. Comparatively, according to FDG–PET, cognitive functions were typically associated with metabolic changes of cortical regions, including the cingulated gyrus, occipital cortex, middle front gyrus, precuneus cortex, and the cerebellum. These findings brought insights into complex AD etiology and emphasized the importance of investigating different brain-imaging modalities.
更多查看译文
关键词
Alzheimer's disease,Neural network,Brain imaging,Cognitive function
AI 理解论文
溯源树
样例
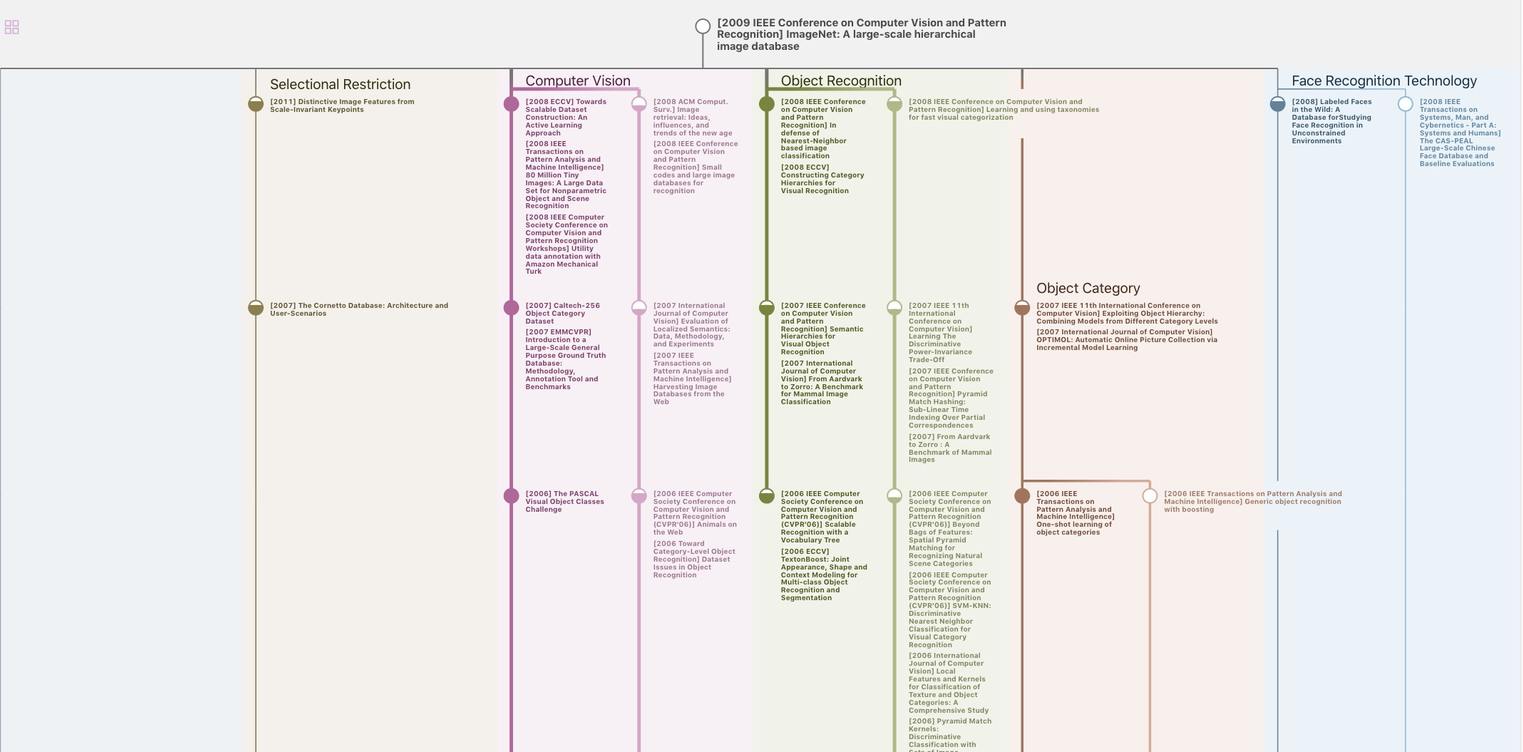
生成溯源树,研究论文发展脉络
Chat Paper
正在生成论文摘要