Monotone, Bi-Lipschitz, and Polyak-Lojasiewicz Networks
arxiv(2024)
摘要
This paper presents a new bi-Lipschitz invertible neural network, the
BiLipNet, which has the ability to control both its Lipschitzness
(output sensitivity to input perturbations) and inverse Lipschitzness
(input distinguishability from different outputs). The main contribution is a
novel invertible residual layer with certified strong monotonicity and
Lipschitzness, which we compose with orthogonal layers to build bi-Lipschitz
networks. The certification is based on incremental quadratic constraints,
which achieves much tighter bounds compared to spectral normalization.
Moreover, we formulate the model inverse calculation as a three-operator
splitting problem, for which fast algorithms are known. Based on the proposed
bi-Lipschitz network, we introduce a new scalar-output network, the PLNet,
which satisfies the Polyak-Łojasiewicz condition. It can be applied to learn
non-convex surrogate losses with favourable properties, e.g., a unique and
efficiently-computable global minimum.
更多查看译文
AI 理解论文
溯源树
样例
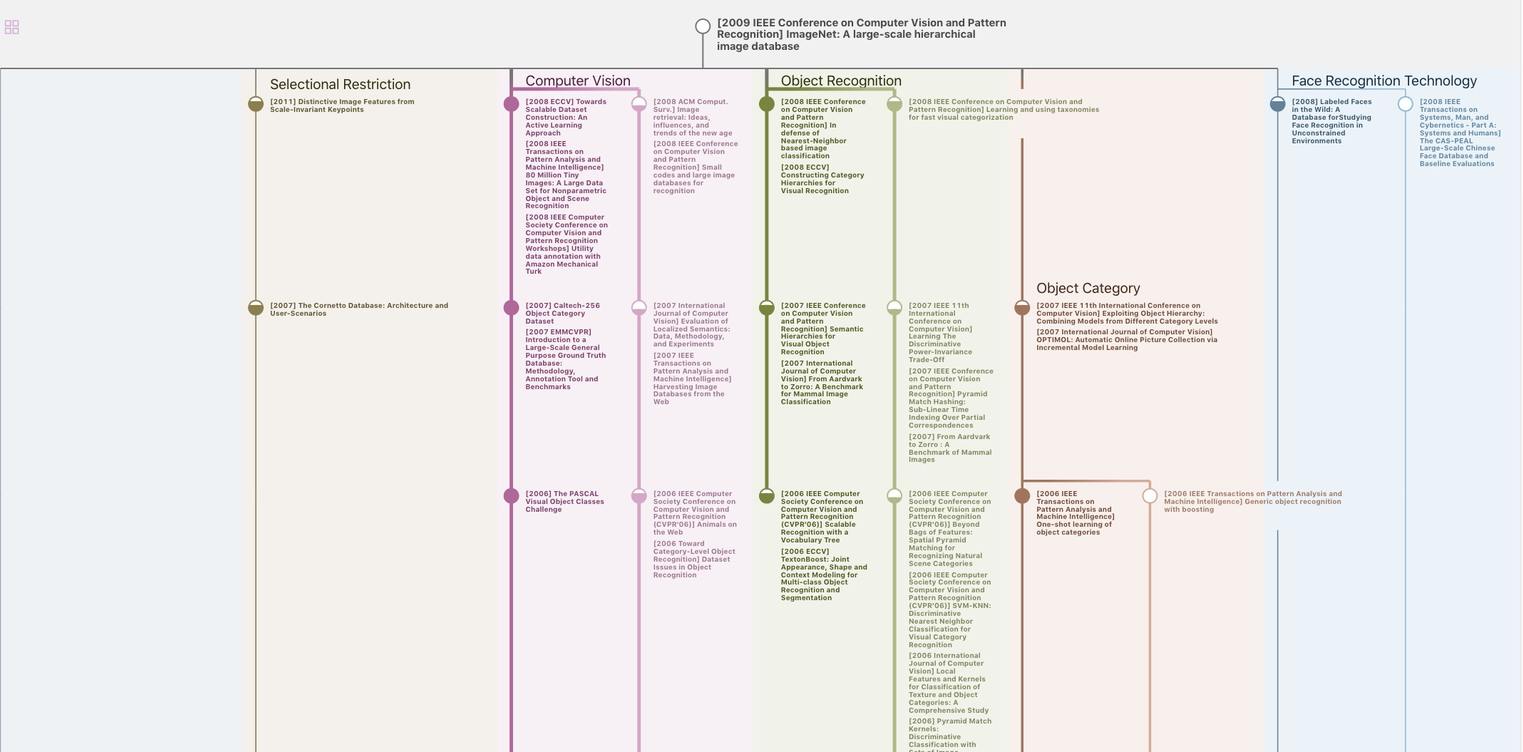
生成溯源树,研究论文发展脉络
Chat Paper
正在生成论文摘要