Towards Quantum-Safe Federated Learning via Homomorphic Encryption: Learning with Gradients
CoRR(2024)
摘要
This paper introduces a privacy-preserving distributed learning framework via
private-key homomorphic encryption. Thanks to the randomness of the
quantization of gradients, our learning with error (LWE) based encryption can
eliminate the error terms, thus avoiding the issue of error expansion in
conventional LWE-based homomorphic encryption. The proposed system allows a
large number of learning participants to engage in neural network-based deep
learning collaboratively over an honest-but-curious server, while ensuring the
cryptographic security of participants' uploaded gradients.
更多查看译文
AI 理解论文
溯源树
样例
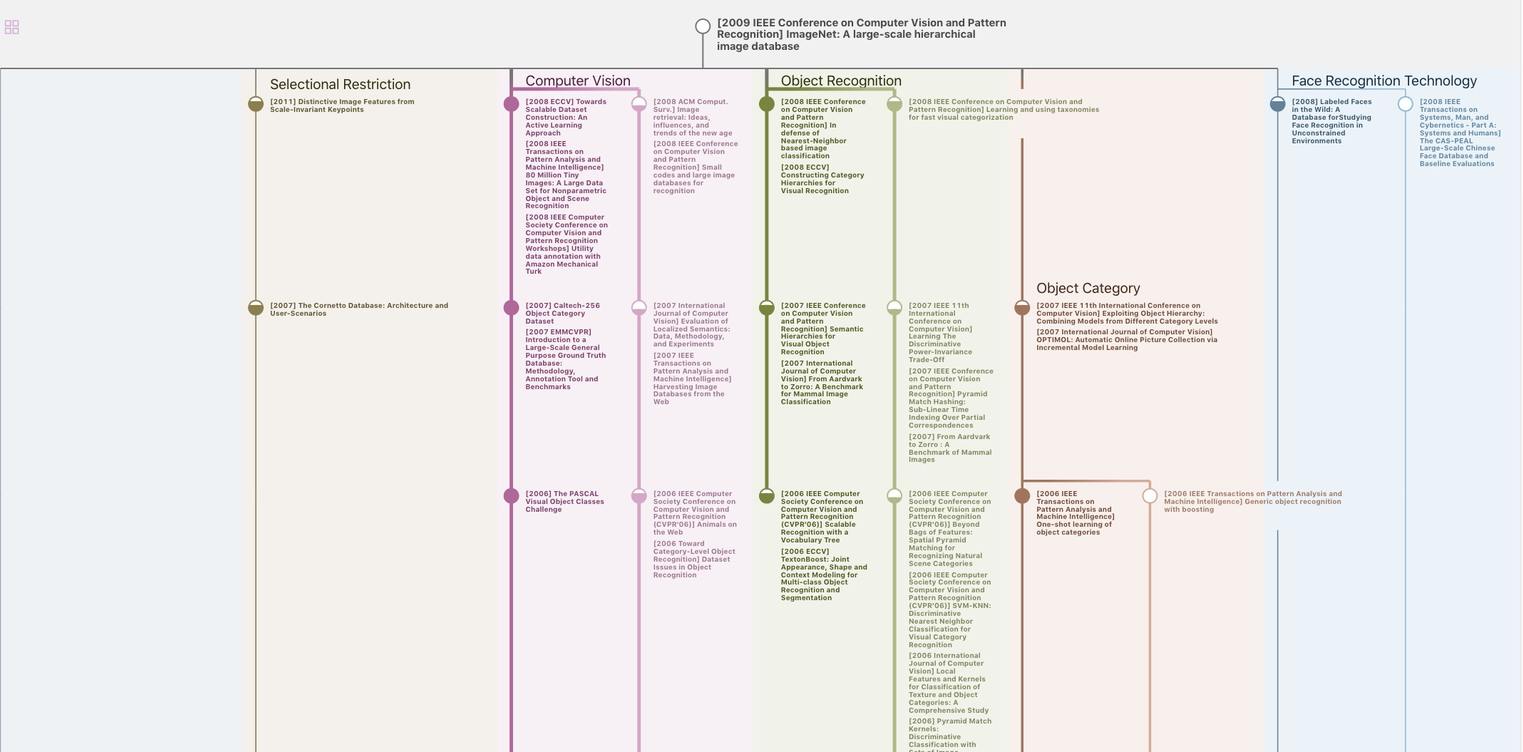
生成溯源树,研究论文发展脉络
Chat Paper
正在生成论文摘要