DRL-Based Dynamic Channel Access and SCLAR Maximization for Networks Under Jamming
arxiv(2024)
摘要
This paper investigates a deep reinforcement learning (DRL)-based approach
for managing channel access in wireless networks. Specifically, we consider a
scenario in which an intelligent user device (iUD) shares a time-varying uplink
wireless channel with several fixed transmission schedule user devices (fUDs)
and an unknown-schedule malicious jammer. The iUD aims to harmoniously coexist
with the fUDs, avoid the jammer, and adaptively learn an optimal channel access
strategy in the face of dynamic channel conditions, to maximize the network's
sum cross-layer achievable rate (SCLAR). Through extensive simulations, we
demonstrate that when we appropriately define the state space, action space,
and rewards within the DRL framework, the iUD can effectively coexist with
other UDs and optimize the network's SCLAR. We show that the proposed algorithm
outperforms the tabular Q-learning and a fully connected deep neural network
approach.
更多查看译文
AI 理解论文
溯源树
样例
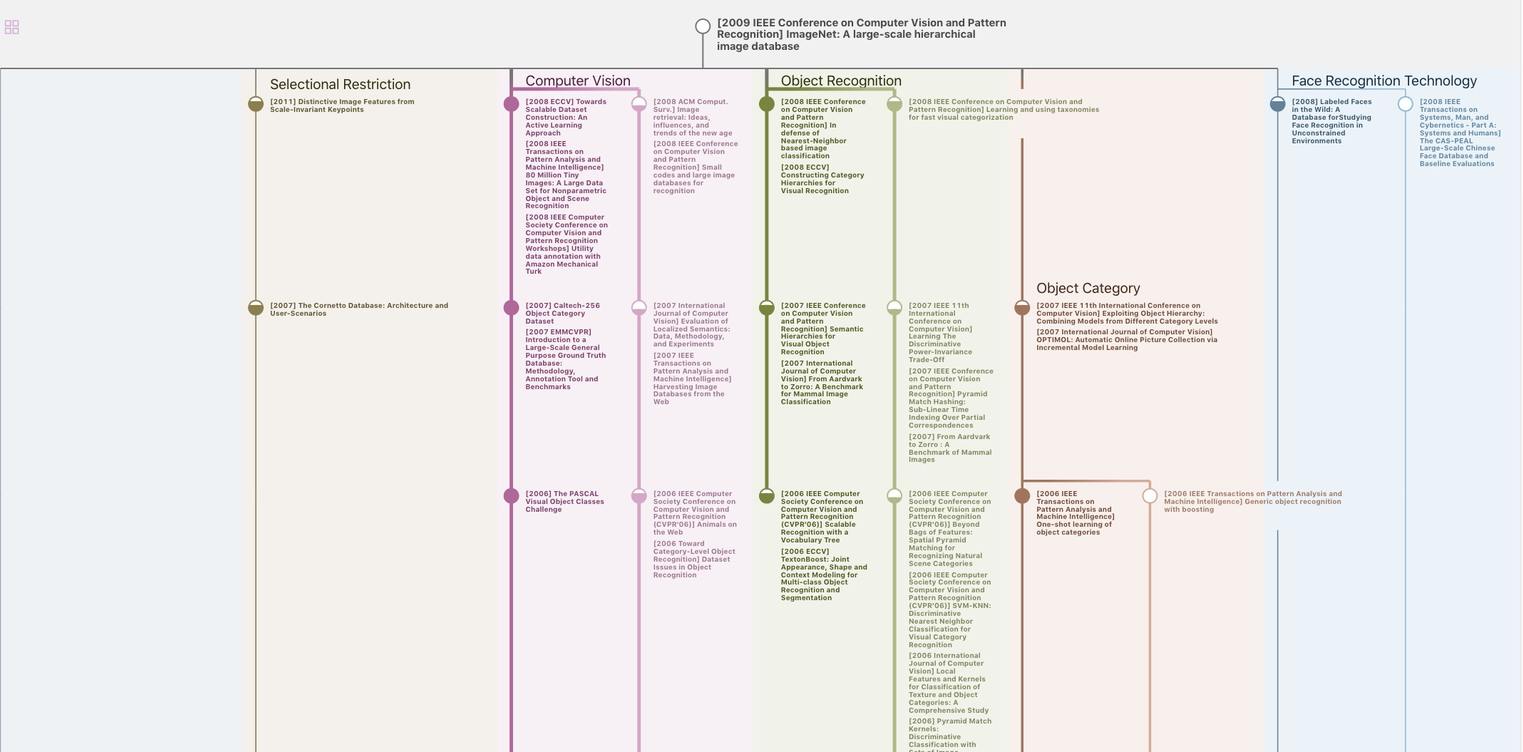
生成溯源树,研究论文发展脉络
Chat Paper
正在生成论文摘要