Unconditional Latent Diffusion Models Memorize Patient Imaging Data
CoRR(2024)
摘要
Generative latent diffusion models hold a wide range of applications in the
medical imaging domain. A noteworthy application is privacy-preserved open-data
sharing by proposing synthetic data as surrogates of real patient data. Despite
the promise, these models are susceptible to patient data memorization, where
models generate patient data copies instead of novel synthetic samples. This
undermines the whole purpose of preserving patient data and may even result in
patient re-identification. Considering the importance of the problem,
surprisingly it has received relatively little attention in the medical imaging
community. To this end, we assess memorization in latent diffusion models for
medical image synthesis. We train 2D and 3D latent diffusion models on CT, MR,
and X-ray datasets for synthetic data generation. Afterwards, we examine the
amount of training data memorized utilizing self-supervised models and further
investigate various factors that can possibly lead to memorization by training
models in different settings. We observe a surprisingly large amount of data
memorization among all datasets, with up to 41.7
training data memorized in CT, MRI, and X-ray datasets respectively. Further
analyses reveal that increasing training data size and using data augmentation
reduce memorization, while over-training enhances it. Overall, our results
suggest a call for memorization-informed evaluation of synthetic data prior to
open-data sharing.
更多查看译文
AI 理解论文
溯源树
样例
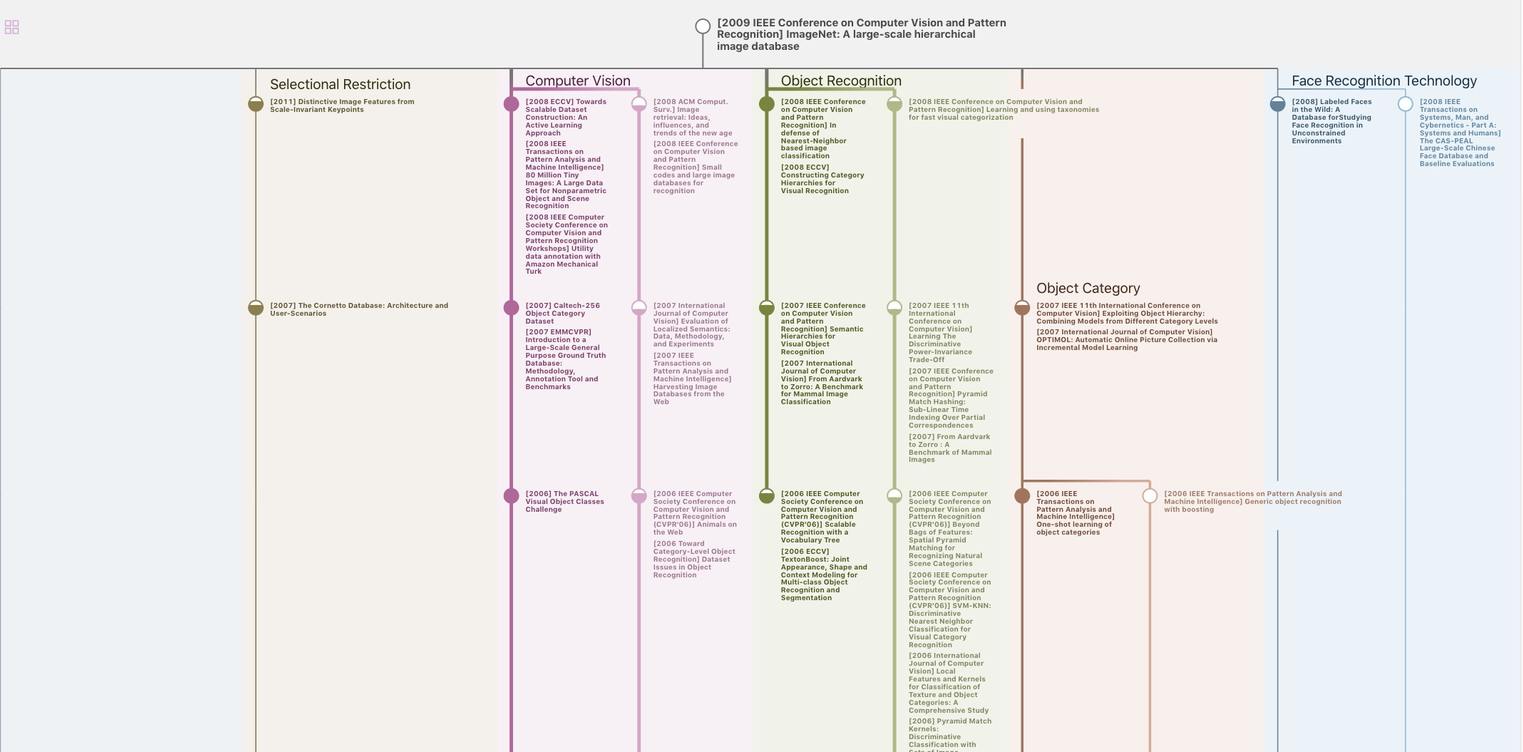
生成溯源树,研究论文发展脉络
Chat Paper
正在生成论文摘要