A framework for confounder considerations in AI-driven precision medicine
medrxiv(2024)
摘要
Introduction Artificial intelligence holds promise for individualized medicine. Yet, transitioning models from prototyping to clinical applications poses challenges, with confounders being a significant hurdle. We introduce a two-dimensional confounder framework ( Confound Continuum ), integrating a statistical dimension with a biomedical perspective. Informed and context-sensitive confounder decisions are indispensable for accurate model building, rigorous evaluation and valid interpretation.
Methods Using prediction of hand grip strength (HGS) from neuroimaging-derived features in a large sample as an example task, we develop a conceptual framework for confounder considerations and integrate it with an exemplary statistical investigation of 130 candidate confounders. We underline the necessity for conceptual considerations by predicting HGS with varying confound removal scenarios, neuroimaging derived features and machine learning algorithms. We use the confounders alone as features or together with grey matter volume to dissect the contribution of the two signal sources.
Results The conceptual confounder framework distinguishes between high-performance models and pure link models that aim to deepen our understanding of feature-target relationships. The biological attributes of different confounders can overlap to varying degrees with those of the predictive problem space, making the development of pure link models increasingly challenging with greater overlap. The degree of biological overlap allows to sort potential confounders on a conceptual Confound Continuum . This conceptual continuum complements statistical investigations with biomedical domain-knowledge, represented as an orthogonal two-dimensional grid.
Exemplary HGS predictions highlighted the substantial impact of confounders on predictive performance. In contrast, choice of features or learning algorithms had considerably smaller influences. Notably, models using confounders as features often outperformed models relying solely on neuroimaging features.
Conclusion Our study provides a confounder framework that combines a statistical perspective on confounders and a biomedical perspective. It stresses the importance of domain expertise in predictive modelling for critical and deliberate interpretation and employment of predictive models in biomedical applications and research.
Short description The paper explores the challenges of transitioning predictive models from scientific prototyping to clinical use, with a focus on the significant impact of confounders. Using the example of predicting hand grip strength in the UK Biobank, the study introduces a framework that integrates statistical and biomedical perspectives on confounders, emphasizing the vital role of informed confounder decisions for accurate model development, evaluation and interpretation.
### Competing Interest Statement
The authors have declared no competing interest.
### Funding Statement
This research was funded by the Deutsche Forschungsgemeinschaft (DFG, German Research Foundation), Project-ID 431549029, Collaborative Research Centre CRC1451 on motor performance, project B05.
### Author Declarations
I confirm all relevant ethical guidelines have been followed, and any necessary IRB and/or ethics committee approvals have been obtained.
Yes
The details of the IRB/oversight body that provided approval or exemption for the research described are given below:
The used human data of this study was retrieved from the The UK Biobank. The UK Biobank has approval from the North West Multi-centre Research Ethics Committee (MREC) as a Research Tissue Bank (RTB) (https://www.ukbiobank.ac.uk/learn-more-about-uk-biobank/about-us/ethics) and is allowed to obtain and disseminate data and samples from the participants. The UK Biobank ethical regulations cover the work in this manuscript. All participants had given written informed consent.
I confirm that all necessary patient/participant consent has been obtained and the appropriate institutional forms have been archived, and that any patient/participant/sample identifiers included were not known to anyone (e.g., hospital staff, patients or participants themselves) outside the research group so cannot be used to identify individuals.
Yes
I understand that all clinical trials and any other prospective interventional studies must be registered with an ICMJE-approved registry, such as ClinicalTrials.gov. I confirm that any such study reported in the manuscript has been registered and the trial registration ID is provided (note: if posting a prospective study registered retrospectively, please provide a statement in the trial ID field explaining why the study was not registered in advance).
Yes
I have followed all appropriate research reporting guidelines, such as any relevant EQUATOR Network research reporting checklist(s) and other pertinent material, if applicable.
Yes
All individual data used in this study were obtained from the UK Biobank, a major biomedical database (www.ukbiobank.ac.uk), and are available to all approved UK Biobank researchers.
更多查看译文
AI 理解论文
溯源树
样例
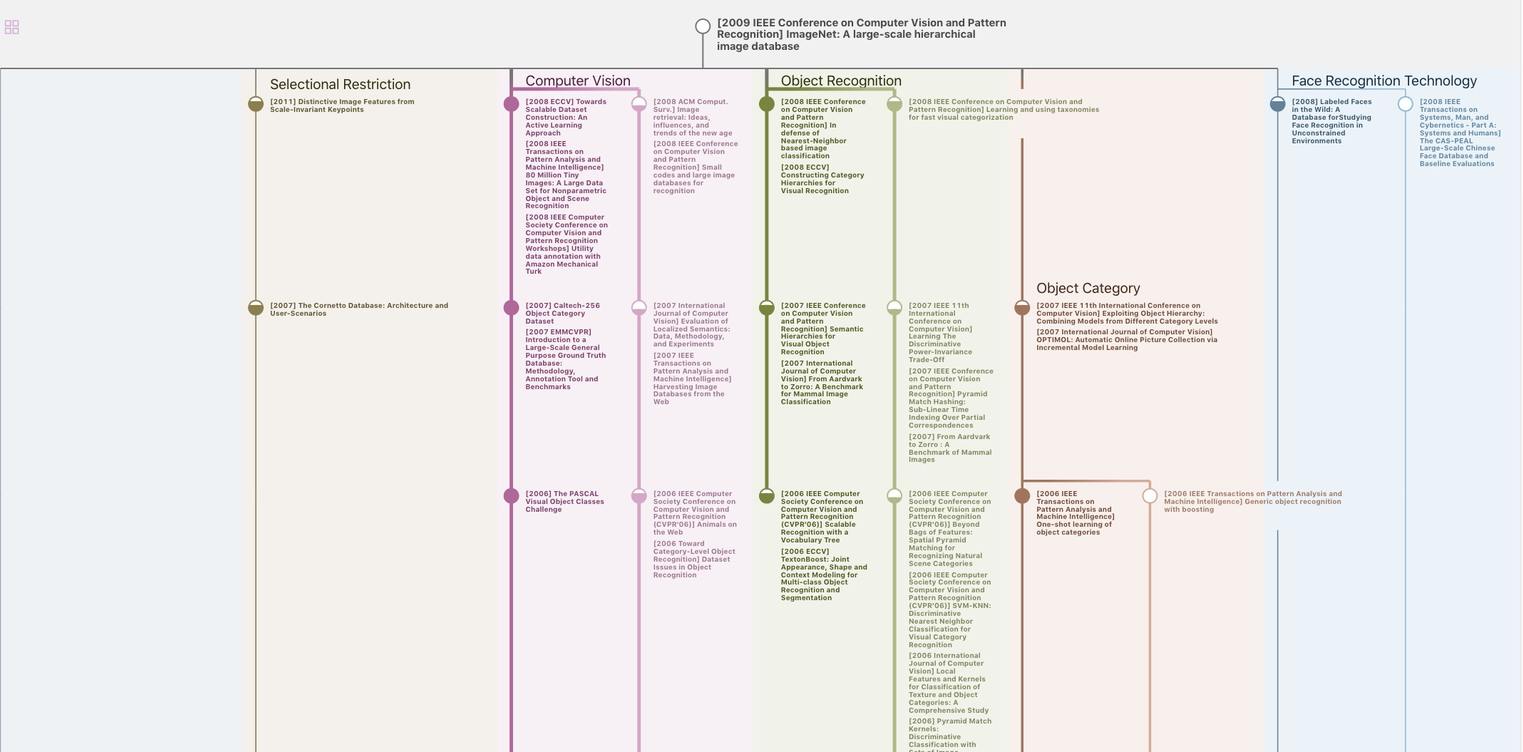
生成溯源树,研究论文发展脉络
Chat Paper
正在生成论文摘要