Unfolding 3D Space into Binary Images for Daylight Simulation via Neural Network
Journal of Daylighting(2023)
摘要
Daylighting plays a crucial role in building science, impacting both occupants’ well-being and energy consumption in buildings. Balancing the size of openings with energy efficiency has long been a challenge. To address this, various daylight metrics have been developed to assess interior spaces’ daylight quality. Additionally, architects have been using simulation algorithms to predict postconstruction light conditions. In recent years, machine learning (ML) has revolutionized daylight simulations, offering a way to predict daylight conditions without cumbersome 3D modeling or heavy computational resources. However, accommodating architects’ creativity remains a challenge for current machine learning-based models. Specifically, the diversity of window shapes and their locations on facades poses difficulties for prediction accuracy. To overcome this limitation, this paper proposes a novel method that transforms wall information into matrices and uses them as input to train an artificial neural network-based model; this model can well predict the annual daylight simulation result generated by the Climate-Based Daylight Modeling tools. This method allows the model to adapt to various real-world design scenarios in real time, and its robust reliability has been demonstrated through evaluations of prediction accuracy concerning different annual daylight metrics. This approach caters to specific cases and opens possibilities for application in other machine learning and deep learning-based methods.
更多查看译文
关键词
daylight simulation,neural network,daylight metrics,machine learning
AI 理解论文
溯源树
样例
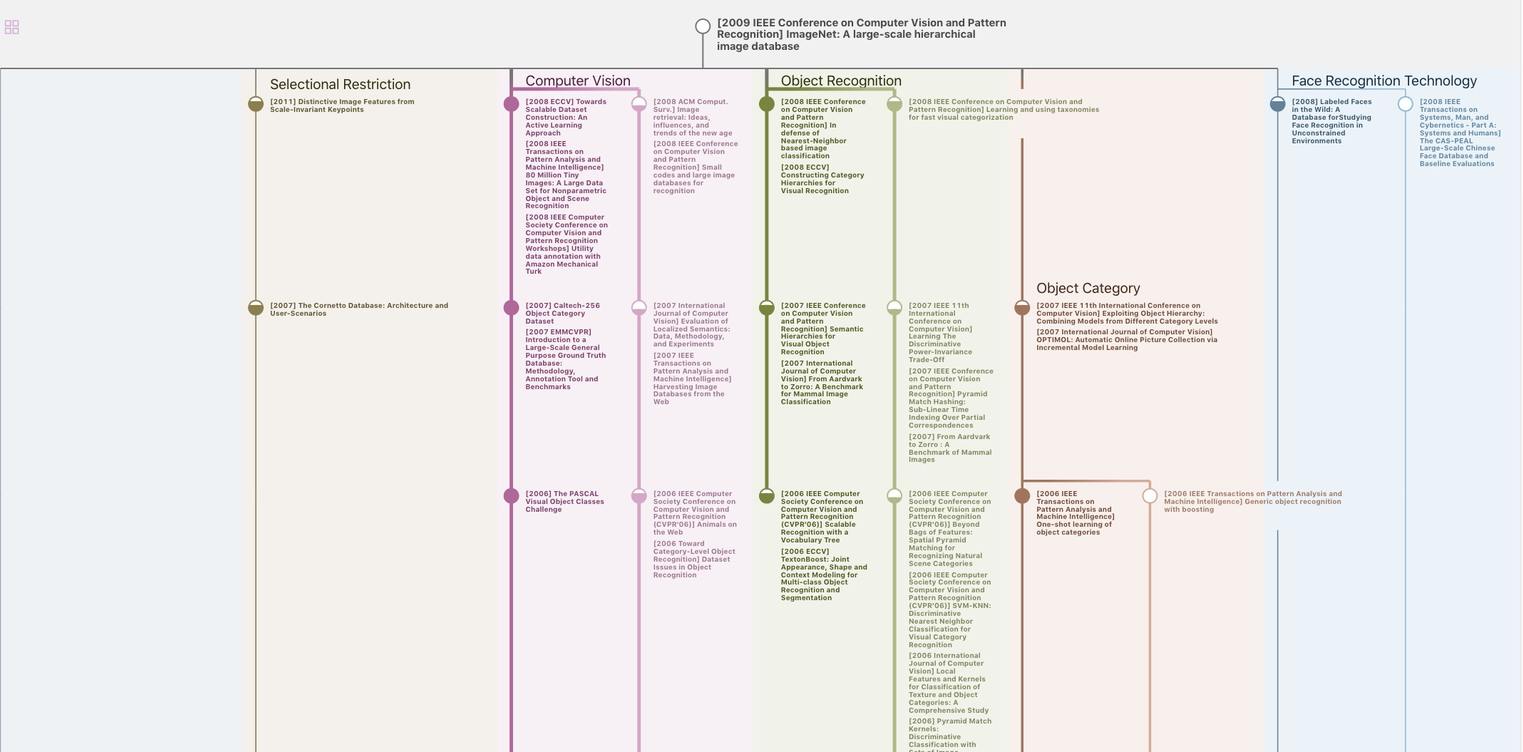
生成溯源树,研究论文发展脉络
Chat Paper
正在生成论文摘要