Effectiveness of CT radiomic features combined with clinical factors in predicting prognosis in patients with limited-stage small cell lung cancer
BMC Cancer(2024)
摘要
Background The prognosis of SCLC is poor and difficult to predict. The aim of this study was to explore whether a model based on radiomics and clinical features could predict the prognosis of patients with limited-stage small cell lung cancer (LS-SCLC). Methods Simulated positioning CT images and clinical features were retrospectively collected from 200 patients with histological diagnosis of LS-SCLC admitted between 2013 and 2021, which were randomly divided into the training ( n = 140) and testing ( n = 60) groups. Radiomics features were extracted from simulated positioning CT images, and the t-test and the least absolute shrinkage and selection operator (LASSO) were used to screen radiomics features. We then constructed radiomic score (RadScore) based on the filtered radiomics features. Clinical factors were analyzed using the Kaplan–Meier method. The Cox proportional hazards model was used for further analyses of possible prognostic features and clinical factors to build three models including a radiomic model, a clinical model, and a combined model including clinical factors and RadScore. When a model has prognostic predictive value (AUC > 0.7) in both train and test groups, a nomogram will be created. The performance of three models was evaluated using area under the receiver operating characteristic curve (AUC) and Kaplan–Meier analysis. Results A total of 1037 features were extracted from simulated positioning CT images which were contrast enhanced CT of the chest. The combined model showed the best prediction, with very poor AUC for the radiomic model and the clinical model. The combined model of OS included 4 clinical features and RadScore, with AUCs of 0.71 and 0.70 in the training and test groups. The combined model of PFS included 4 clinical features and RadScore, with AUCs of 0.72 and 0.71 in the training and test groups. T stages, ProGRP and smoke status were the independent variables for OS in the combined model, whereas T stages, ProGRP and prophylactic cranial irradiation (PCI) were the independent factors for PFS. There was a statistically significant difference between the low- and high-risk groups in the combined model of OS (training group, p < 0.0001; testing group, p = 0.0269) and PFS (training group, p < 0.0001; testing group, p < 0.0001). Conclusion Combined models involved RadScore and clinical factors can predict prognosis in LS-SCLC and show better performance than individual radiomics and clinical models.
更多查看译文
关键词
Small cell lung cancer,Radiomics,Prognosis,Texture analysis,Simulated positioning CT
AI 理解论文
溯源树
样例
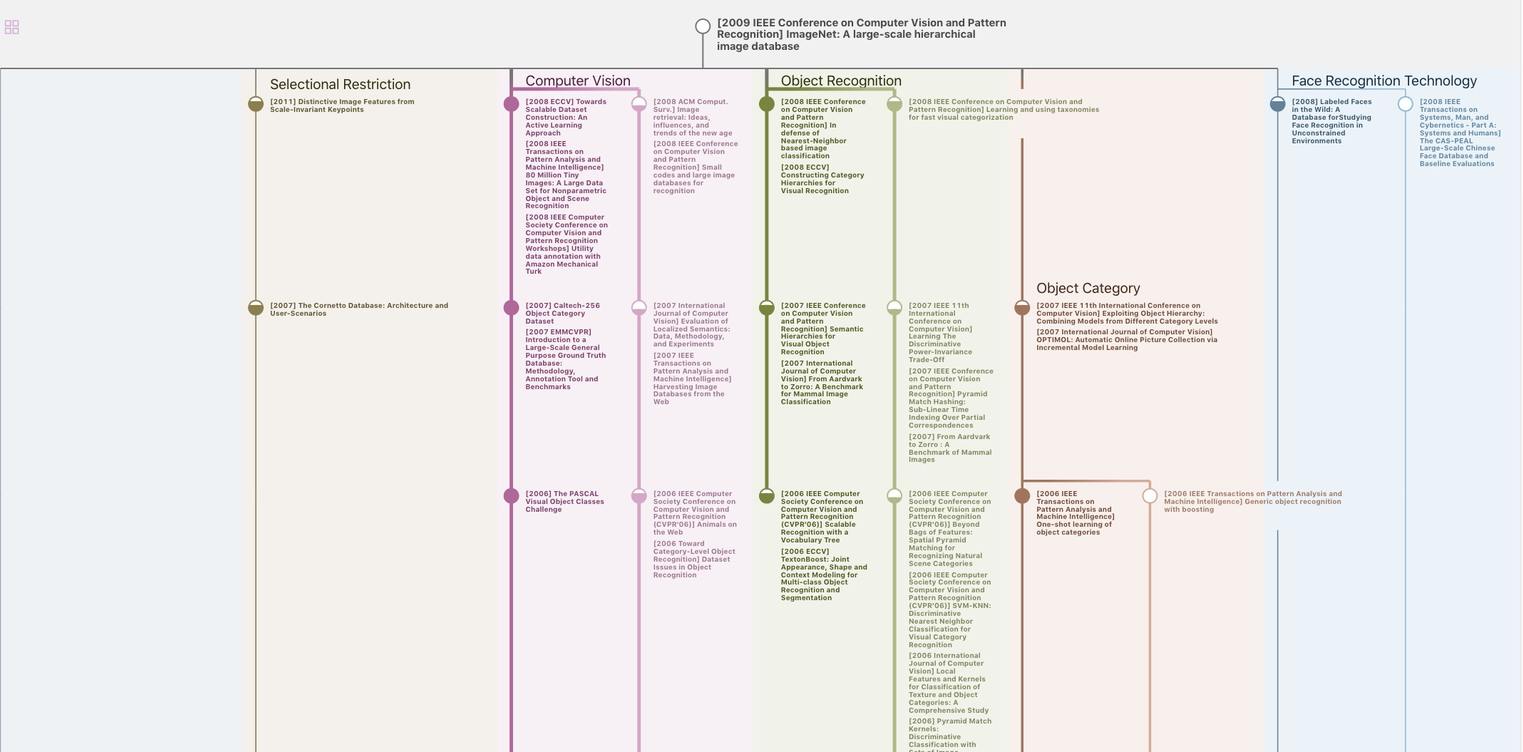
生成溯源树,研究论文发展脉络
Chat Paper
正在生成论文摘要