A Lifetime Prediction Approach Based on Adversarial Variational Auto-Encoder Networks for Dry-Type Power Transformers
2023 IEEE International Conference on Energy Technologies for Future Grids (ETFG)(2023)
摘要
Remaining useful lifetime prediction provides effective means for reasonable lifetime extension and safe functioning of power transformers and supports stable operation and maintenance for power grids. To address the problems of data limitation and massive capital and labor consumptions in traditional prediction methods, a novel power transformer life prediction approach based on Adversarial Variational Auto-encoder(AVAE) is proposed in this paper. Feature extraction and data enhancement are performed by a variational auto-encoder. Adversarial training network is implemented to obtain data- driven in global optimum conditions. Furthermore, Long Short-Term Memory(LSTM) network is utilized to efficiently extract the long-term dependencies in the transformer time series data, which in turn achieves the aging rate prediction. Real data of power transformers are employed for the experiments. The experimental results show that the prediction algorithm based on AVAE-LSTM networks possesses higher accuracy compares to the conventional methods.
更多查看译文
关键词
power transformer,aging rate,lifetime prediction,AVAE
AI 理解论文
溯源树
样例
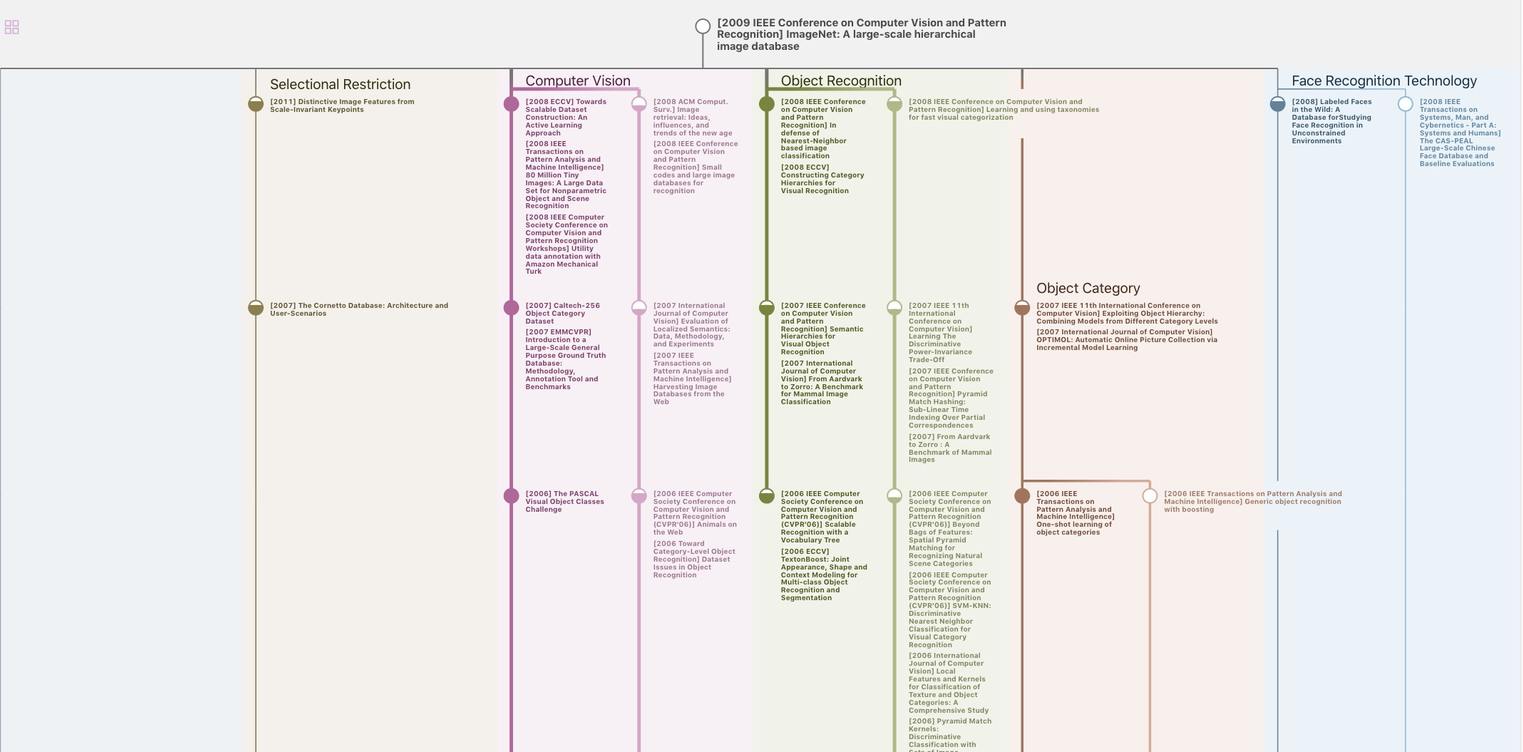
生成溯源树,研究论文发展脉络
Chat Paper
正在生成论文摘要