A Data Augmentation-Based Household Profile Identification Approach Under Semi-supervised and Federated Learning Framework
2023 IEEE International Conference on Energy Technologies for Future Grids (ETFG)(2023)
摘要
Millions of residential households have adopted smart meters, which provide valuable insights for power companies and retailers. Detailed smart meter data allows these entities to extract essential information, such as consumers' household profiles, including social demographics. This information, in turn, enables retailers to offer a diverse range of value-added services. It's important to note that smart meter data is typically controlled by various retailers. Considering the protection of user privacy and retailer interests, retailers tend to use their own data to train identification models, which leads to the problem of low identification accuracy due to insufficient training data. In addition, most existing identification models use supervised learning, but it is difficult to obtain label samples. Therefore, to address the issue of insufficient data samples mentioned above, this paper presents a data augmentation-based semi-supervised and federated learning framework. By adopting the federated learning framework, retailers can learn the features of other retailers' data without sharing smart meter data, and in a disguised way expand the number of training samples, which helps to improve the identification accuracy and protect user privacy. Experiments show that the proposed Federated Semi-supervised Back propagation (FL-SSBP) model has the best performance among the benchmarks.
更多查看译文
关键词
Federated Learning,household profile,smart meter data,machine learning
AI 理解论文
溯源树
样例
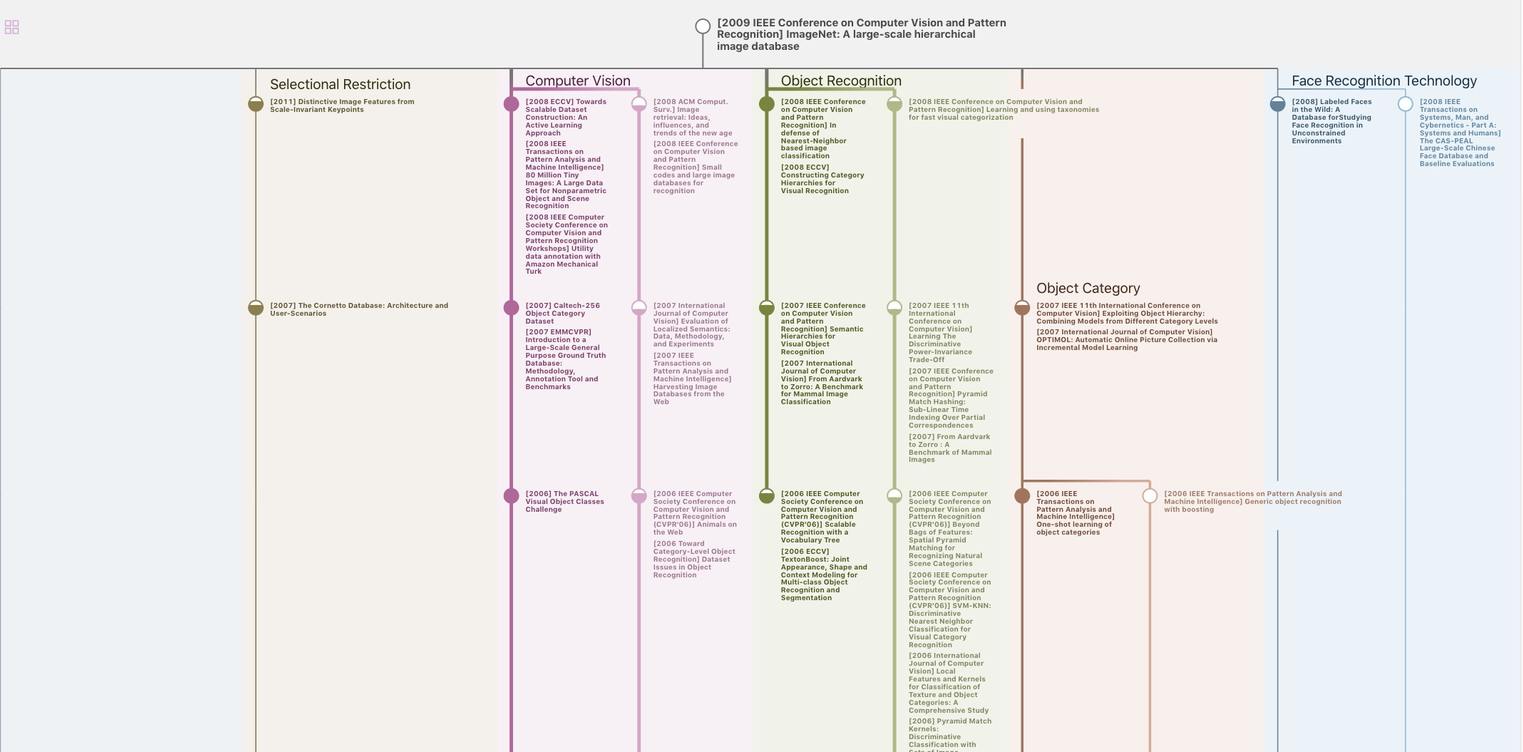
生成溯源树,研究论文发展脉络
Chat Paper
正在生成论文摘要