Fault Detection, Classification, and Localization in ADNs with Optimally Placed Micro-PMUs
2023 IEEE International Conference on Energy Technologies for Future Grids (ETFG)(2023)
摘要
It is increasingly challenging to accurately identify, classify, and localize faults in active distribution networks (ADNs). By accurately identifying faults, distribution system operators (DSOs) will be able to provide reliable power to customers while reducing downtime and minimizing their maintenance costs. In this paper, a holistic fault detection, classification, and coordinated localization framework are presented using optimally placed micro-phasor measurement units (µPMUs) with voltage sag characteristics. This research introduces a coordinated fault strategy by first determining the approximate fault location using machine learning (ML) techniques, then using the closest µPMUs to determine the exact fault location. Fault detection and classification are accomplished through a simple feed-forward neural network, whereas an ensemble bagged trees classifier is utilized for fault localization. Moreover, with the proposed method, fault impedance, and ground resistance can also be correlated. The effectiveness of the method is verified in IEEE-13 and IEEE-34 node test feeders, including the effects of various µPMU placement methods from previous literature.
更多查看译文
关键词
Micro-PMU,Distribution Network,Optimal Placement,Fault Analysis,Fault Localization
AI 理解论文
溯源树
样例
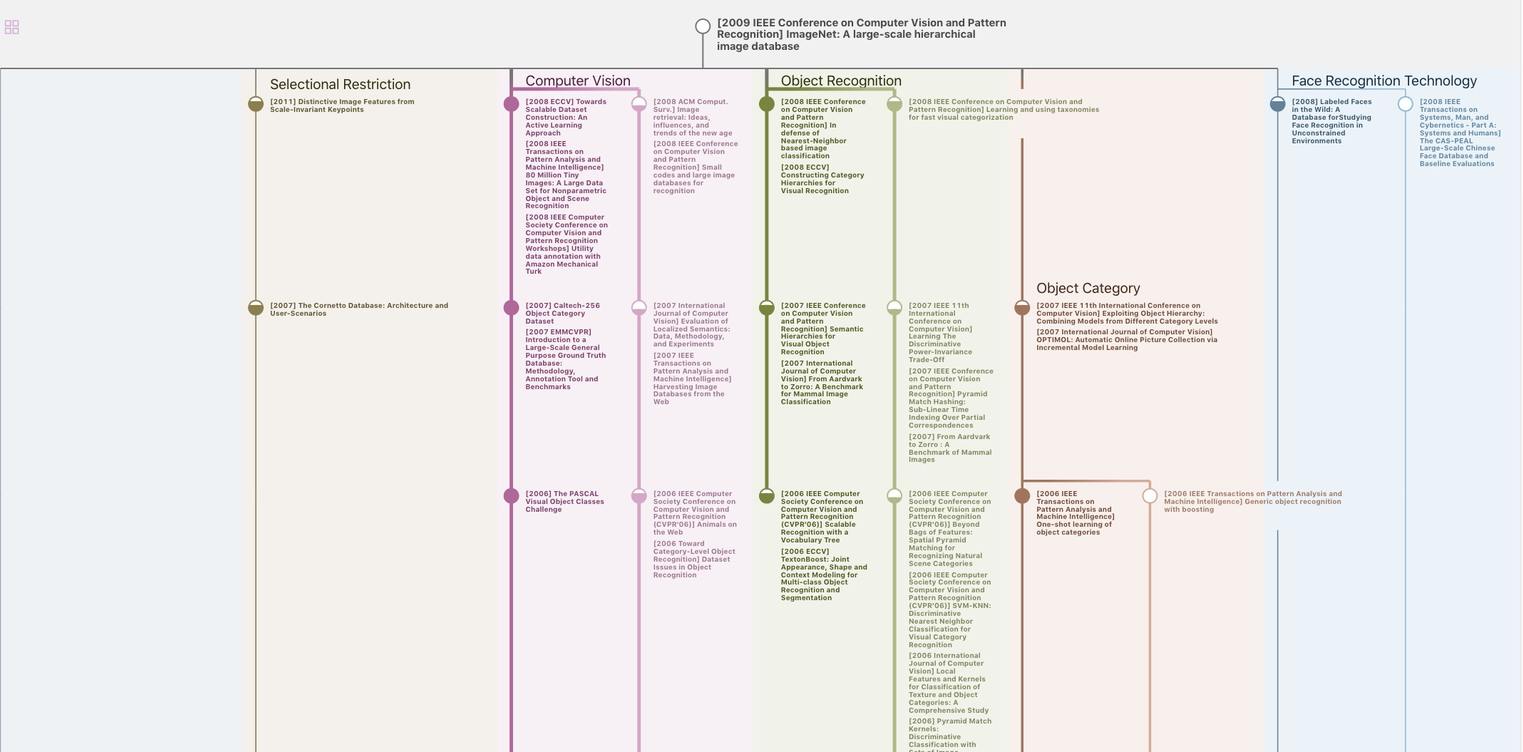
生成溯源树,研究论文发展脉络
Chat Paper
正在生成论文摘要