Model-driven Learning for Cell-Free MIMO Beamforming with Compression Strategy.
2023 International Conference on Wireless Communications and Signal Processing (WCSP)(2023)
摘要
Cell-free Multiple-Input Multiple-Output (MIMO) system is an appealing solution to improve the performance of wireless communication systems. However, the system performance improvement is restricted by the capacity limitations of fronthaul links. To address this issue, a compress-and-forward scheme is introduced, and the joint optimization of precoding and fronthaul compression is considered. Existing algorithms for this joint optimization often involve high computational complexity and latency due to iterative processes, making them unsuitable for real-time implementation. In this paper, we propose a model-driven neural network-based deep learning method that leverages expert knowledge, i.e., the uplink-downlink duality, to characterize the structure of beamforming matrix using low-dimensional parameters. These parameters, referred to as "key features", are learned from the channel state information through a neural network. Simulation results show that the proposed method achieves performance close to the optimal solution while significantly reducing computational time.
更多查看译文
关键词
Deep learning,cell-free MIMO,precoding,fronthaul compression,uplink-downlink duality
AI 理解论文
溯源树
样例
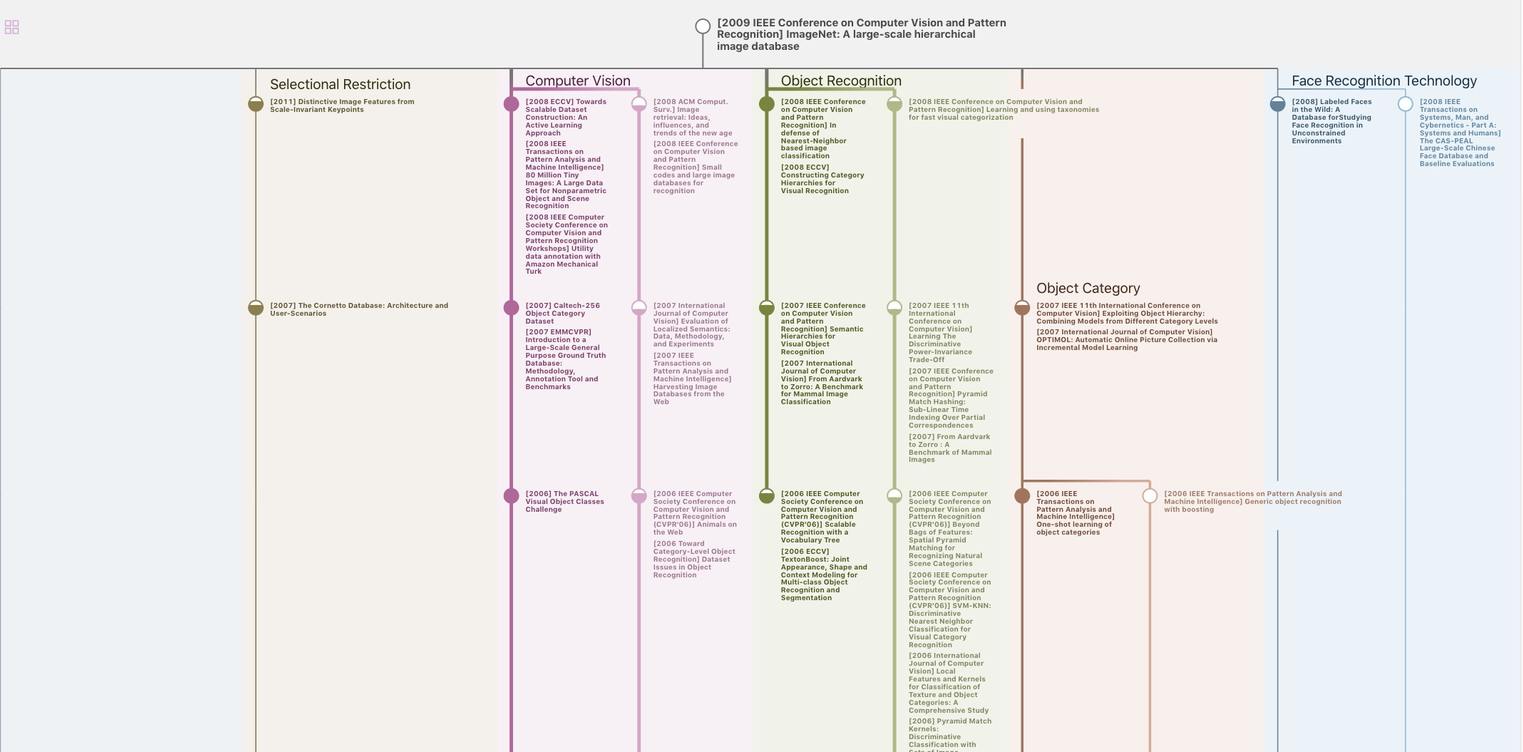
生成溯源树,研究论文发展脉络
Chat Paper
正在生成论文摘要