A machine learning strategy for detection of stator winding short-circuit faults in induction motors
2023 IEEE International Autumn Meeting on Power, Electronics and Computing (ROPEC)(2023)
摘要
Currently, induction motor (IM) is the most common electric machine in the industry. IMs are robust machines; however, they are prone to suffer failures, often located in stator winding due to external factors such as electrical or mechanical overstress, thermal changes, and environmental conditions, which affect the proper functioning of the IM. Therefore, implementing a monitoring system serves the purpose of timely detecting stator failures and determining their severity in order to evaluate the physical state of the winding. In this work, a machine learning strategy is proposed for the analysis and recognition of various degrees of severity of short-circuit damages in the winding of an IM. In general, this strategy consists of statistical indicators, the one-way analysis of variance (ANOVA) method, and a support vector machine (SVM) classifier. Results demonstrate the effectiveness of the proposal.
更多查看译文
关键词
ANOVA,shot-circuit damage,machine learning,induction motor,statistical indicators,support vector machine
AI 理解论文
溯源树
样例
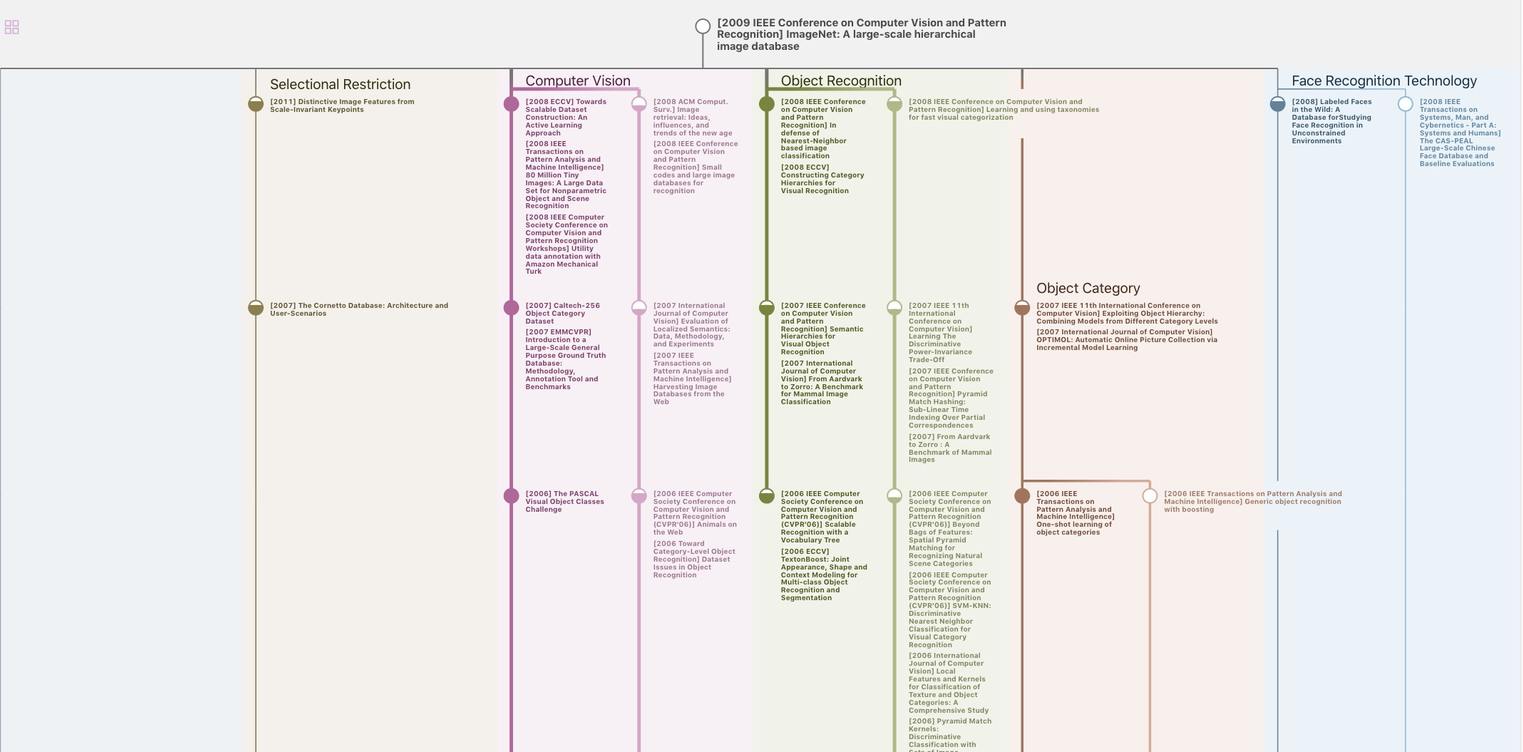
生成溯源树,研究论文发展脉络
Chat Paper
正在生成论文摘要