A lightweight bladder tumor segmentation method based on attention mechanism
Medical & Biological Engineering & Computing(2024)
摘要
In the endoscopic images of bladder, accurate segmentation of different grade bladder tumor from blurred boundary regions and highly variable shapes is of great significance for doctors’ diagnosis and patients’ later treatment. We propose a nested attentional feature fusion segmentation network (NAFF-Net) based on the encoder-decoder structure formed by the combination of weighted pyramid pooling module (WPPM) and nested attentional feature fusion (NAFF). Among them, WPPM applies the cascade of atrous convolution to enhance the overall perceptual field while introducing adaptive weights to optimize multi-scale feature extraction, NAFF integrates deep semantic information into shallow feature maps, effectively focusing on edge and detail information in bladder tumor images. Additionally, a weighted mixed loss function is constructed to alleviate the impact of imbalance between positive and negative sample distribution on segmentation accuracy. Experiments illustrate the proposed NAFF-Net achieves better segmentation results compared to other mainstream models, with a MIoU of 84.05%, MPrecision of 91.52%, MRecall of 90.81%, and F1-score of 91.16%, and also achieves good results on the public datasets Kvasir-SEG and CVC-ClinicDB. Compared to other models, NAFF-Net has a smaller number of parameters, which is a significant advantage in model deployment. Graphical abstract
更多查看译文
关键词
Bladder tumor segmentation,Weighted pyramid pooling module,Attentional feature fusion,Weighted mixed loss function
AI 理解论文
溯源树
样例
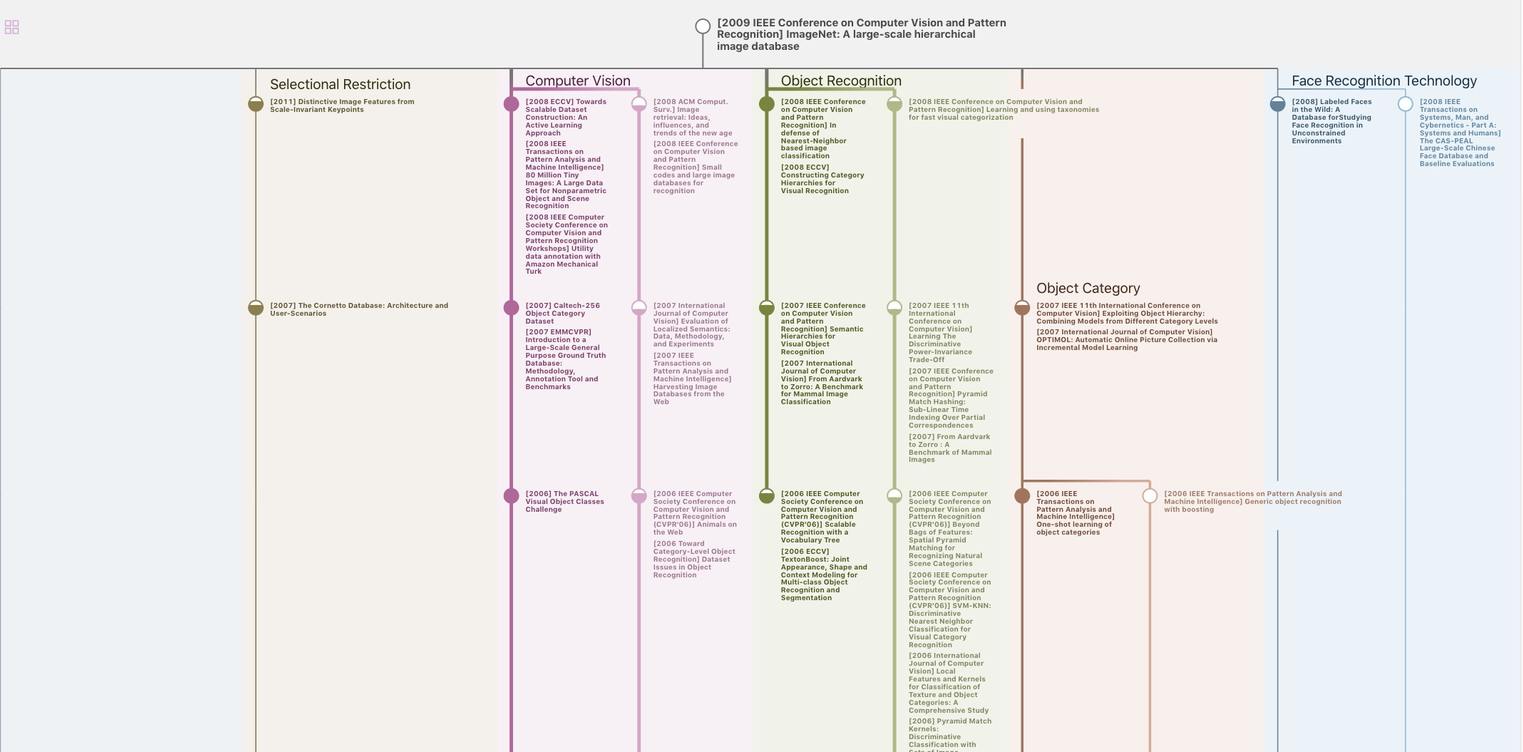
生成溯源树,研究论文发展脉络
Chat Paper
正在生成论文摘要