A machine learning based model for student’s dropout prediction in online training
Education and Information Technologies(2024)
摘要
School dropout is a significant issue in distance learning, and early detection is crucial for addressing the problem. Our study aims to create a binary classification model that anticipates students’ activity levels based on their current achievements and engagement on a Canadian Distance learning Platform. Predicting student dropout, a common classification problem in educational data analysis, is addressed by utilizing a comprehensive dataset that includes 49 features ranging from socio-demographic to behavioral data. This dataset provides a unique opportunity to analyze student interactions and success factors in a distance learning environment. We have developed a student profiling system and implemented a predictive approach using XGBoost, selecting the most important features for the prediction process. In this work, our methodology was developed in Python, using the widely used sci-kit-learn package. Alongside XGBoost, logistic regression was also employed as part of our combination of strategies to enhance the models predictive capabilities. Our work can accurately predict student dropout, achieving an accuracy rate of approximately 82% on unseen data from the next academic year.
更多查看译文
关键词
Dropout school,Machine learning,Prediction,Sociodemographic data,Bihavioral data
AI 理解论文
溯源树
样例
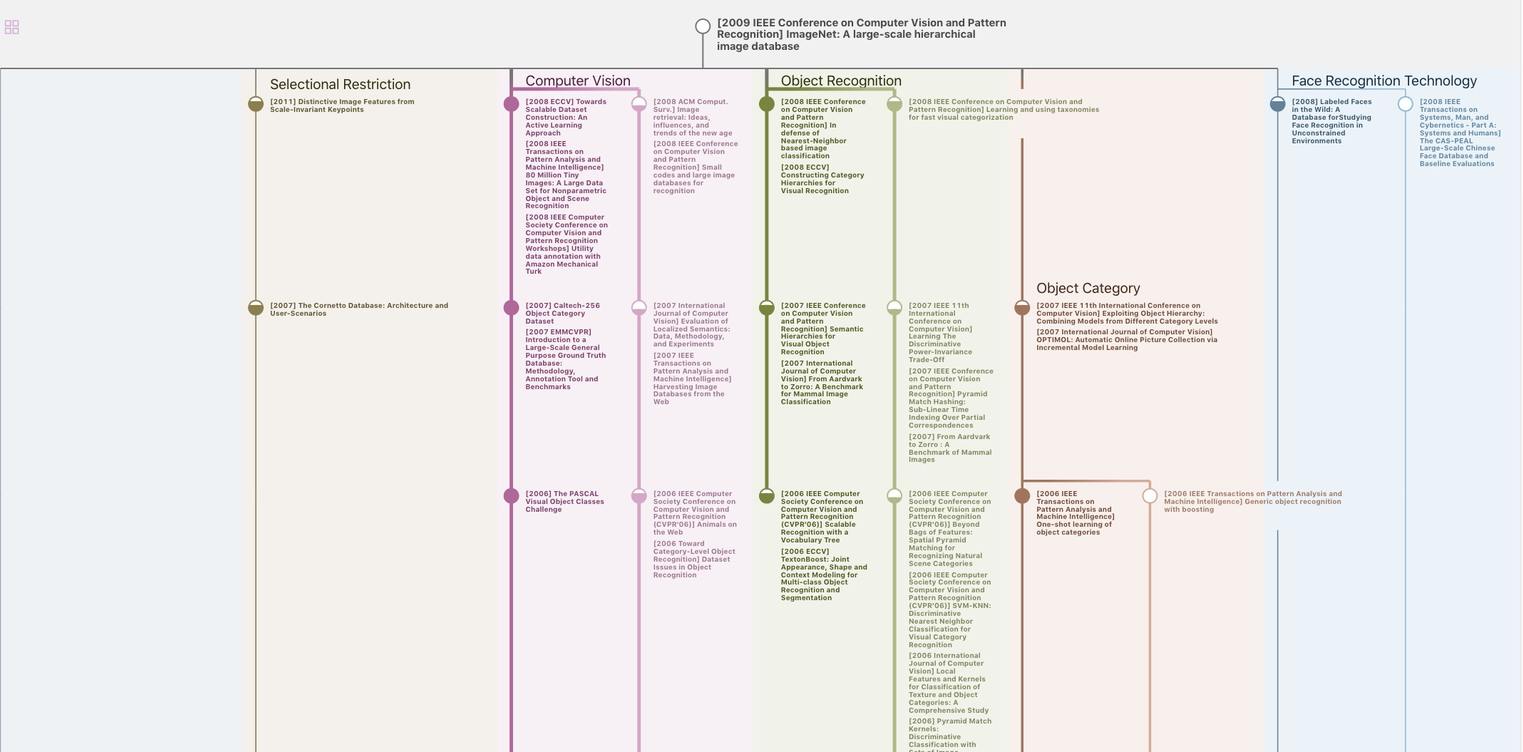
生成溯源树,研究论文发展脉络
Chat Paper
正在生成论文摘要