T2LR-Net: An unrolling network learning transformed tensor low-rank prior for dynamic MR image reconstruction
COMPUTERS IN BIOLOGY AND MEDICINE(2024)
摘要
The tensor low -rank prior has attracted considerable attention in dynamic MR reconstruction. Tensor lowrank methods preserve the inherent high -dimensional structure of data, allowing for improved extraction and utilization of intrinsic low -rank characteristics. However, most current methods are still confined to utilizing low -rank structures either in the image domain or predefined transformed domains. Designing an optimal transformation adaptable to dynamic MRI reconstruction through manual efforts is inherently challenging. In this paper, we propose a deep unrolling network that utilizes the convolutional neural network (CNN) to adaptively learn the transformed domain for leveraging tensor low -rank priors. Under the supervised mechanism, the learning of the tensor low -rank domain is directly guided by the reconstruction accuracy. Specifically, we generalize the traditional t-SVD to a transformed version based on arbitrary high -dimensional unitary transformations and introduce a novel unitary transformed tensor nuclear norm (UTNN). Subsequently, we present a dynamic MRI reconstruction model based on UTNN and devise an efficient iterative optimization algorithm using ADMM, which is finally unfolded into the proposed T2LR-Net. Experiments on two dynamic cardiac MRI datasets demonstrate that T2LR-Net outperforms the state-of-the-art optimization -based and unrolling network -based methods.
更多查看译文
关键词
Dynamic MR imaging,Transformed tensor low-rank prior,Unrolling network,t-SVD,Tensor nuclear norm
AI 理解论文
溯源树
样例
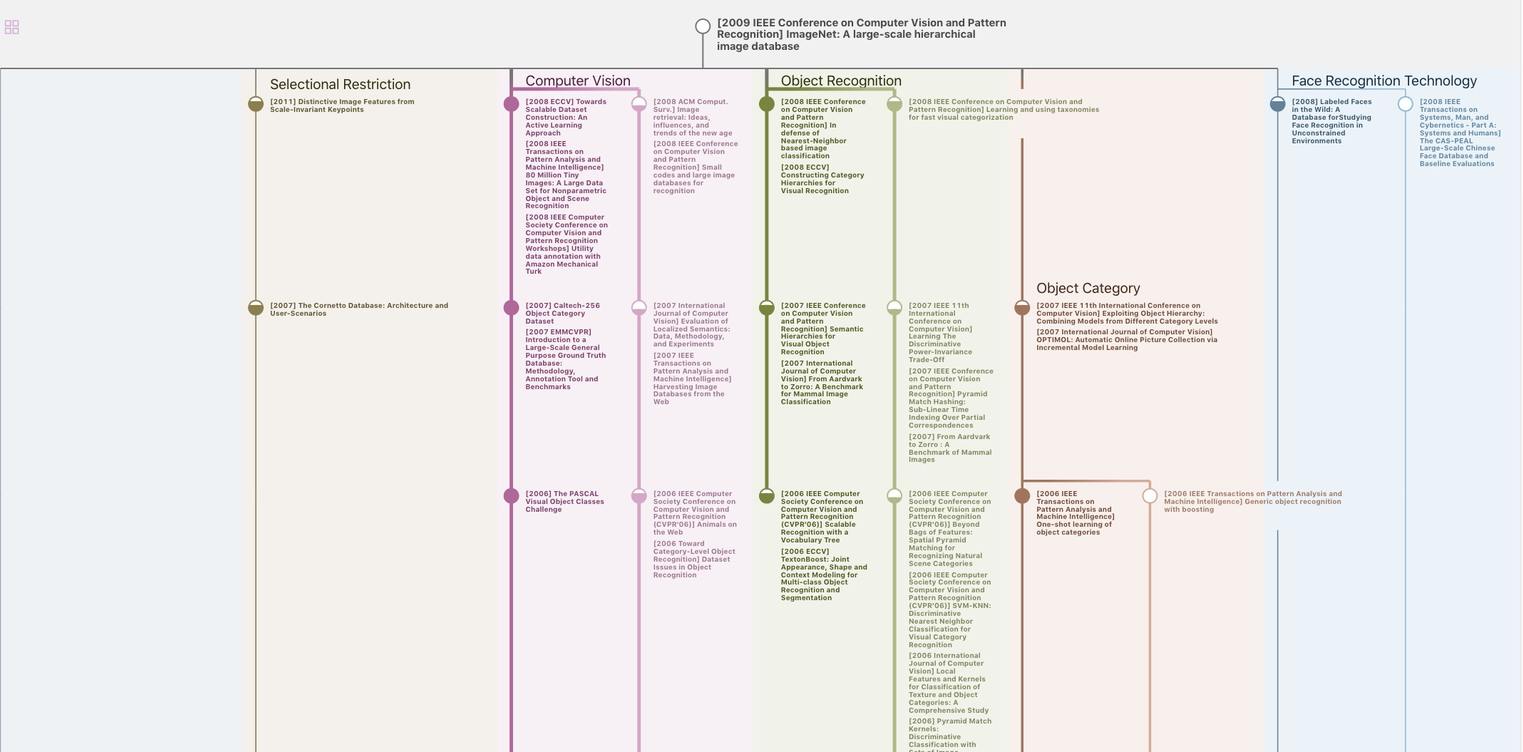
生成溯源树,研究论文发展脉络
Chat Paper
正在生成论文摘要