Vision-based Analytics of Flare Stacks Using Deep Learning Detection
2023 21ST INTERNATIONAL CONFERENCE ON ADVANCED ROBOTICS, ICAR(2023)
摘要
Flare stacks play a critical role in oil refineries and chemical plants, but monitoring their performance is a challenging task that often requires skilled operators. To address this challenge, we propose a novel approach that combines video capturing and machine learning techniques to automate the monitoring of flare stack operations in realtime. Our vision-based system analyzes captured video footage of the flare stack's scene and employs state-of-the-art deep learning detection models, including YOLOv5, YOLOv7, and the Detection Transformer (DETR), to detect and analyze combustion-related objects such as flame and smoke. Rigorous experiments show that the proposed technique was able to accurately detect flame and smoke objects in flare stacks scene and the best model showed encouraging performance metrics. By leveraging the power of recent deep detection models, our proposed system offers a promising alternative to labor-intensive manual inspection by keeping a continuous and automated watchable eye in combustion quality, facilitating more efficient and reliable flare stack operation analysis.
更多查看译文
关键词
Deep Learning,Deep Learning For Detection,Gas Flaring,Deep Models,Performance Metrics,Detection Model,Role In Plants,Video Capture,Oil Refinery,Drinking Water,Convolutional Neural Network,Superior Performance,Water Vapor,Oil And Gas,Object Detection,Intersection Over Union,Bounding Box,Precision Values,Feed-forward Network,Objective Presentation,Optimal Assignment,Frames Per Second,Vision Sensors,Mean Average Precision,Inference Time,Value Of Map,Object Detection Model,You Only Look Once,Inference Speed,Model Performance
AI 理解论文
溯源树
样例
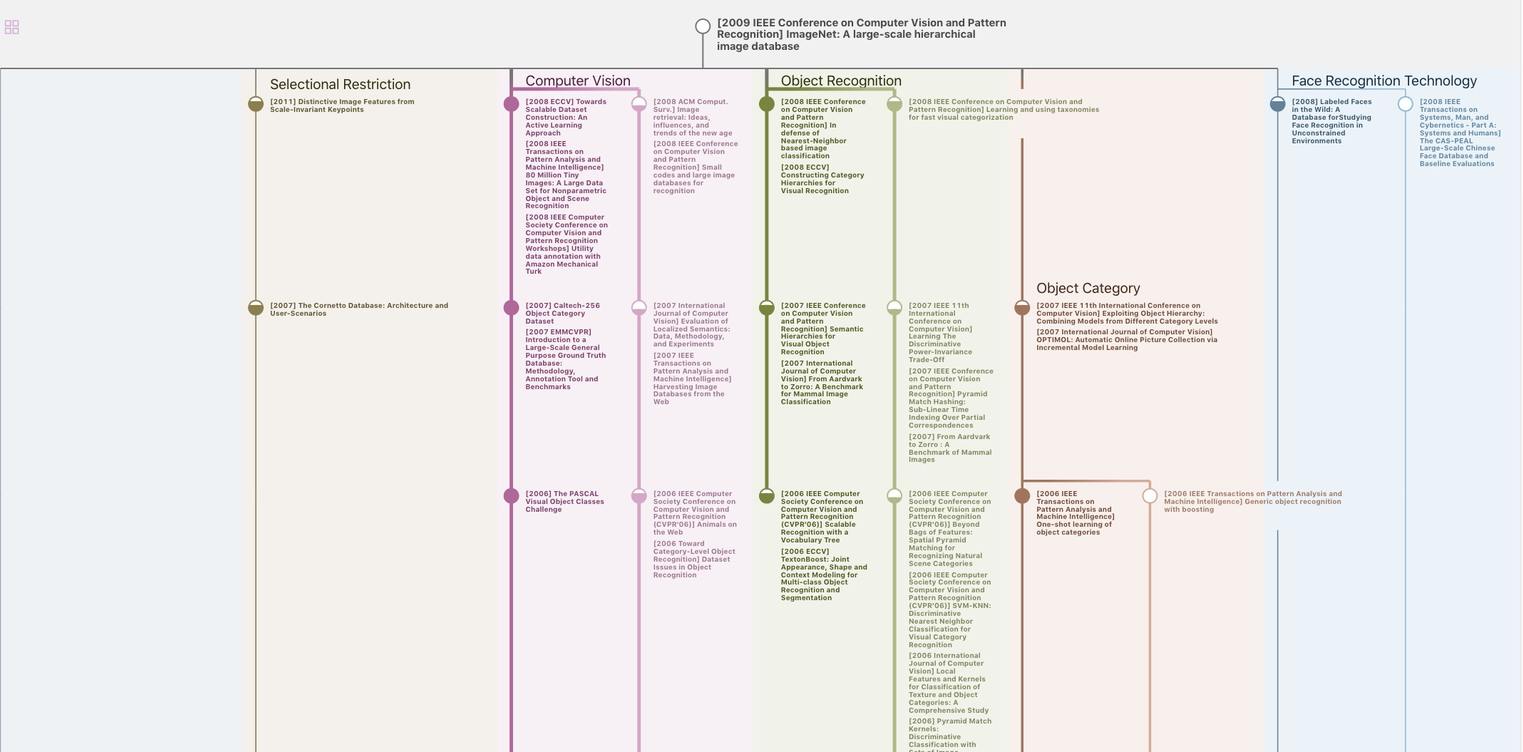
生成溯源树,研究论文发展脉络
Chat Paper
正在生成论文摘要