On Learning of Inverse Kinematics for Highly Redundant Robots with Neural Networks
2023 21ST INTERNATIONAL CONFERENCE ON ADVANCED ROBOTICS, ICAR(2023)
摘要
The inverse kinematic problem for redundant robots is still difficult to solve. One approach is learning the inverse kinematic model with artificial neural networks, while the key challenge is the ambiguity of solutions. Due to the redundancy in the robot's degrees of freedom, there are multiple or even unlimited valid joint states bringing the end effector to a desired position. We show to what extent this problem influences the achievable accuracy of supervised training approaches depending on the number of degrees of freedom. To overcome the difficulties, a new training scheme is proposed, which uses the analytically solvable forward kinematics model. The new unsupervised training method uses random sampling in the joint state space and is not dependent on ambiguous tuples of joint values and end effector poses. We analyze the effect of the sample density on the remaining position error and show that additional soft constraints can easily be integrated in the training scheme, which offers the possibility to consider obstacle avoidance directly in the inverse kinematic model. Evaluations have been done using different robot models with up to 20 degrees of freedom, while not only position, but also the end effector's orientation at the goal point is considered.
更多查看译文
关键词
Neural Network,Inverse Kinematics,Redundant Robot,Degrees Of Freedom,Ambiguity,Random Sampling,Artificial Neural Network,Sampling Density,Training Methods,Additional Constraints,Unsupervised Methods,Sample Space,Position Error,Forward Model,End-effector,Obstacle Avoidance,Joint State,Robot Model,Unsupervised Training,End-effector Pose,Cartesian Space,Artificial Model,Multilayer Perceptron,Joint Angles,Target Pose,Kinematic Chain,Multiple Solutions,Static Scenes,Real Robot,Joint Space
AI 理解论文
溯源树
样例
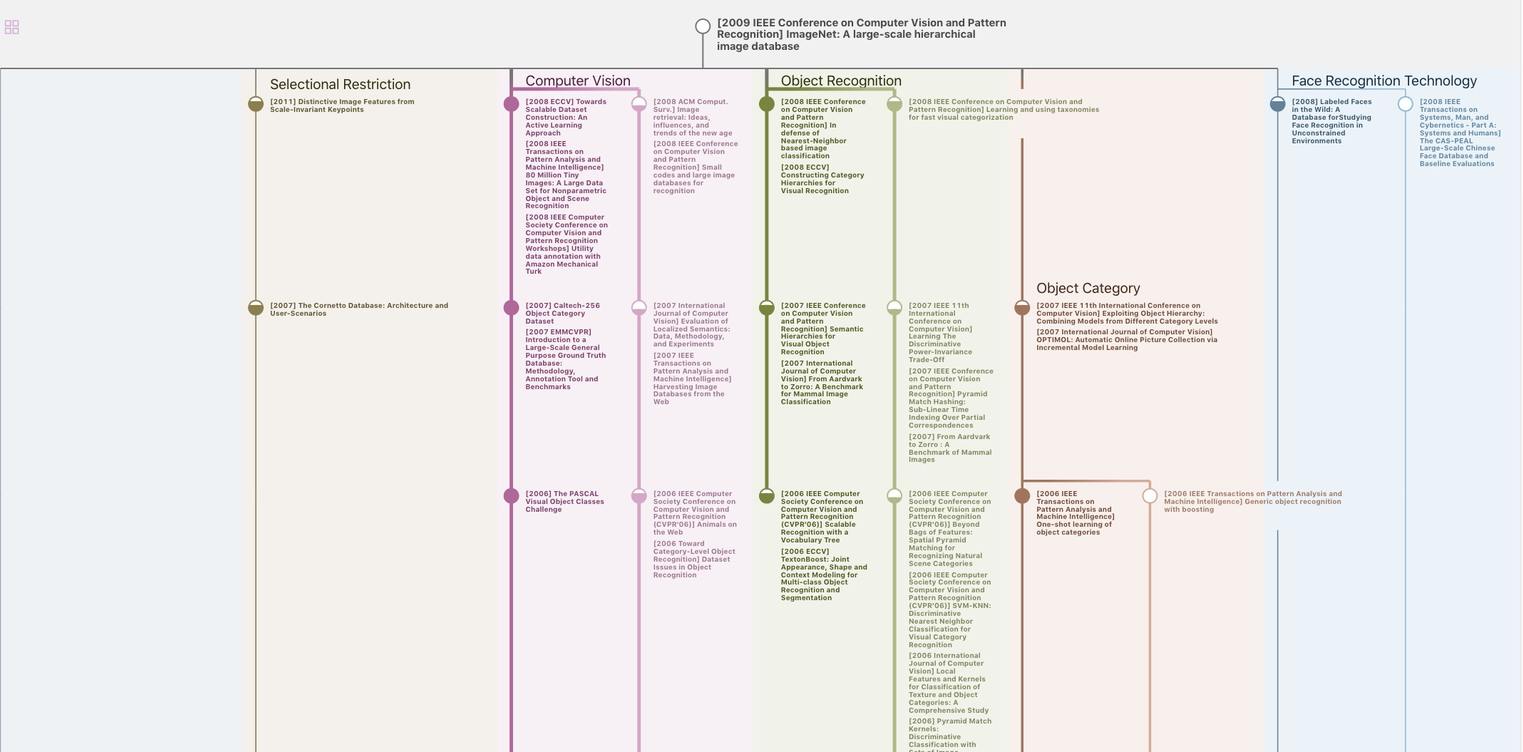
生成溯源树,研究论文发展脉络
Chat Paper
正在生成论文摘要