Scalable and Efficient Hierarchical Visual Topological Mapping
2023 21ST INTERNATIONAL CONFERENCE ON ADVANCED ROBOTICS, ICAR(2023)
摘要
Hierarchical topological representations can significantly reduce search times within mapping and localization algorithms. Although recent research has shown the potential for such approaches, limited consideration has been given to the suitability and comparative performance of different global feature representations within this context. In this work, we evaluate state-of-the-art hand-crafted and learned global descriptors using a hierarchical topological mapping technique on benchmark datasets and present results of a comprehensive evaluation of the impact of the global descriptor used. Although learned descriptors have been incorporated into place recognition methods to improve retrieval accuracy and enhance overall recall, the problem of scalability and efficiency when applied to longer trajectories has not been adequately addressed in a majority of research studies. Based on our empirical analysis of multiple runs, we identify that continuity and distinctiveness are crucial characteristics for an optimal global descriptor that enable efficient and scalable hierarchical mapping, and present a methodology for quantifying and contrasting these characteristics across different global descriptors. Our study demonstrates that the use of global descriptors based on an unsupervised learned Variational Autoencoder (VAE) excels in these characteristics and achieves significantly lower runtime. It runs on a consumer grade desktop, up to 2.3x faster than the second best global descriptor, NetVLAD, and up to 9.5x faster than the hand-crafted descriptor, PHOG, on the longest track evaluated (St Lucia, 17.6 km), without sacrificing overall recall performance.
更多查看译文
关键词
Scalable,Mapping Efficiency,Hierarchical Map,Topological Map,Empirical Analysis,Feature Representation,Benchmark Datasets,Variational Autoencoder,Limited Consideration,Hierarchical Representation,Global Descriptors,Place Recognition,Longer Trajectories,Neural Network,Convolutional Neural Network,Number Of Images,Semantic Information,Exhaustive Search,Total Run Time,Image Representation,Loop Closure,Increase In Recall,Large Number Of Loci,Content-based Image Retrieval,Bag Of Visual Words,Image Descriptors,Longer Running Time,Smooth Changes,Image Retrieval,Ground Truth Location
AI 理解论文
溯源树
样例
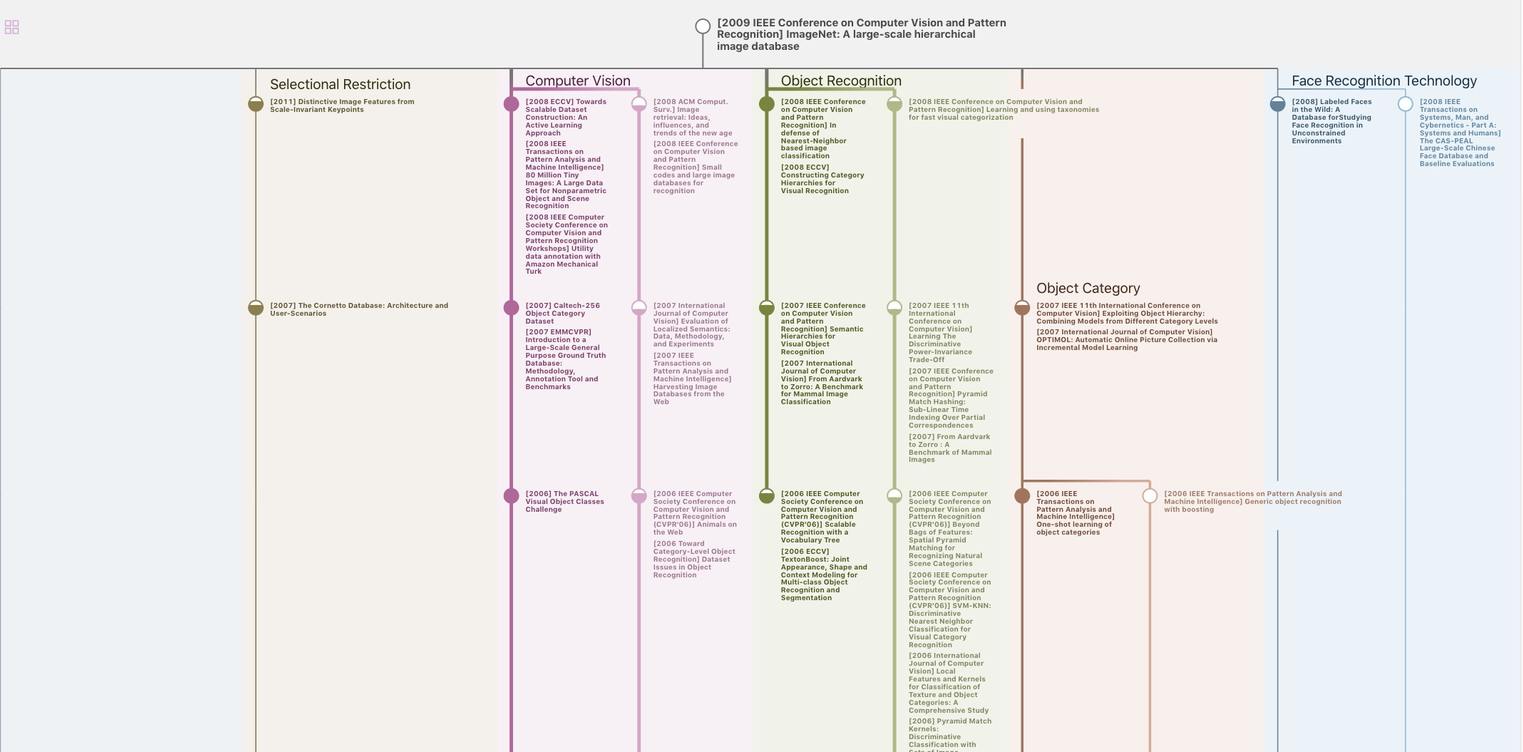
生成溯源树,研究论文发展脉络
Chat Paper
正在生成论文摘要