Autonomous learning of task sequences in robotic scenarios with non-stationary interdependencies
2023 21ST INTERNATIONAL CONFERENCE ON ADVANCED ROBOTICS, ICAR(2023)
摘要
Autonomously acquiring knowledge and skills interacting with the environment is fundamental for systems operating in real-world scenarios. While the majority of robotics applications consider a limited range of autonomy, research in autonomous open-ended learning is developing systems capable of adapting to contexts that cannot be foreseen at designtime, selecting their own goals and acquiring skills without the need for task-specific motivations or learning signals. In this perspective, the autonomous learning of interdependent tasks is still an open issue, especially in scenarios where dependencies are non-stationary and the system is forced to re-modulate previously acquired knowledge. Here we investigate these issues, presenting a hierarchical architecture allowing both the autonomous learning of multiple goals, and the sequences reflecting the relations between them. Leveraging the capability of the robot to self-monitor its competence, we endowed the system with a mechanism allowing the autonomous balancing of exploration/exploitation strategies to cope with the nonstationarity of the environment. We implemented the proposed architecture in a UR5e robot and tested it in a scenario with different manipulation tasks with changing interdependencies. Results show the effectiveness of our solution, paving the way to its application to more realistic scenarios involving compound objects and unstructured conditions changing over time.
更多查看译文
关键词
Sequence Learning,Autonomous Learning,Robot Scenario,Learning Task,Real-world Scenarios,Hierarchical Architecture,Majority Of Applications,Task Interdependence,Time Step,Learning Process,Preconditioning,Specific Tasks,Intrinsic Motivation,Multiple Tasks,Markov Decision Process,Changes In Tasks,Real Robot,Smoothing Factor,Robot Performance,End Of Epoch,Goal Selection,Bandit Problem
AI 理解论文
溯源树
样例
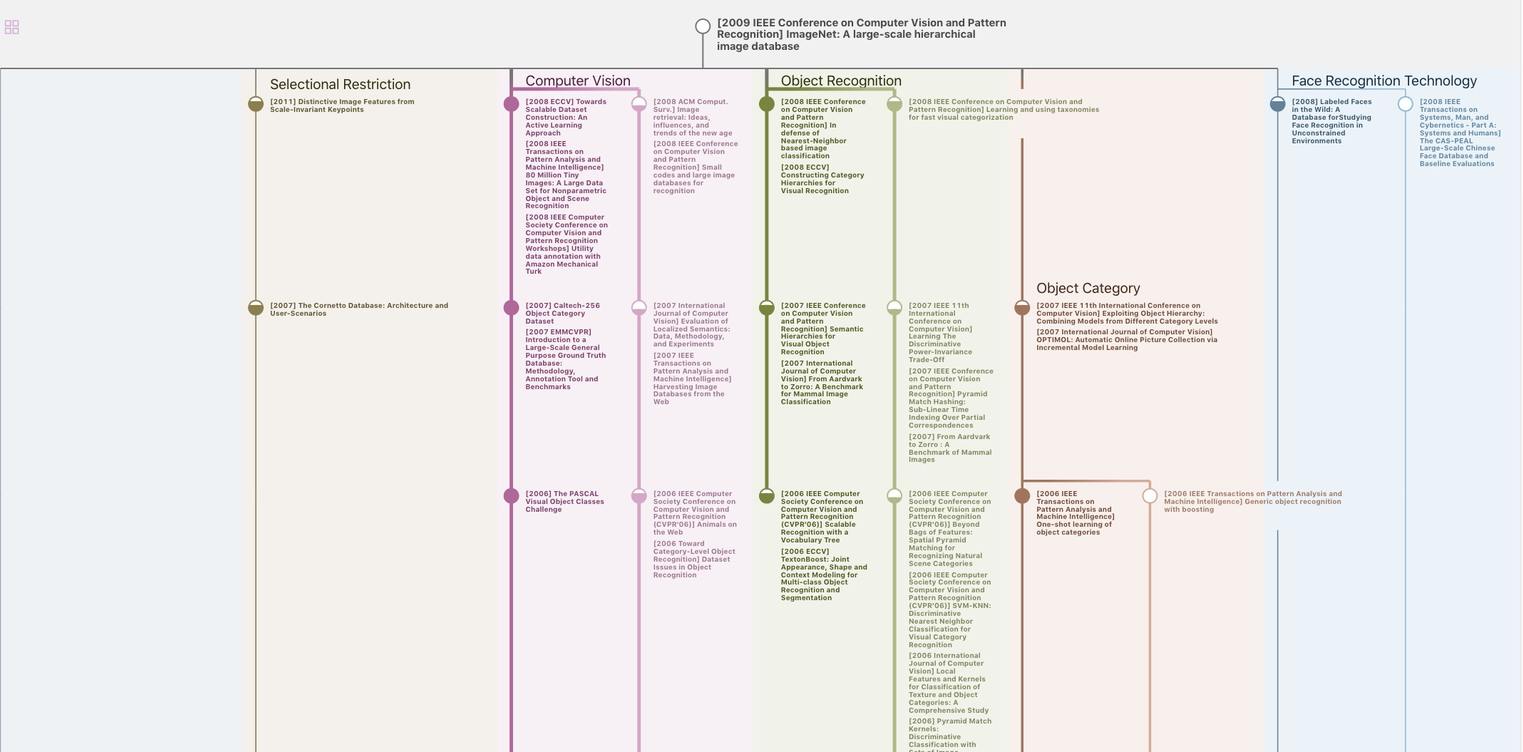
生成溯源树,研究论文发展脉络
Chat Paper
正在生成论文摘要