Transformer with Multi-block Encoder for Multi-turn Dialogue Translation.
2023 IEEE International Conference on Industrial Engineering and Engineering Management (IEEM)(2023)
摘要
Dialogue translation, typically reliant on sentence-level translation models, often struggles with accurately capturing contextual relationships and cross-sentence semantics. To address this, we took inspiration from document-level translation models and propose a Transformer architecture with a multi-block encoder, equipped with our novel context aggregation method. The applicability and effectiveness of these proposals were tested across three chat translation datasets using automated evaluation metrics. Notably, the integration of the context aggregation method improved the baseline model performance, while the Transformer with Multi-block Encoder demonstrated substantial gains in particular datasets (BLEU, METEOR). Moreover, our model and method displayed versatility, adapting effectively to various chat scenarios. These findings affirm the potential of the Transformer with Multi-block Encoder and the context aggregation method in enhancing dialogue translation by ensuring greater context sensitivity and adaptability.
更多查看译文
关键词
Dialogue Translation,Deep Learning
AI 理解论文
溯源树
样例
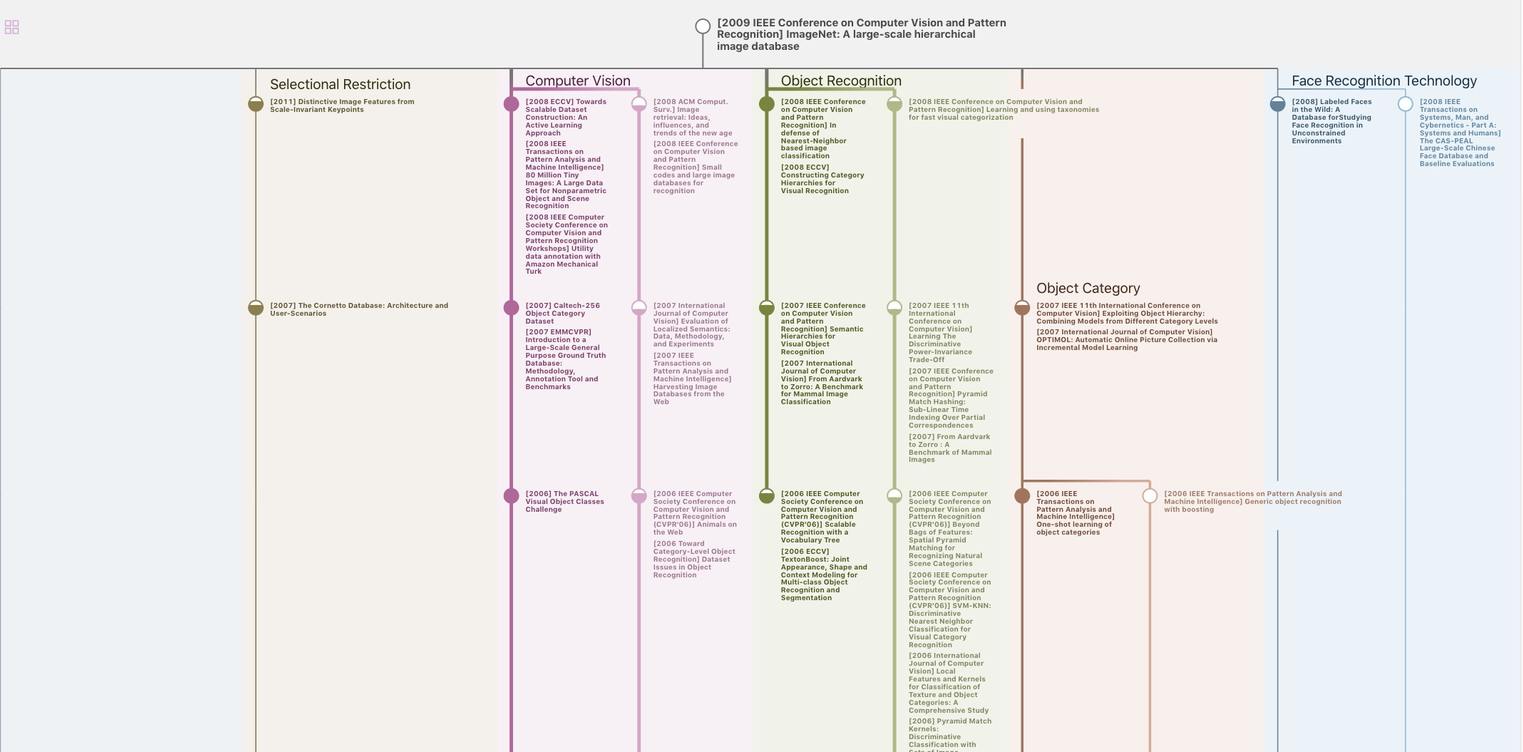
生成溯源树,研究论文发展脉络
Chat Paper
正在生成论文摘要