Information Extraction from Lengthy Legal Contracts: Leveraging Query-Based Summarization and GPT-3.5
LEGAL KNOWLEDGE AND INFORMATION SYSTEMS(2023)
摘要
In the legal domain, extracting information from contracts poses significant challenges, primarily due to the scarcity of annotated data. In such situations, leveraging large language models (LLMs), such as the Generative Pretrained Transformer (GPT) models, offers a promising solution. However, the inherent token limitations of these models can be a bottleneck for processing lengthy legal contracts. This paper presents an unsupervised two-step approach to address these challenges. First, we propose a query-based summarization model that extracts sentences pertinent to predefined queries, concisely representing lengthy contracts. This summarization ensures that the core information remains intact while simultaneously addressing the token limitation issue. Subsequently, the generated summary is fed to GPT-3.5 for precise information extraction. Our approach effectively overcomes the challenges of token limitations and zero resources, enabling efficient and scalable information extraction from legal contracts. We compare our results with those obtained from supervised models that have been fine-tuned on domain-specific annotated data. Experimental results demonstrate the remarkable effectiveness of our approach, as it achieves state-of-the-art performance without the need for domain-specific training data.
更多查看译文
关键词
Information extraction,text summarization,lengthy legal contracts,zero-resource,large language models,unsupervised approach
AI 理解论文
溯源树
样例
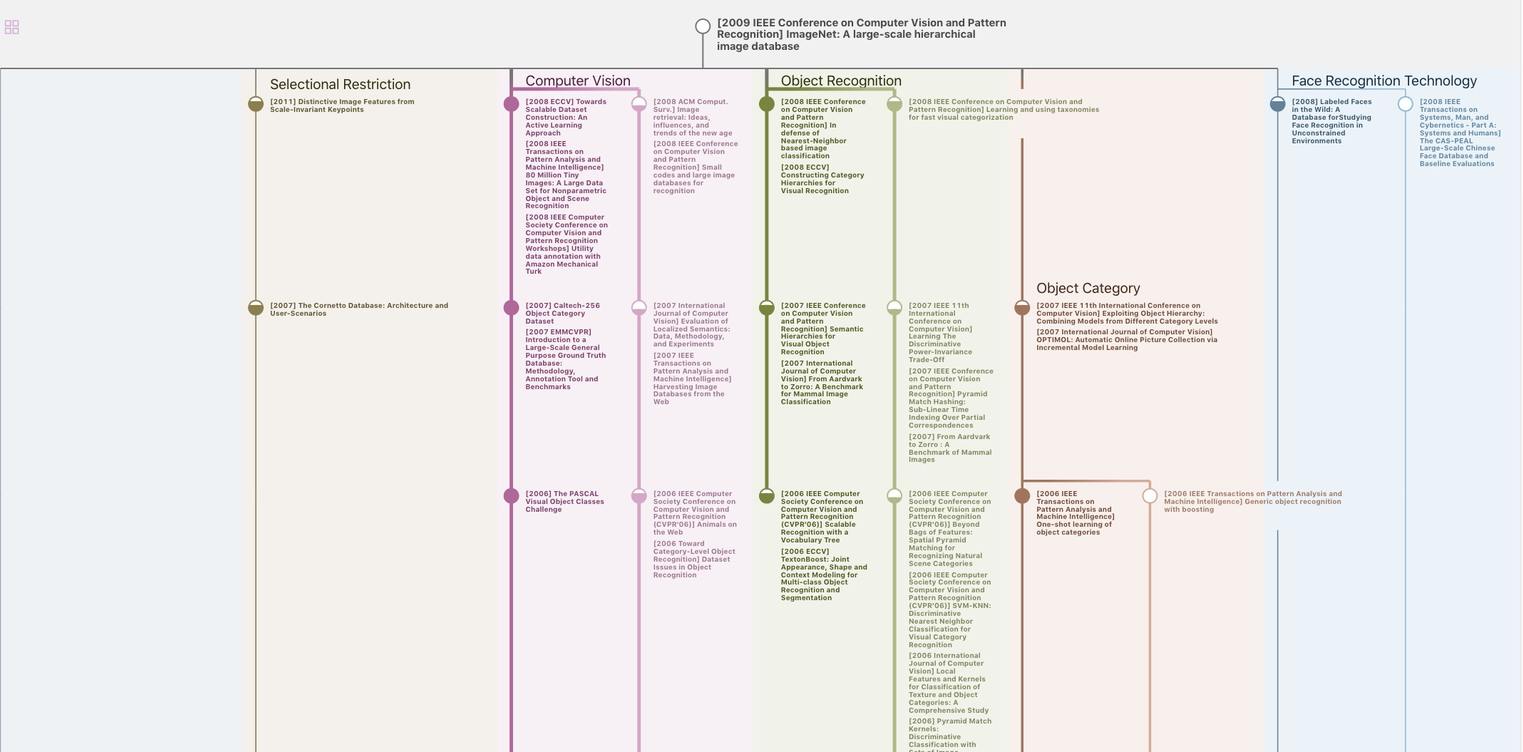
生成溯源树,研究论文发展脉络
Chat Paper
正在生成论文摘要