CGS-Mask: Making Time Series Predictions Intuitive for All
AAAI 2024(2024)
摘要
Artificial intelligence (AI) has immense potential in time series prediction, but most explainable tools have limited capabilities in providing a systematic understanding of important features over time. These tools typically rely on evaluating a single time point, overlook the time ordering of inputs, and neglect the time-sensitive nature of time series applications. These factors make it difficult for users, particularly those without domain knowledge, to comprehend AI model decisions and obtain meaningful explanations. We propose CGS-Mask, a post-hoc and model-agnostic cellular genetic strip mask-based saliency approach to address these challenges. CGS-Mask uses consecutive time steps as a cohesive entity to evaluate the impact of features on the final prediction, providing binary and sustained feature importance scores over time. Our algorithm optimizes the mask population iteratively to obtain the optimal mask in a reasonable time. We evaluated CGS-Mask on synthetic and real-world datasets, and it outperformed state-of-the-art methods in elucidating the importance of features over time. According to our pilot user study via a questionnaire survey, CGS-Mask is the most effective approach in presenting easily understandable time series prediction results, enabling users to comprehend the decision-making process of AI models with ease.
更多查看译文
关键词
ML: Transparent, Interpretable, Explainable ML,ML: Time-Series/Data Streams
AI 理解论文
溯源树
样例
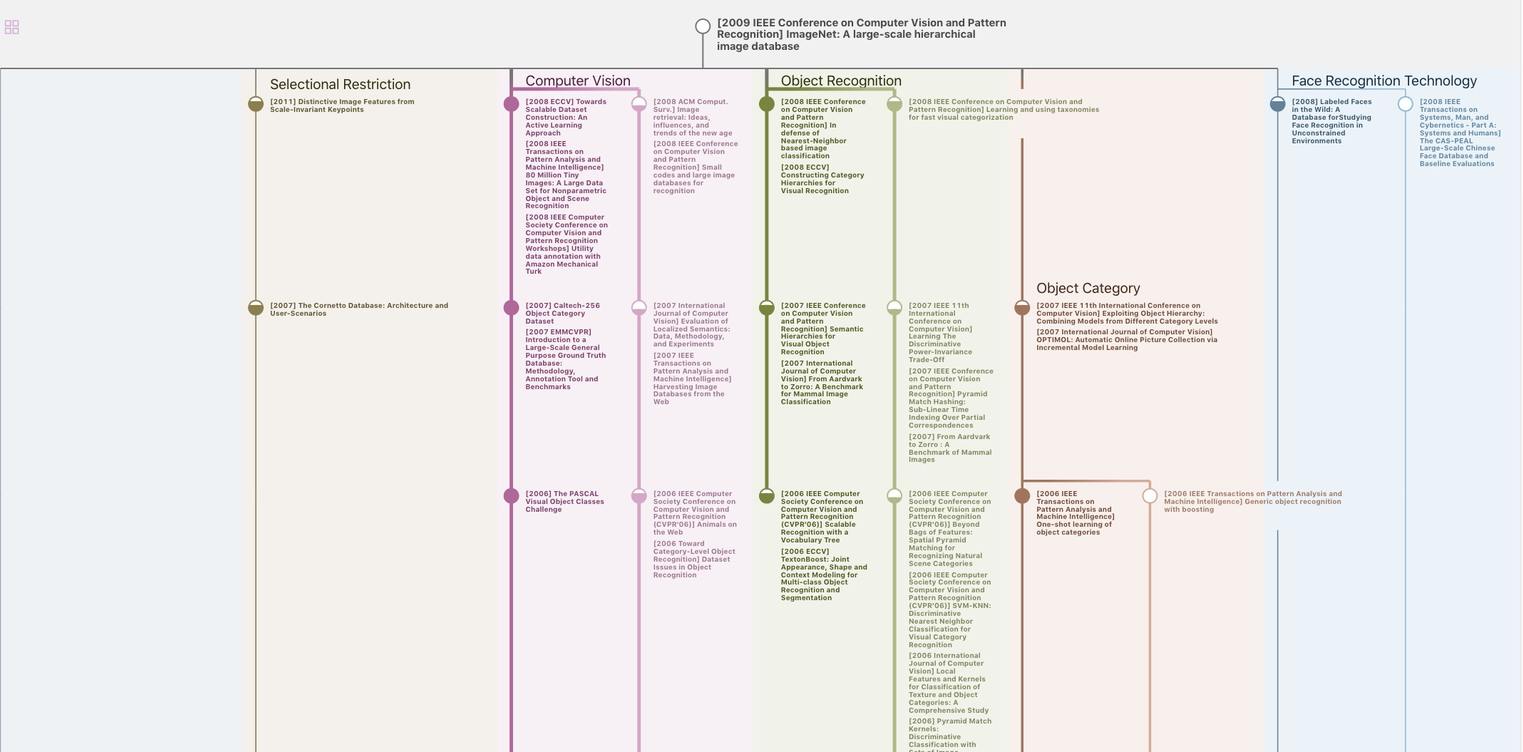
生成溯源树,研究论文发展脉络
Chat Paper
正在生成论文摘要